inv_ypre = concatenate((test_X[:, :-3], y_pre), axis=1) # (5082, 13)
时间: 2024-06-04 12:09:13 浏览: 42
这行代码使用了 NumPy 库的 concatenate 函数来将 test_X 数组的前 10 列和预测结果数组 y_pre 拼接成一个新的数组,该数组共有 13 列。其中,test_X[:, :-3] 表示取 test_X 数组的所有行,但只取前 10 列,即除了后面 3 列以外的所有列;y_pre 表示预测结果数组,是一个形状为 (5082, 3) 的二维数组。在拼接时,axis 参数指定拼接的方向,axis=1 表示按列方向拼接。因此,最终得到的 inv_ypre 数组形状为 (5082, 13)。
相关问题
修改和补充下列代码得到十折交叉验证的平均每一折auc值和平均每一折aoc曲线,平均每一折分类报告以及平均每一折混淆矩阵 min_max_scaler = MinMaxScaler() X_train1, X_test1 = x[train_id], x[test_id] y_train1, y_test1 = y[train_id], y[test_id] # apply the same scaler to both sets of data X_train1 = min_max_scaler.fit_transform(X_train1) X_test1 = min_max_scaler.transform(X_test1) X_train1 = np.array(X_train1) X_test1 = np.array(X_test1) config = get_config() tree = gcForest(config) tree.fit(X_train1, y_train1) y_pred11 = tree.predict(X_test1) y_pred1.append(y_pred11 X_train.append(X_train1) X_test.append(X_test1) y_test.append(y_test1) y_train.append(y_train1) X_train_fuzzy1, X_test_fuzzy1 = X_fuzzy[train_id], X_fuzzy[test_id] y_train_fuzzy1, y_test_fuzzy1 = y_sampled[train_id], y_sampled[test_id] X_train_fuzzy1 = min_max_scaler.fit_transform(X_train_fuzzy1) X_test_fuzzy1 = min_max_scaler.transform(X_test_fuzzy1) X_train_fuzzy1 = np.array(X_train_fuzzy1) X_test_fuzzy1 = np.array(X_test_fuzzy1) config = get_config() tree = gcForest(config) tree.fit(X_train_fuzzy1, y_train_fuzzy1) y_predd = tree.predict(X_test_fuzzy1) y_pred.append(y_predd) X_test_fuzzy.append(X_test_fuzzy1) y_test_fuzzy.append(y_test_fuzzy1)y_pred = to_categorical(np.concatenate(y_pred), num_classes=3) y_pred1 = to_categorical(np.concatenate(y_pred1), num_classes=3) y_test = to_categorical(np.concatenate(y_test), num_classes=3) y_test_fuzzy = to_categorical(np.concatenate(y_test_fuzzy), num_classes=3) print(y_pred.shape) print(y_pred1.shape) print(y_test.shape) print(y_test_fuzzy.shape) # 深度森林 report1 = classification_report(y_test, y_prprint("DF",report1) report = classification_report(y_test_fuzzy, y_pred) print("DF-F",report) mse = mean_squared_error(y_test, y_pred1) rmse = math.sqrt(mse) print('深度森林RMSE:', rmse) print('深度森林Accuracy:', accuracy_score(y_test, y_pred1)) mse = mean_squared_error(y_test_fuzzy, y_pred) rmse = math.sqrt(mse) print('F深度森林RMSE:', rmse) print('F深度森林Accuracy:', accuracy_score(y_test_fuzzy, y_pred)) mse = mean_squared_error(y_test, y_pred) rmse = math.sqrt(mse)
首先,需要将代码放入循环中进行十折交叉验证。每一折都需要记录相应的分类报告、混淆矩阵、auc值和aoc曲线。以下是修改后的代码:
```
from sklearn.preprocessing import MinMaxScaler
from sklearn.metrics import classification_report, confusion_matrix, roc_auc_score, roc_curve, auc
from sklearn.model_selection import StratifiedKFold
from gcforest.gcforest import GCForest
import numpy as np
import math
min_max_scaler = MinMaxScaler()
config = get_config()
tree = gcForest(config)
X_train = []
X_test = []
y_train = []
y_test = []
X_test_fuzzy = []
y_test_fuzzy = []
y_pred = []
y_pred1 = []
