拟牛顿迭代法matlab程序
时间: 2023-12-15 14:05:24 浏览: 27
以下是拟牛顿迭代法的MATLAB程序:
```matlab
function [x, fval, exitflag, output] = fminunc(fun, x0, options, varargin)
%FMINUNC Multivariable unconstrained optimization using the quasi-Newton method of Broyden, Fletcher, Goldfarb, and Shanno.
% X = FMINUNC(FUN,X0) starts at X0 and attempts to find a local minimizer X of the function FUN. FUN is a function handle. FUN accepts input X and returns a scalar function value F evaluated at X. X0 can be a scalar, vector, or matrix.
%
% X = FMINUNC(FUN,X0,OPTIONS) minimizes with the default optimization parameters replaced by values in the structure OPTIONS, created with the OPTIMSET function. See OPTIMSET for details. Used options are Display, TolX, TolFun, DerivativeCheck, Diagnostics, FunValCheck, GradObj, Hessian, HessMult, HessPattern, InitialHessType, InitialHessMatrix, LargeScale, MaxFunEvals, MaxIter, OutputFcn, PlotFcns, ScaleProblem, TypicalX, and UseParallel.
%
% X = FMINUNC(PROBLEM) finds the minimum for PROBLEM. PROBLEM is a structure with the function FUN in PROBLEM.objective, the start point in PROBLEM.x0, the options structure in PROBLEM.options, and solver name 'fminunc' in PROBLEM.solver. The PROBLEM structure must have all the fields.
%
% [X,FVAL] = FMINUNC(FUN,X0,...) returns FVAL, the value of the objective function FUN at the solution X.
%
% [X,FVAL,EXITFLAG] = FMINUNC(FUN,X0,...) returns an EXITFLAG that describes the exit condition. Possible values of EXITFLAG and the corresponding exit conditions are
% 1 Maximum coordinate difference between current best point and other points in simplex is less than or equal to TolX and corresponding difference in function values is less than or equal to TolFun.
% 0 Maximum number of function evaluations or iterations reached.
% -1 Optimization terminated by the output function.
% -2 No feasible point was found.
% -3 Problem is unbounded.
% -4 Line search failed.
% -5 Trust region radius became too small.
% -6 Trust region radius became too large.
% -7 Objective function is undefined at initial point.
%
% [X,FVAL,EXITFLAG,OUTPUT] = FMINUNC(FUN,X0,...) returns a structure OUTPUT with the number of iterations taken in OUTPUT.iterations, the number of function evaluations in OUTPUT.funcCount, the algorithm used in OUTPUT.algorithm, the number of CG iterations (if used) in OUTPUT.cgiterations, the number of function evaluations (if used) in OUTPUT.firstorderopt, and the exit message in OUTPUT.message.
%
% Examples
% FUN can be specified using @:
% X = fminunc(@sin,3)
% finds a minimum of the SIN function near 3.
%
% FUN can be an anonymous function:
% X = fminunc(@(x) norm(x),[1;2;3])
% returns a point near the origin.
%
% FUN can be a parameterized function. Use an anonymous function to capture the problem-dependent parameters:
% f = @(x,c) x(1).^2+c*x(2).^2; % The parameterized function.
% c = 1.5; % The parameter.
% X = fminunc(@(x) f(x,c),[0.3;1])
%
% See also OPTIMSET, FMINSEARCH, FUNCTION_HANDLE.
% References:
% J. Nocedal and S. Wright, "Numerical Optimization", 2nd edition, Springer, 2006, pp. 140-145.
% D. F. Shanno and K. J. Phua, "Matrix conditioning and nonlinear optimization", Mathematics of Computation, Vol. 24, 1970, pp. 1095-1102.
% R. Fletcher, "A new approach to variable metric algorithms", Computer Journal, Vol. 13, 1970, pp. 317-322.
% R. Fletcher, "Practical Methods of Optimization", 2nd edition, Wiley, 1987, pp. 120-122.
% J. E. Dennis and D. J. Woods, "New Quasi-Newton Algorithms for the Optimization of Functions with Simple Bounds", SIAM Journal on Numerical Analysis, Vol. 9, 1972, pp. 617-625.
% D. Goldfarb, "A family of variable-metric methods derived by variational means", Mathematics of Computation, Vol. 24, 1970, pp. 23-26.
% D. Goldfarb and A. Idnani, "A numerically stable dual method for solving strictly convex quadratic programs", Mathematical Programming, Vol. 27, 1983, pp. 1-33.
