如何将logits 和 aux_logits的结果整合为一个2分类的张量结果
时间: 2024-10-07 16:03:12 浏览: 36
将`logits`和`aux_logits`整合成一个二分类(Binary Classification)张量结果,通常是在二分类任务中,你需要从一个多分类模型中选择一个特定类别作为正类,其余为负类。对于`logits`,这通常是通过softmax函数处理,得到每个类别的概率,然后找到概率最高的那个类作为正类。
例如,在`logits`上进行操作:
```python
softmax_logits = F.softmax(logits, dim=1)
binary_pred = torch.argmax(softmax_logits, dim=1).unsqueeze(1) # 取最大值所在的索引,并添加维度以便匹配形状
```
对于`aux_logits`,如果模型提供的话,你也可以采取类似的方式处理。如果没有特别说明,有些时候可能会忽略它们,因为主分类 logits 更重要。
如果需要二分类,你可以设置一个阈值,比如大于某个值的作为正类,小于等于该值的作为负类。比如:
```python
threshold = 0.5
binary_pred = (softmax_logits[:, 1] > threshold).float() # 1代表正类,0代表负类
```
需要注意的是,这里的假设是最后一维代表类别的数量,如果是二分类,则通常最后一维为2。具体操作可能会因模型结构和需求而变化。
相关问题
将下面代码改为用checkpoint保存saver=tf.train.Saver() # 训练或预测 train = False # 模型文件路径 model_path = "model" if train: print("训练模式") # 训练初始化参数 # 定义输入和Label以填充容器 训练时dropout为0.25 train_feed_dict = { xs: x_train, ys: y_train, drop: 0.25 } # 训练学习1000次 for step in range(1000): with tf.GradientTape() as tape: logits_val = logits(train_feed_dict) loss_val = tf.reduce_mean(tf.nn.softmax_cross_entropy_with_logits(labels=tf.one_hot(y_train, num_classes), logits=logits_val)) grads = tape.gradient(loss_val, logits.trainable_variables) optimizer.apply_gradients(zip(grads, logits.trainable_variables)) if step % 50 == 0: #每隔50次输出一次结果 print("step = {}\t mean loss = {}".format(step, loss_val)) # 保存模型 saver.save(logits, model_path) print("训练结束,保存模型到{}".format(model_path)) else: print("测试模式") # 测试载入参数 logits=tf.keras.models.load_model(model_path) print("从{}载入模型".format(model_path))
# 首先需要在计算图中定义一个变量来保存模型的全局步数
global_step = tf.Variable(0, dtype=tf.int32, trainable=False, name='global_step')
# 在训练过程中需要增加global_step
train_op = optimizer.apply_gradients(zip(grads, logits.trainable_variables), global_step=global_step)
# 在定义saver时,指定要保存的变量和保存路径,注意不要在文件名中包含global_step变量
saver = tf.train.Saver(var_list=logits.trainable_variables, max_to_keep=3) # 最多保存3个模型
with tf.Session() as sess:
if train:
print("训练模式")
# 恢复之前训练好的模型
latest_checkpoint = tf.train.latest_checkpoint(model_path)
if latest_checkpoint:
print("从{}载入模型".format(latest_checkpoint))
saver.restore(sess, latest_checkpoint)
else:
sess.run(tf.global_variables_initializer())
# 训练学习1000次
for step in range(1000):
_, loss_val, global_step_val = sess.run([train_op, loss, global_step], feed_dict=train_feed_dict)
if step % 50 == 0:
print("step = {}\t mean loss = {}".format(global_step_val, loss_val))
# 每隔100步保存一次模型
if global_step_val % 100 == 0:
saver.save(sess, model_path + "/model", global_step=global_step_val)
print("保存模型到{}-{}".format(model_path, global_step_val))
else:
print("测试模式")
# 载入最新的模型
latest_checkpoint = tf.train.latest_checkpoint(model_path)
if latest_checkpoint:
print("从{}载入模型".format(latest_checkpoint))
saver.restore(sess, latest_checkpoint)
else:
print("没有找到模型文件")
exit()
# 进行预测
我是一个初学者,请你帮我解释以下这段代码的每个变量的含义和每句代码的语法:def top_k_top_p_filtering(logits, top_k=0, top_p=0.0, filter_value=-float('Inf')): assert logits.dim() == 1 # batch size 1 for now - could be updated for more but the code would be less clear top_k = min(top_k, logits.size(-1)) # Safety check if top_k > 0: # Remove all tokens with a probability less than the last token of the top-k # torch.topk()返回最后一维最大的top_k个元素,返回值为二维(values,indices) # ...表示其他维度由计算机自行推断 indices_to_remove = logits < torch.topk(logits, top_k)[0][..., -1, None] logits[indices_to_remove] = filter_value if top_p > 0.0: sorted_logits, sorted_indices = torch.sort(logits, descending=True) cumulative_probs = torch.cumsum(F.softmax(sorted_logits, dim=-1), dim=-1) # Remove tokens with cumulative probability above the threshold sorted_indices_to_remove = cumulative_probs > top_p # Shift the indices to the right to keep also the first token above the threshold sorted_indices_to_remove[..., 1:] = sorted_indices_to_remove[..., :-1].clone() sorted_indices_to_remove[..., 0] = 0 indices_to_remove = sorted_indices[sorted_indices_to_remove] logits[indices_to_remove] = filter_value return logits
这段代码的作用是根据给定的top_k和top_p值,过滤掉logits分数较低的预测结果,从而生成更准确的预测结果。下面是每个变量的含义和每句代码的语法:
1. `logits`:一个张量,表示对应词汇表中单词的logits分数。
2. `top_k`:一个整数,表示要保留的最高可能性预测的数量。
3. `top_p`:一个浮点数,表示要保留的累积概率质量。
4. `filter_value`:一个浮点数,用于过滤掉不想要的预测。默认值为负无穷大。
5. `assert`:断言语句,用于判断logits张量的维度是否为1,如果维度不为1,程序将会报错并停止运行。
6. `logits.dim()`:张量的维度数。
7. `top_k = min(top_k, logits.size(-1))`:将top_k值与logits张量的最后一维大小进行比较,保证top_k值不会大于张量的维度。
8. `if top_k > 0:`:如果指定了top_k值,则进行以下操作。
9. `indices_to_remove = logits < torch.topk(logits, top_k)[0][..., -1, None]`:返回logits张量中最后一维的最大值的top_k个元素,并将剩余元素的值设置为过滤值, 然后返回不需要的结果的索引。
10. `logits[indices_to_remove] = filter_value`:将logits张量中的索引为indices_to_remove的元素的值设置为过滤值。
11. `if top_p > 0.0:`:如果指定了top_p值,则进行以下操作。
12. `sorted_logits, sorted_indices = torch.sort(logits, descending=True)`:按照降序对logits张量进行排序,并返回排序后的结果和对应的索引。
13. `cumulative_probs = torch.cumsum(F.softmax(sorted_logits, dim=-1), dim=-1)`:计算softmax函数的累积概率值。
14. `sorted_indices_to_remove = cumulative_probs > top_p`:返回累积概率大于top_p的索引。
15. `sorted_indices_to_remove[..., 1:] = sorted_indices_to_remove[..., :-1].clone()`:将索引向右移一位,保留第一个索引。
16. `sorted_indices_to_remove[..., 0] = 0`:将第一个索引设置为0。
17. `indices_to_remove = sorted_indices[sorted_indices_to_remove]`:返回不需要的结果的索引。
18. `logits[indices_to_remove] = filter_value`:将logits张量中的索引为indices_to_remove的元素的值设置为过滤值。
19. `return logits`:返回过滤后的logits张量。
阅读全文
相关推荐
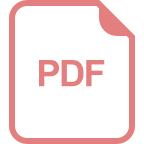
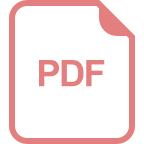