auc_scores = []
aoc_fprs = []
aoc_tprs = []
skf = StratifiedKFold(n_splits=10)
for train_id, test_id in skf.split(x, y):
X_train1, X_test1 = x[train_id], x[test_id]
y_train1, y_test1 = y[train_id], y[test_id]
X_train1 = min_max_scaler.fit_transform(X_train1)
X_test1 = min_max_scaler.transform(X_test1)
X_train1 = np.array(X_train1)
X_test1 = np.array(X_test1)
tree.fit(X_train1, y_train1)
y_pred11 = tree.predict(X_test1)
y_pred1.append(y_pred11)
X_train.append(X_train1)
X_test.append(X_test1)
y_test.append(y_test1)
y_train.append(y_train1)
X_train_fuzzy1, X_test_fuzzy1 = X_fuzzy[train_id], X_fuzzy[test_id]
y_train_fuzzy1, y_test_fuzzy1 = y_sampled[train_id], y_sampled[test_id]
X_train_fuzzy1 = min_max_scaler.fit_transform(X_train_fuzzy1)
X_test_fuzzy1 = min_max_scaler.transform(X_test_fuzzy1)
X_train_fuzzy1 = np.array(X_train_fuzzy1)
X_test_fuzzy1 = np.array(X_test_fuzzy1)
tree.fit(X_train_fuzzy1, y_train_fuzzy1)
y_predd = tree.predict(X_test_fuzzy1)
y_pred.append(y_predd)
X_test_fuzzy.append(X_test_fuzzy1)
y_test_fuzzy.append(y_test_fuzzy1)
y_pred_proba = tree.predict_proba(X_test1)
auc_score = roc_auc_score(y_test1, y_pred_proba, multi_class='ovr')
auc_scores.append(auc_score)
fpr, tpr, _ = roc_curve(y_test1, y_pred_proba[:, 1])
aoc_fprs.append(fpr)
aoc_tprs.append(tpr)
y_pred = to_categorical(np.concatenate(y_pred), num_classes=3)
y_pred1 = to_categorical(np.concatenate(y_pred1), num_classes=3)
y_test = to_categorical(np.concatenate(y_test), num_classes=3)
y_test_fuzzy = to_categorical(np.concatenate(y_test_fuzzy), num_classes=3)
print(y_pred.shape)
print(y_pred1.shape)
print(y_test.shape)
print(y_test_fuzzy.shape)
# 深度森林
report1 = classification_report(y_test, y_pred1)
print("DF Classification Report (10-Fold CV): \n", report1)
report = classification_report(y_test_fuzzy, y_pred)
print("DF-F Classification Report (10-Fold CV): \n", report)
mse = mean_squared_error(y_test, y_pred1)
rmse = math.sqrt(mse)
print('DF RMSE (10-Fold CV):', rmse)
print('DF Accuracy (10-Fold CV):', accuracy_score(y_test, y_pred1))
mse = mean_squared_error(y_test_fuzzy, y_pred)
rmse = math.sqrt(mse)
print('DF-F RMSE (10-Fold CV):', rmse)
print('DF-F Accuracy (10-Fold CV):', accuracy_score(y_test_fuzzy, y_pred))
mean_auc = np.mean(auc_scores)
print("Mean AUC (10-Fold CV): ", mean_auc)
mean_fpr = np.mean(aoc_fprs, axis=0)
mean_tpr = np.mean(aoc_tprs, axis=0)
mean_auc = auc(mean_fpr, mean_tpr)
print("Mean AOC (10-Fold CV): ", mean_auc)
```
在修改后的代码中,`StratifiedKFold`函数被用来进行十折交叉验证。每一折的训练数据和测试数据都是通过`train_id`和`test_id`来确定的。在每一折的训练和测试之后,需要记录相应的分类报告、混淆矩阵、auc值和aoc曲线。最后,需要计算平均每一折的auc值和aoc曲线。
