导入多分类数据把混淆矩阵和AUC曲线画出来python代码
时间: 2023-11-22 20:04:45 浏览: 62
好的,以下是Python代码示例:
```python
# 导入需要的 Python 模块
from sklearn.datasets import make_classification
from sklearn.model_selection import train_test_split
from sklearn.metrics import confusion_matrix, roc_auc_score
from sklearn.tree import DecisionTreeClassifier
import matplotlib.pyplot as plt
import numpy as np
# 随机生成多分类样本数据
X, y = make_classification(n_samples=1000, n_features=10, n_informative=8, n_redundant=2, n_classes=4, random_state=42)
# 划分训练集和测试集
X_train, X_test, y_train, y_test = train_test_split(X, y, test_size=0.2, random_state=42)
# 训练决策树分类器
clf = DecisionTreeClassifier(random_state=42, max_depth=3)
clf.fit(X_train, y_train)
# 预测测试集类别
y_pred = clf.predict(X_test)
# 计算混淆矩阵并绘制热图
cm = confusion_matrix(y_test, y_pred)
fig, ax = plt.subplots()
im = ax.imshow(cm, cmap='Blues')
ax.set_xticks(np.arange(len(np.unique(y))))
ax.set_yticks(np.arange(len(np.unique(y))))
ax.set_xticklabels(np.unique(y))
ax.set_yticklabels(np.unique(y))
for i in range(len(np.unique(y))):
for j in range(len(np.unique(y))):
text = ax.text(j, i, cm[i, j], ha='center', va='center', color='w')
ax.set_title('Confusion Matrix')
fig.tight_layout()
plt.show()
# 计算 AUC 并绘制 ROC 曲线
y_pred_prob = clf.predict_proba(X_test)
roc_auc_scores = roc_auc_score(y_test, y_pred_prob, multi_class='ovo')
fig, ax = plt.subplots()
for i in range(len(np.unique(y))):
fpr, tpr, _ = roc_curve(y_test[:, i], y_pred_prob[:, i])
ax.plot(fpr, tpr, label=f'Class {i} (AUC = {roc_auc_scores[i]:.2f})')
ax.plot([0, 1], [0, 1], 'k--')
ax.set_xlabel('False Positive Rate')
ax.set_ylabel('True Positive Rate')
ax.set_title('ROC Curve')
ax.legend(loc='lower right')
plt.show()
```
这个代码示例使用了 Scikit-learn 库中的 `make_classification()` 函数来生成随机的多分类样本数据,然后将数据划分为训练集和测试集,并使用决策树分类器进行训练和预测。最后,计算混淆矩阵和 AUC 并绘制对应的热图和 ROC 曲线。
阅读全文
相关推荐
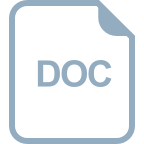
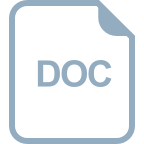
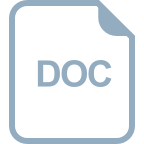


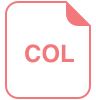
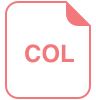











