class Mushybox(Atoms): def __init__(self, atomsx, express=np.zeros((3, 3)), fixstrain=np.ones((3, 3))): """box relaxation atomsx: an Atoms object express: external pressure, a 3*3 lower triangular matrix in the unit of GPa; define positive values as compression fixstrain: 3*3 matrix as express. 0 fixes strain at the corresponding direction """
时间: 2024-02-14 14:32:20 浏览: 209
这是一个自定义的`Mushybox`类,继承于ASE中的`Atoms`类,并添加了一些自定义的功能。具体来说,这个类实现了一个晶胞弛豫的功能,可以通过给定一个外部压力和一个固定应变的矩阵,来对晶格常数进行优化。
在这个类的构造函数`__init__`中,有三个参数:
- `atomsx`:一个`Atoms`对象,表示需要进行晶胞优化的初始结构;
- `express`:一个3x3的下三角矩阵,表示外部施加的压力,以GPa为单位。这里定义正值表示压缩,负值表示拉伸;
- `fixstrain`:一个3x3的矩阵,表示固定应变的矩阵。矩阵中的元素为0表示该方向上的应变被固定,为1表示该方向上的应变可以自由变化。
根据这三个参数,`Mushybox`类可以实现晶胞弛豫的功能,并返回优化后的结构。
需要注意的是,这个类仅为示例代码,实际使用时应根据具体问题进行算法和参数的选择,并根据需要进行自定义设置,以获得最佳的优化效果和计算效率。
相关问题
解释class GraphMLPEncoder(FairseqEncoder): def __init__(self, args): super().__init__(dictionary=None) self.max_nodes = args.max_nodes self.emb_dim = args.encoder_embed_dim self.num_layer = args.encoder_layers self.num_classes = args.num_classes self.atom_encoder = GraphNodeFeature( num_heads=1, num_atoms=512*9, num_in_degree=512, num_out_degree=512, hidden_dim=self.emb_dim, n_layers=self.num_layer, ) self.linear = torch.nn.ModuleList() self.batch_norms = torch.nn.ModuleList() for layer in range(self.num_layer): self.linear.append(torch.nn.Linear(self.emb_dim, self.emb_dim)) self.batch_norms.append(torch.nn.BatchNorm1d(self.emb_dim)) self.graph_pred_linear = torch.nn.Linear(self.emb_dim, self.num_classes)
这段代码定义了一个名为GraphMLPEncoder的类,该类继承自FairseqEncoder类。在初始化方法中,它首先调用父类的初始化方法,并将dictionary参数设为None。然后,它从args参数中获取一些配置信息,如最大节点数(max_nodes)、嵌入维度(emb_dim)、编码器层数(num_layer)和类别数(num_classes)。
接下来,它创建了一个名为atom_encoder的GraphNodeFeature对象,该对象用于对图节点特征进行编码。它具有一些参数,如头数(num_heads)、原子数(num_atoms)、入度数(num_in_degree)、出度数(num_out_degree)、隐藏维度(hidden_dim)和层数(n_layers)。
然后,它创建了两个列表:linear和batch_norms。这些列表用于存储线性层和批归一化层的实例。它通过循环来创建多个线性层和批归一化层,并将它们添加到相应的列表中。
最后,它创建了一个线性层graph_pred_linear,该层将嵌入维度映射到类别数。这个线性层用于图预测任务中的分类操作。
Traceback (most recent call last): File "ADF.py", line 18, in <module> atoms.set_calculator(SinglePointCalculator(atoms, energy=0, forces=np.zeros((len(atoms), 3)), stress=np.zeros(6), neighborlist=nl)) File "/export/home/anliying/.local/lib/python3.8/site-packages/ase-3.22.1-py3.8.egg/ase/calculators/singlepoint.py", line 22, in init assert property in all_properties AssertionError。import numpy as np from ase.io import read from ase.build import make_supercell from ase.visualize import view from ase.neighborlist import NeighborList from ase.calculators.singlepoint import SinglePointCalculator # 读入三个POSCAR文件,计算原子分布函数 structures = [] for file in ['structure1.cif', 'structure2.cif', 'structure3.cif']: atoms = read(file) # 构造超胞,避免周期性边界对ADF计算的影响 atoms = make_supercell(atoms, [[2, 0, 0], [0, 2, 0], [0, 0, 2]]) # 计算邻居列表 nl = NeighborList([1.2] * len(atoms), self_interaction=False) nl.update(atoms) # 将邻居列表传递给SinglePointCalculator atoms.set_calculator(SinglePointCalculator(atoms, energy=0, forces=np.zeros((len(atoms), 3)), stress=np.zeros(6), neighborlist=nl)) # 计算原子分布函数 adf = atoms.get_atomic_distribution_function() structures.append(adf) # 将ADF转化为特征矩阵 bins = np.linspace(0, 10, num=100) # 分100个bin adf_hists = [np.histogram(adf, bins=bins)[0] for adf in structures] feature_matrix = np.array(adf_hists) / [len(atoms) for atoms in structures] # 归一化特征矩阵 feature_matrix = feature_matrix / np.linalg.norm(feature_matrix, axis=1, keepdims=True),基于错误改代码
It seems that the error occurs because the `property` argument is not recognized by the `SinglePointCalculator` constructor.
To fix this error, you can replace the `property` argument with `properties` in the `SinglePointCalculator` constructor. The corrected code should look like this:
```
atoms.set_calculator(SinglePointCalculator(atoms, energy=0, forces=np.zeros((len(atoms), 3)), stress=np.zeros(6), properties=['energy', 'forces'], neighborlist=nl))
```
Note that I have also added the `properties` argument with the values `['energy', 'forces']` to specify the properties that the calculator should compute.
阅读全文
相关推荐
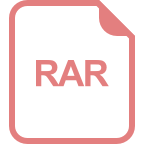
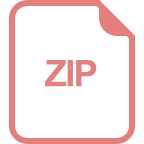
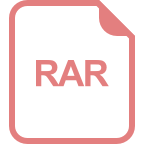