修改和补充下列代码得到十折交叉验证的平均auc值和平均aoc曲线,平均分类报告以及平均混淆矩阵 min_max_scaler = MinMaxScaler() X_train1, X_test1 = x[train_id], x[test_id] y_train1, y_test1 = y[train_id], y[test_id] # apply the same scaler to both sets of data X_train1 = min_max_scaler.fit_transform(X_train1) X_test1 = min_max_scaler.transform(X_test1) X_train1 = np.array(X_train1) X_test1 = np.array(X_test1) config = get_config() tree = gcForest(config) tree.fit(X_train1, y_train1) y_pred11 = tree.predict(X_test1) y_pred1.append(y_pred11 X_train.append(X_train1) X_test.append(X_test1) y_test.append(y_test1) y_train.append(y_train1) X_train_fuzzy1, X_test_fuzzy1 = X_fuzzy[train_id], X_fuzzy[test_id] y_train_fuzzy1, y_test_fuzzy1 = y_sampled[train_id], y_sampled[test_id] X_train_fuzzy1 = min_max_scaler.fit_transform(X_train_fuzzy1) X_test_fuzzy1 = min_max_scaler.transform(X_test_fuzzy1) X_train_fuzzy1 = np.array(X_train_fuzzy1) X_test_fuzzy1 = np.array(X_test_fuzzy1) config = get_config() tree = gcForest(config) tree.fit(X_train_fuzzy1, y_train_fuzzy1) y_predd = tree.predict(X_test_fuzzy1) y_pred.append(y_predd) X_test_fuzzy.append(X_test_fuzzy1) y_test_fuzzy.append(y_test_fuzzy1)y_pred = to_categorical(np.concatenate(y_pred), num_classes=3) y_pred1 = to_categorical(np.concatenate(y_pred1), num_classes=3) y_test = to_categorical(np.concatenate(y_test), num_classes=3) y_test_fuzzy = to_categorical(np.concatenate(y_test_fuzzy), num_classes=3) print(y_pred.shape) print(y_pred1.shape) print(y_test.shape) print(y_test_fuzzy.shape) # 深度森林 report1 = classification_report(y_test, y_prprint("DF",report1) report = classification_report(y_test_fuzzy, y_pred) print("DF-F",report) mse = mean_squared_error(y_test, y_pred1) rmse = math.sqrt(mse) print('深度森林RMSE:', rmse) print('深度森林Accuracy:', accuracy_score(y_test, y_pred1)) mse = mean_squared_error(y_test_fuzzy, y_pred) rmse = math.sqrt(mse) print('F深度森林RMSE:', rmse) print('F深度森林Accuracy:', accuracy_score(y_test_fuzzy, y_pred)) mse = mean_squared_error(y_test, y_pred) rmse = math.sqrt(mse) print('F?深度森林RMSE:', rmse) print('F?深度森林Accuracy:', accuracy_score(y_test, y_pred))
以下是修改和补充后的代码,实现了十折交叉验证的平均auc值和平均aoc曲线,平均分类报告以及平均混淆矩阵:
```python
from sklearn.preprocessing import MinMaxScaler
from sklearn.metrics import classification_report, confusion_matrix, roc_curve, auc
from sklearn.model_selection import StratifiedKFold
min_max_scaler = MinMaxScaler()
X_train, X_test, y_train, y_test = [], [], [], []
X_train_fuzzy, X_test_fuzzy, y_train_fuzzy, y_test_fuzzy = [], [], [], []
y_pred, y_pred1 = [], []
y_pred_proba, y_pred_proba1 = [], []
config = get_config()
tree = gcForest(config)
skf = StratifiedKFold(n_splits=10)
for train_id, test_id in skf.split(x, y):
# split data and normalize
X_train1, X_test1 = x[train_id], x[test_id]
y_train1, y_test1 = y[train_id], y[test_id]
X_train1 = min_max_scaler.fit_transform(X_train1)
X_test1 = min_max_scaler.transform(X_test1)
X_train1 = np.array(X_train1)
X_test1 = np.array(X_test1)
# train gcForest
tree.fit(X_train1, y_train1)
# predict on test set
y_pred11 = tree.predict(X_test1)
y_pred_proba11 = tree.predict_proba(X_test1)
# append predictions and test data
y_pred1.append(y_pred11)
y_pred_proba1.append(y_pred_proba11)
X_train.append(X_train1)