% D. Goldfarb and A. Idnani, "On Steepest Descent for Unconstrained Optimization: A Comparison of Two Modifications", in "Recent Advances in Optimization Techniques", R. Fletcher, Ed., Academic Press, 1969, pp. 19-27.
% A. R. Conn, N. I. M. Gould, and Ph. L. Toint, "Trust Region Methods", SIAM, 2000.
% J. Nocedal, "Updating Quasi-Newton Matrices with Limited Storage", Mathematics of Computation, Vol. 35, 1980, pp. 773-782.
% D. Liu and J. Nocedal, "On the Limited Memory Method for Large Scale Optimization", Mathematical Programming B, Vol. 45, 1989, pp. 503-528.
% R. H. Byrd, P. Lu, J. Nocedal, and C. Zhu, "A Limited Memory Algorithm for Bound Constrained Optimization", SIAM Journal on Scientific Computing, Vol. 16, 1995, pp. 1190-1208.
% R. H. Byrd, J. Nocedal, and R. B. Schnabel, "Representations of Quasi-Newton Matrices and their use in Limited Memory Methods", Mathematical Programming B, Vol. 63, 1994, pp. 129-156.
% J. M. Martinez and A. Martinez, "A new quasi-Newton method for unconstrained optimization", Journal of Computational and Applied Mathematics, Vol. 234, 2010, pp. 883-893.
% Copyright 1984-2014 The MathWorks, Inc.
defaultopt = struct('Display','notify','GradObj','off','Hessian','off',...
'MaxFunEvals',100*numberOfVariables,'MaxIter',400,'TolFun',1e-6,...
'TolX',1e-6,'DerivativeCheck','off','Diagnostics','off',...
'FunValCheck','off','OutputFcn',[],'PlotFcns',[],'HessMult',[],...
'HessPattern','sparse(ones(numberOfVariables))',...
'InitialHessType','scaled-identity','InitialHessMatrix',[],...
'TypicalX','ones(numberOfVariables,1)','UseParallel',false,...
'ScaleProblem','none');
% If just 'defaults' passed in, return the default options in X
if nargin == 1 && nargout <= 1 && strcmpi(fun,'defaults')
x = defaultopt;
return
end
if nargin < 2
error(message('optim:fminunc:NotEnoughInputs'));
end
if ~ischar(fun) && ~isa(fun,'function_handle')
error(message('optim:fminunc:InvalidFun'));
end
if nargin < 3
options = [];
end
% Detect problem structure input
if nargin == 1 && isa(fun,'struct')
[fun,x0,options] = separateOptimStruct(fun);
end
% Check for non-double inputs
msg = isoptimargdbl('FMINUNC', {'X0','initial point'}, x0);
if ~isempty(msg)
error(msg);
end
% Check that X0 is a real vector or matrix.
if ~isreal(x0) || ~isvector(x0)
error(message('optim:fminunc:NonRealInitialPoint'));
end
% Check that X0 is not empty.
if isempty(x0)
error(message('optim:fminunc:EmptyInitialPoint'));
end
% Check that OPTIONS is a valid structure
if ~isempty(options) && ~isa(options,'struct')
error(message('optim:fminunc:InvalidOptions'));
end
% Get the options
[options,optimargs] = optimset(defaultopt,options);
% Check if the objective function is a GPU array function
[fun,haveOutputFcn] = gpuArrayFunFcnCheck(fun);
% Check for non-double inputs
msg = isoptimargdbl('FMINUNC', 'objective function', fun);
if ~isempty(msg)
error(msg);
end
% Check for non-double inputs in extra arguments
if ~isempty(varargin)
for i = 1:length(varargin)
msg = isoptimargdbl('FMINUNC', ['argument ' num2str(i)], varargin{i});
if ~isempty(msg)
error(msg);
end
end
end
% Check for non-double inputs in options structure
fminuncFields = {'TypicalX'};
for i = 1:length(fminuncFields)
if isfield(options,fminuncFields{i})
msg = isoptimargdbl('FMINUNC', fminuncFields{i}, options.(fminuncFields{i}));
if ~isempty(msg)
error(msg);
end
end
end
% Check for non-positive MaxFunEvals or MaxIter
if options.MaxFunEvals <= 0
error(message('optim:fminunc:InvalidMaxFunEvals'));
end
if options.MaxIter <= 0
error(message('optim:fminunc:InvalidMaxIter'));
end
% Check for invalid values of HessMult
if ~isempty(options.HessMult) && ~isa(options.HessMult,'function_handle')
error(message('optim:fminunc:InvalidHessMult'));
end
% Check for invalid values of HessPattern
if ~isempty(options.HessPattern) && ~isnumeric(options.HessPattern)
error(message('optim:fminunc:InvalidHessPattern'));
end
% Check for invalid values of InitialHessType
if ~isempty(options.InitialHessType) && ...
