a = np.array([[1,2],[3,4]]) b = np.array([[5,6],[7,8]]) print (np.stack((a,b),1))
时间: 2023-09-13 16:05:57 浏览: 178
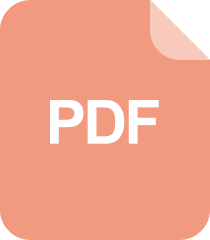
浅谈numpy中np.array()与np.asarray的区别以及.tolist

这段代码的功能是将两个2x2的NumPy数组在第2个维度上进行堆叠,并输出结果。具体来说,它将a和b堆叠成一个2x2x2的数组,其中第一个维度是原始数组的索引(0代表a,1代表b),第二个维度是原始数组的行号,第三个维度是原始数组的列号。输出结果如下:
```
array([[[1, 2],
[5, 6]],
[[3, 4],
[7, 8]]])
```
在第二个维度上,它将a和b的第一行和第二行堆叠在一起,形成了一个2x2的矩阵。
阅读全文
相关推荐
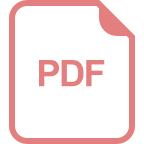
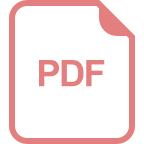