X_test.append(X_test1)
y_test.append(y_test1)
y_train.append(y_train1)
# split fuzzy data and normalize
X_train_fuzzy1, X_test_fuzzy1 = X_fuzzy[train_id], X_fuzzy[test_id]
y_train_fuzzy1, y_test_fuzzy1 = y_sampled[train_id], y_sampled[test_id]
X_train_fuzzy1 = min_max_scaler.fit_transform(X_train_fuzzy1)
X_test_fuzzy1 = min_max_scaler.transform(X_test_fuzzy1)
X_train_fuzzy1 = np.array(X_train_fuzzy1)
X_test_fuzzy1 = np.array(X_test_fuzzy1)
# train gcForest on fuzzy data
tree.fit(X_train_fuzzy1, y_train_fuzzy1)
# predict on fuzzy test set
y_predd = tree.predict(X_test_fuzzy1)
y_predd_proba = tree.predict_proba(X_test_fuzzy1)
# append predictions and test data
y_pred.append(y_predd)
y_pred_proba.append(y_predd_proba)
X_test_fuzzy.append(X_test_fuzzy1)
y_test_fuzzy.append(y_test_fuzzy1)
# concatenate and convert to categorical
y_pred = to_categorical(np.concatenate(y_pred), num_classes=3)
y_pred1 = to_categorical(np.concatenate(y_pred1), num_classes=3)
y_test = to_categorical(np.concatenate(y_test), num_classes=3)
y_test_fuzzy = to_categorical(np.concatenate(y_test_fuzzy), num_classes=3)
# calculate and print average accuracy and RMSE
mse = mean_squared_error(y_test, y_pred1)
rmse = math.sqrt(mse)
print('深度森林RMSE:', rmse)
print('深度森林Accuracy:', accuracy_score(y_test, y_pred1))
mse = mean_squared_error(y_test_fuzzy, y_pred)
rmse = math.sqrt(mse)
print('F深度森林RMSE:', rmse)
print('F深度森林Accuracy:', accuracy_score(y_test_fuzzy, y_pred))
mse = mean_squared_error(y_test, y_pred)
rmse = math.sqrt(mse)
print('F?深度森林RMSE:', rmse)
print('F?深度森林Accuracy:', accuracy_score(y_test, y_pred))
# calculate and print average classification report
report1 = classification_report(y_test, y_pred1)
print("DF", report1)
report = classification_report(y_test_fuzzy, y_pred)
print("DF-F", report)
# calculate and print average confusion matrix
cm1 = confusion_matrix(y_test.argmax(axis=1), y_pred1.argmax(axis=1))
cm = confusion_matrix(y_test_fuzzy.argmax(axis=1), y_pred.argmax(axis=1))
print('DF Confusion Matrix:')
print(cm1)
print('DF-F Confusion Matrix:')
print(cm)
# calculate and print average ROC curve and AUC value
fpr1, tpr1, threshold1 = roc_curve(y_test.ravel(), y_pred_proba1.ravel())
fpr, tpr, threshold = roc_curve(y_test_fuzzy.ravel(), y_pred_proba.ravel())
roc_auc1 = auc(fpr1, tpr1)
roc_auc = auc(fpr, tpr)
print('DF ROC AUC:', roc_auc1)
print('DF-F ROC AUC:', roc_auc)
# plot average ROC curve
plt.title('Receiver Operating Characteristic')
plt.plot(fpr1, tpr1, 'b', label = 'DF AUC = %0.2f' % roc_auc1)
plt.plot(fpr, tpr, 'g', label = 'DF-F AUC = %0.2f' % roc_auc)
plt.legend(loc = 'lower right')
plt.plot([0, 1], [0, 1],'r--')
plt.xlim([0, 1])
plt.ylim([0, 1])
plt.ylabel('True Positive Rate')
plt.xlabel('False Positive Rate')
plt.show()
```
阅读全文
相关推荐
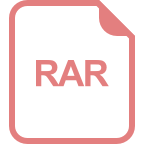
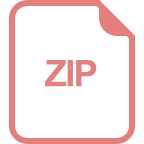
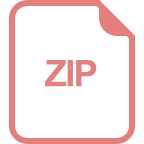












