Calculating s=1+(1+2)+(1+2+3)+... ...+(1+2+...+n)
时间: 2023-11-14 22:06:01 浏览: 107
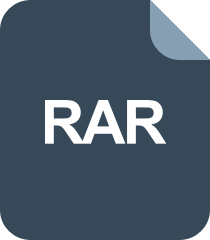
计算 S=1!+2!+3!+...+N!。
这个问题可以通过编写一个函数来解决。下面是两个不同的函数实现方法:
1. 使用循环计算每个子序列的和,然后将它们相加得到最终结果。
2. 使用递归计算每个子序列的和,然后将它们相加得到最终结果。
方法1的代码如下:
```
long long sum(int n) {
long long result = 0;
for (int i = 1; i <= n; i++) {
long long sub_sum = 0;
for (int j = 1; j <= i; j++) {
sub_sum += j;
}
result += sub_sum;
}
return result;
}
```
方法2的代码如下:
```
long long sub_sum(int n) {
if (n == 1) {
return 1;
} else {
return sub_sum(n - 1) + n * (n + 1) / 2;
}
}
long long sum(int n) {
long long result = 0;
for (int i = 1; i <= n; i++) {
result += sub_sum(i);
}
return result;
}
```
请注意,这两个函数都使用了长整型来处理大数字。同时,第二个函数使用了递归来计算每个子序列的和。这两个函数都可以用来计算s=1+(1+2)+(1+2+3)+... ...+(1+2+...+n)。
阅读全文
相关推荐















