if mode == 'TRAIN': self.preprocess_rpn_training_data() else: self.sample_id_list = [int(sample_id) for sample_id in self.image_idx_list] self.logger.info('Load testing samples from %s' % self.imageset_dir) self.logger.info('Done: total test samples %d' % len(self.sample_id_list))该语句中的sample_id_list最后等于什么?
时间: 2024-02-14 20:35:55 浏览: 140
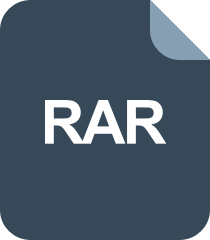
OpenCV_TEST.rar_openCVTest_opencv 中心线_opencv 道路识别_site:www.pudn.

在这个代码片段中,如果mode等于'TRAIN',则会调用preprocess_rpn_training_data()函数进行训练数据的预处理。如果mode不等于'TRAIN',则会将self.image_idx_list中的元素转换为整数类型并存储在sample_id_list中。因此,sample_id_list最后会等于self.image_idx_list中的元素转换为整数类型后的列表。
阅读全文
相关推荐
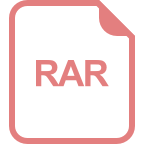
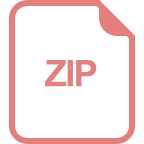
