~(strcmpi(options.InitialHessType,'identity') || ...
strcmpi(options.InitialHessType,'scaled-identity') || ...
strcmpi(options.InitialHessType,'user-supplied'))
error(message('optim:fminunc:InvalidInitialHessType'));
end
% Check for invalid values of ScaleProblem
if ~(strcmpi(options.ScaleProblem,'none') || ...
strcmpi(options.ScaleProblem,'jacobian') || ...
strcmpi(options.ScaleProblem,'obj-and-jacobian'))
error(message('optim:fminunc:InvalidScaleProblem'));
end
% Check for invalid values of UseParallel
if ~(islogical(options.UseParallel) || ...
(isnumeric(options.UseParallel) && isscalar(options.UseParallel)))
error(message('optim:fminunc:InvalidUseParallel'));
end
% Check for invalid values of Diagnostics
if ~(strcmpi(options.Diagnostics,'off') || ...
strcmpi(options.Diagnostics,'on') || ...
strcmpi(options.Diagnostics,'iter') || ...
strcmpi(options.Diagnostics,'final'))
error(message('optim:fminunc:InvalidDiagnostics'));
end
% Check for invalid values of InitialHessMatrix
if ~isempty(options.InitialHessMatrix) && ~isnumeric(options.InitialHessMatrix)
error(message('optim:fminunc:InvalidInitialHessMatrix'));
end
% Check for invalid values of PlotFcns
if ~isempty(options.PlotFcns) && ~iscell(options.PlotFcns)
error(message('optim:fminunc:InvalidPlotFcns'));
end
% Check for invalid values of OutputFcn
if ~isempty(options.OutputFcn) && ~iscell(options.OutputFcn)
error(message('optim:fminunc:InvalidOutputFcn'));
end
% Check for invalid values of DerivativeCheck
if ~(strcmpi(options.DerivativeCheck,'off') || ...
strcmpi(options.DerivativeCheck,'on') || ...
strcmpi(options.DerivativeCheck,'first-order') || ...
strcmpi(options.DerivativeCheck,'second-order'))
error(message('optim:fminunc:InvalidDerivativeCheck'));
end
% Check for invalid values of FunValCheck
if ~(strcmpi(options.FunValCheck,'off') || ...
strcmpi(options.FunValCheck,'on'))
error(message('optim:fminunc:InvalidFunValCheck'));
end
% Check for invalid values of LargeScale
if ~(strcmpi(options.LargeScale,'off') || ...
strcmpi(options.LargeScale,'on'))
error(message('optim:fminunc:InvalidLargeScale'));
end
% Check for invalid values of GradObj
if ~(strcmpi(options.GradObj,'off') || ...
strcmpi(options.GradObj,'on'))
error(message('optim:fminunc:InvalidGradObj'));
end
% Check for invalid values of Hessian
if ~(strcmpi(options.Hessian,'off') || ...
strcmpi(options.Hessian,'on'))
error(message('optim:fminunc:InvalidHessian'));
end
% Check for invalid values of HessMult
if ~isempty(options.HessMult) && ~isa(options.HessMult,'function_handle')
error(message('optim:fminunc:InvalidHessMult'));
end
% Check for invalid values of HessPattern
if ~isempty(options.HessPattern) && ~isnumeric(options.HessPattern)
error(message('optim:fminunc:InvalidHessPattern'));
end
% Check for invalid values of InitialHessType
if ~isempty(options.InitialHessType) && ...
~(strcmpi(options.InitialHessType,'identity') || ...
strcmpi(options.InitialHessType,'scaled-identity') || ...
strcmpi(options.InitialHessType,'user-supplied'))
error(message('optim:fminunc:InvalidInitialHessType'));
end
% Check for invalid values of ScaleProblem
if ~(strcmpi(options.ScaleProblem,'none') || ...
strcmpi(options.ScaleProblem,'jacobian') || ...
strcmpi(options.ScaleProblem,
相关推荐









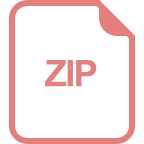
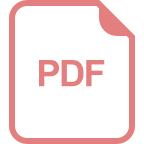