使用pytorch训练一个基于多尺度自编码网络,实现红外与可见光图像融合的模型
时间: 2023-08-21 09:06:22 浏览: 131
首先,需要准备好可见光和红外图像的数据集,并将它们转换成张量形式。接着,我们可以定义一个多尺度自编码网络的模型,该模型包含了编码器和解码器两部分。编码器将输入图像压缩成一个较小的向量,解码器则将该向量还原成与原图像尺寸相同的输出图像。同时,为了实现多尺度融合,我们需要定义多个不同分辨率的自编码器,并将它们连接在一起。
以下是一个基于PyTorch的多尺度自编码网络的代码实现:
```python
import torch
import torch.nn as nn
import torch.nn.functional as F
class Encoder(nn.Module):
def __init__(self, in_channels, out_channels):
super(Encoder, self).__init__()
self.conv1 = nn.Conv2d(in_channels, out_channels, kernel_size=3, stride=1, padding=1)
self.conv2 = nn.Conv2d(out_channels, out_channels, kernel_size=3, stride=1, padding=1)
self.pool = nn.MaxPool2d(kernel_size=2, stride=2, return_indices=True)
def forward(self, x):
x = F.relu(self.conv1(x))
x = F.relu(self.conv2(x))
x, indices = self.pool(x)
return x, indices
class Decoder(nn.Module):
def __init__(self, in_channels, out_channels):
super(Decoder, self).__init__()
self.unpool = nn.MaxUnpool2d(kernel_size=2, stride=2)
self.conv1 = nn.Conv2d(in_channels, out_channels, kernel_size=3, stride=1, padding=1)
self.conv2 = nn.Conv2d(out_channels, out_channels, kernel_size=3, stride=1, padding=1)
def forward(self, x, indices):
x = self.unpool(x, indices)
x = F.relu(self.conv1(x))
x = F.relu(self.conv2(x))
return x
class MultiScaleAutoEncoder(nn.Module):
def __init__(self, in_channels, out_channels):
super(MultiScaleAutoEncoder, self).__init__()
self.encoder1 = Encoder(in_channels, out_channels)
self.encoder2 = Encoder(out_channels, out_channels*2)
self.encoder3 = Encoder(out_channels*2, out_channels*4)
self.decoder3 = Decoder(out_channels*4, out_channels*2)
self.decoder2 = Decoder(out_channels*2, out_channels)
self.decoder1 = Decoder(out_channels, in_channels)
def forward(self, x):
x1, indices1 = self.encoder1(x)
x2, indices2 = self.encoder2(x1)
x3, indices3 = self.encoder3(x2)
y3 = self.decoder3(x3, indices3)
y2 = self.decoder2(torch.cat([y3, x2], dim=1), indices2)
y1 = self.decoder1(torch.cat([y2, x1], dim=1), indices1)
return y1
```
在训练模型之前,我们需要定义损失函数和优化器。常用的损失函数包括均方误差和结构相似性损失,而常用的优化器包括Adam和SGD等。下面是一个训练函数的代码实现:
```python
def train(model, train_loader, criterion, optimizer):
model.train()
train_loss = 0
for batch_idx, (data, target) in enumerate(train_loader):
optimizer.zero_grad()
output = model(data)
loss = criterion(output, target)
loss.backward()
optimizer.step()
train_loss += loss.item() * data.size(0)
return train_loss / len(train_loader.dataset)
```
最后,我们可以使用上述代码来训练我们的多尺度自编码网络模型,以实现红外与可见光图像融合的功能。
阅读全文
相关推荐
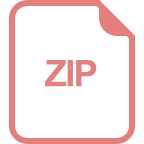
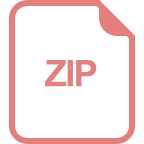
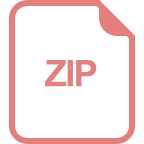









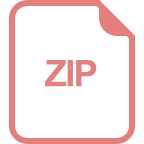
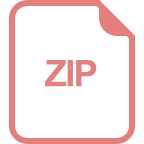
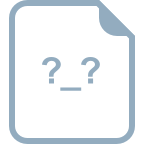