def r_squared(self, y_true, y_pred): ss_res = np.sum((y_true - y_pred) ** 2) ss_tot = np.sum((y_true - np.mean(y_true)) ** 2) print(1 - (ss_res / ss_tot)) return 1 - (ss_res / ss_tot) 详细解释一下,并告诉我评价标准
时间: 2024-04-07 12:28:09 浏览: 60
这是一个计算R平方值的函数,用于评估一个回归模型的好坏程度。该函数的输入包括:
- y_true:形状为(n_samples,)的目标向量,其中n_samples是样本数。
- y_pred:形状为(n_samples,)的预测结果向量,其中n_samples是样本数。
该函数的输出是一个R平方值,它是一个介于0和1之间的数值,数值越大表示模型的拟合效果越好,最大值为1。具体地,R平方值表示模型所解释的响应变量方差的比例。如果R平方值接近1,则表示模型能够解释目标变量中大部分的方差,说明拟合效果很好;如果R平方值接近0,则表示模型未能解释目标变量中大部分的方差,说明拟合效果很差。
该函数的实现逻辑是,首先计算残差平方和(SS_res)和总平方和(SS_tot),然后用1减去它们的比值,从而得到R平方值。其中,残差平方和表示模型预测值与目标变量之间的误差平方和,而总平方和表示目标变量离其平均值的偏差平方和。
需要注意的是,R平方值只适用于线性回归模型或其他类似线性模型,并且适用于连续型目标变量。对于其他类型的模型或目标变量,可能需要使用其他的评估指标。
相关问题
解决以下报错:--------------------------------------------------------------------------- TypeError Traceback (most recent call last) Cell In[40], line 387 380 save_intoCSV(Results, './Results_MSE.csv') 386 if __name__ == '__main__': --> 387 main() Cell In[40], line 334, in main() 331 test_MSE = test_total_MSE / (test_count * 1.0) 333 print("Evaluating...") --> 334 evaluate_forecast(y_true,y_pred) 336 # Update results or break? 337 if (test_MSE < test_best_MSE): Cell In[40], line 35, in evaluate_forecast(y_true, y_pred) 31 def evaluate_forecast(y_true, y_pred): 32 #rmse = mean_squared_error(y_true, y_pred, squared=False) 33 #mae = mean_absolute_error(y_true, y_pred) 34 #mape = np.mean(np.abs((y_true - y_pred) / y_true)) * 100 ---> 35 rse=np.sqrt(np.sum(np.square(y_true - y_pred))) / np.sqrt(np.sum(np.square(y_true - np.mean(y_true)))) 36 rae=np.sum(np.abs(y_true - y_pred)) / np.sum(np.abs(y_true - np.mean(y_true))) 37 # 将输入数据转换为numpy数组 TypeError: unsupported operand type(s) for -: 'list' and 'list'
这个报错是因为在 evaluate_forecast 函数中,y_true 和 y_pred 是列表类型,而 np.square 和 np.sum 函数只能作用于 numpy 数组。因此,需要将 y_true 和 y_pred 转换为 numpy 数组。
可以使用以下代码将列表转换为 numpy 数组:
```python
import numpy as np
y_true = np.array(y_true)
y_pred = np.array(y_pred)
```
将此代码添加到 evaluate_forecast 函数的开始部分,即可解决该报错。
class NeuralNetwork: def init(self, input_dim, hidden_dim, output_dim): self.input_dim = input_dim self.hidden_dim = hidden_dim self.output_dim = output_dim self.weights1 = np.random.randn(input_dim, hidden_dim) self.bias1 = np.zeros((1, hidden_dim)) self.weights2 = np.random.randn(hidden_dim, output_dim) self.bias2 = np.zeros((1, output_dim)) def relu(self, x): return np.maximum(0, x) def relu_derivative(self, x): return np.where(x >= 0, 1, 0) def forward(self, x): self.z1 = np.dot(x, self.weights1) + self.bias1 self.a1 = self.relu(self.z1) self.z2 = np.dot(self.a1, self.weights2) + self.bias2 self.y_hat = self.z2 return self.y_hat def backward(self, x, y, learning_rate): error = self.y_hat - y delta2 = error delta1 = np.dot(delta2, self.weights2.T) * self.relu_derivative(self.a1) grad_weights2 = np.dot(self.a1.T, delta2) grad_bias2 = np.sum(delta2, axis=0, keepdims=True) grad_weights1 = np.dot(x.T, delta1) grad_bias1 = np.sum(delta1, axis=0) self.weights2 -= learning_rate * grad_weights2 self.bias2 -= learning_rate * grad_bias2 self.weights1 -= learning_rate * grad_weights1 根据代码加上损失函数和优化
函数,可以将这个神经网络变为完整的模型。一种常见的损失函数是均方误差(Mean Squared Error),可以用于回归问题:
```python
class NeuralNetwork:
def __init__(self, input_dim, hidden_dim, output_dim):
self.input_dim = input_dim
self.hidden_dim = hidden_dim
self.output_dim = output_dim
self.weights1 = np.random.randn(input_dim, hidden_dim)
self.bias1 = np.zeros((1, hidden_dim))
self.weights2 = np.random.randn(hidden_dim, output_dim)
self.bias2 = np.zeros((1, output_dim))
def relu(self, x):
return np.maximum(0, x)
def relu_derivative(self, x):
return np.where(x >= 0, 1, 0)
def forward(self, x):
self.z1 = np.dot(x, self.weights1) + self.bias1
self.a1 = self.relu(self.z1)
self.z2 = np.dot(self.a1, self.weights2) + self.bias2
self.y_hat = self.z2
return self.y_hat
def backward(self, x, y, learning_rate):
error = self.y_hat - y
delta2 = error
delta1 = np.dot(delta2, self.weights2.T) * self.relu_derivative(self.a1)
grad_weights2 = np.dot(self.a1.T, delta2)
grad_bias2 = np.sum(delta2, axis=0, keepdims=True)
grad_weights1 = np.dot(x.T, delta1)
grad_bias1 = np.sum(delta1, axis=0)
self.weights2 -= learning_rate * grad_weights2
self.bias2 -= learning_rate * grad_bias2
self.weights1 -= learning_rate * grad_weights1
self.bias1 -= learning_rate * grad_bias1
def mse_loss(self, y, y_hat):
return np.mean((y - y_hat)**2)
def sgd_optimizer(self, x, y, learning_rate):
y_hat = self.forward(x)
loss = self.mse_loss(y, y_hat)
self.backward(x, y, learning_rate)
return loss
```
在这个模型中,我们添加了 `mse_loss` 函数,用于计算均方误差,同时添加了 `sgd_optimizer` 函数,用于执行随机梯度下降优化算法。在每次迭代中,我们计算预测值 `y_hat`,然后计算损失值并执行反向传播算法更新神经网络的权重和偏置。最后,我们返回损失值作为当前迭代的结果。根据需要,我们可以使用其他损失函数和优化器来训练这个神经网络。
阅读全文
相关推荐


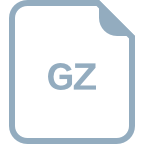














