1.r语言自带的数据集iris列出了3个物种(species)的萼片长(sepal.length)、萼片宽(
时间: 2023-09-27 18:02:56 浏览: 62
sepal.width)、花瓣长(petal.length)和花瓣宽(petal.width)四个特征的测量数据。这些数据集包含了150个样本,每个样本都属于三个物种之一:setosa、versicolor和virginica。
萼片长和萼片宽是关于花萼(萼片)的两个测量维度。它们可以帮助我们了解花萼的大小和形状。
花瓣长和花瓣宽是关于花瓣的两个测量维度。花瓣是花朵上色彩鲜艳的部分,测量花瓣的大小和形状可以帮助我们区分不同的物种。
通过分析iris数据集中不同物种的特征测量值,我们可以得出一些有用的结论。例如,我们可以比较不同物种的萼片和花瓣的大小差异,从而判断它们之间的区别。此外,我们还可以使用统计分析和数据可视化工具来探索数据之间的相关性和模式。
总之,iris数据集是一个用于研究和分析不同物种之间特征差异的常用数据集。它通过提供简单且易于理解的特征测量数据,帮助研究者深入了解植物分类学中的群体差异,并为机器学习和数据科学领域的模型训练提供了一个常用的示例数据集。
相关问题
r自带的数据集iris 列出了3 个物种(species)的萼片长(sepal.length)萼片宽(sepal.
iris数据集是一种经典的数据集,其中包含了150朵鸢尾花的相关数据。这些数据包括鸢尾花的萼片长度(sepal length)、萼片宽度(sepal width)、花瓣长度(petal length)、花瓣宽度(petal width)以及所属的物种(species)。其中物种分为setosa、versicolor和virginica三类。
在iris数据集中,我们可以列出三个物种的萼片长度和萼片宽度的数据如下:
1. setosa鸢尾花的萼片长度与萼片宽度数据分别为:5.1, 3.5;4.9, 3.0;4.7, 3.2;4.6, 3.1;5.0, 3.6;5.4, 3.9;4.6, 3.4;5.0, 3.4;4.4, 2.9;4.9, 3.1等。
2. versicolor鸢尾花的萼片长度与萼片宽度数据分别为:7.0, 3.2;6.4, 3.2;6.9, 3.1;5.5, 2.3;6.5, 2.8;5.7, 2.8;6.3, 3.3;4.9, 2.4;6.6, 2.9;5.2, 2.7等。
3. virginica鸢尾花的萼片长度与萼片宽度数据分别为:6.3, 3.3;5.8, 2.7;7.1, 3.0;6.3, 2.9;6.5, 3.0;7.6, 3.0;4.9, 2.5;7.3, 2.9;6.7, 2.5;7.2, 3.6等。
通过iris数据集中的这些数据,我们可以对不同物种的鸢尾花的萼片长度和萼片宽度进行分析并比较,从而更好地了解这些物种之间的特点和差异。
换个代码绘制代码R自带的数据集iris 列出了3个物种(specie) 的萼片长(sepal.lngth)、曹片宽(spal. width)、花瓣长(ptal.length).花瓣宽(petalwidth) 4个变量的各50个样本数据聚类图
好的,你可以使用下面的代码来绘制iris数据集的聚类图:
```R
# 加载必要的包
library(ggplot2)
library(dplyr)
library(cluster)
# 加载iris数据集
data(iris)
# 选择特定的变量和物种
iris_subset <- iris %>%
filter(Species %in% c("setosa", "versicolor", "virginica")) %>%
select(Species, Sepal.Length, Sepal.Width, Petal.Length, Petal.Width)
# 对数据进行聚类
iris_cluster <- iris_subset %>%
select(-Species) %>%
scale() %>%
dist() %>%
hclust()
# 绘制聚类图
ggplot(iris_subset, aes(x = Species, y = Petal.Length, color = as.factor(iris_cluster$clusters))) +
geom_point(size = 3) +
scale_color_manual(values = c("red", "blue", "green")) +
labs(title = "Iris Clustering", x = "Species", y = "Petal Length") +
theme_minimal()
```
这个代码首先加载了必要的包,然后从iris数据集中选择了特定的变量和物种。接下来,对数据进行了聚类,然后绘制了聚类图。聚类图上的颜色表示聚类结果,可以使用 `scale_color_manual` 函数来自定义颜色。最后,添加标题、轴标签和主题等,使图表更加美观易懂。
相关推荐
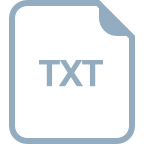
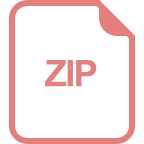













