matlab牛顿法求根
时间: 2024-03-20 19:37:19 浏览: 87
牛顿法是一种常用的数值计算方法,用于求解方程的根。在MATLAB中,可以使用牛顿法求解方程的根。下面是使用MATLAB实现牛顿法求根的步骤:
1. 定义方程:首先需要定义要求解根的方程。可以使用函数句柄或者匿名函数来表示方程。
2. 初始化:选择一个初始点作为起始点。
3. 迭代计算:根据牛顿法的迭代公式进行迭代计算,直到满足停止条件。
4. 停止条件:可以选择迭代次数达到一定值或者迭代结果的精度满足要求作为停止条件。
5. 输出结果:输出最终的根值。
下面是一个使用MATLAB实现牛顿法求根的示例代码:
```matlab
% 定义方程
f = @(x) x^3 - 2*x - 5;
% 定义方程的导数
df = @(x) 3*x^2 - 2;
% 初始化
x0 = 2; % 初始点
% 迭代计算
maxIter = 100; % 最大迭代次数
tol = 1e-6; % 迭代结果的精度要求
iter = 0; % 迭代次数计数器
x = x0; % 当前迭代点
while abs(f(x)) > tol && iter < maxIter
x = x - f(x) / df(x); % 牛顿法迭代公式
iter = iter + 1;
end
% 输出结果
root = x;
disp(['方程的根为:', num2str(root)]);
```
相关问题
matlab牛顿法求根程序
牛顿法是一种用于求解非线性方程的迭代算法,在数值计算中得到广泛应用。下面是用MATLAB实现牛顿法求根的程序示例:
```matlab
function [root, iter] = newton_method(func, initial_guess, tolerance)
% func为输入的目标函数
% initial_guess为初始猜测值
% tolerance为容差,控制迭代的停止条件
% 迭代次数初始化为0
iter = 0;
% 初始化根的值为初始猜测值
root = initial_guess;
% 使用while循环进行迭代计算
while true
% 当前的函数值和导数值
f = func(root);
f_prime = diff(func);
% 计算下一次迭代的根的值
next_root = root - f / f_prime(root);
% 判断当前迭代结果是否满足容差条件
if abs(next_root - root) < tolerance
break; % 满足条件,停止迭代
end
% 更新根的值
root = next_root;
% 迭代次数加1
iter = iter + 1;
end
end
```
使用上述代码,可以通过调用`newton_method`函数来求解非线性方程的根。其中`func`为目标函数,`initial_guess`为初始猜测值,`tolerance`为容差。函数返回的`root`为计算得到的根的值,`iter`为迭代次数。
matlab牛顿法求根例题截图
很抱歉,根据提供的引用内容,没有给出matlab牛顿法求根例题的截图。但是,可以为您提供一个matlab牛顿法求根的例子代码,您可以自行尝试运行并查看结果。
```matlab
% 定义函数f(x)和f'(x)
f = @(x) x^3 - 2*x - 5;
df = @(x) 3*x^2 - 2;
% 初值和误差限
x0 = 2;
e = 1e-6;
% 迭代求解
k = 1;
x = x0;
x0 = x + e*2;
while (abs(x-x0))>e && (k<=N)
x0 = x;
x = x0 - f(x0)/df(x0);
k = k+1;
end
% 输出结果
fprintf('迭代次数:%d\n', k);
fprintf('方程的根为:%f\n', x);
```
阅读全文
相关推荐
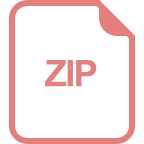
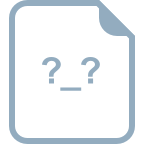

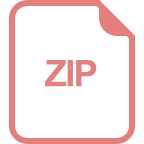











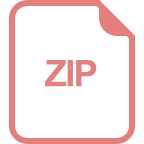