if class_list.count(class_list[0]) == len(class_list):
时间: 2024-02-18 12:03:03 浏览: 21
这是一个条件语句,用于判断数据集中所有实例是否属于同一类别。具体来说,该条件语句中的`class_list`是一个列表,其中包含了数据集中所有实例的类别信息。该条件语句首先使用`count`函数计算列表中第一个元素出现的次数,如果该次数等于列表长度,则说明所有实例的类别都相同,即所有实例属于同一类别。下面是该条件语句的语法格式:
```
if 条件:
代码块
```
其中`条件`可以是任何返回布尔值的表达式,如果该表达式的值为`True`,则执行代码块中的内容。如果该条件语句的条件成立,即所有实例属于同一类别,则执行条件语句后面的代码块,通常是返回该类别作为叶子节点的分类结果。
相关问题
为每句代码做注释:flower_list = train_dataset.class_to_idx cla_dict = dict((val, key) for key, val in flower_list.items()) json_str = json.dumps(cla_dict, indent=4) with open('class_indices.json', 'w') as json_file: json_file.write(json_str) batch_size = 16 nw = min([os.cpu_count(), batch_size if batch_size > 1 else 0, 8]) print('Using {} dataloader workers every process'.format(nw)) train_loader = torch.utils.data.DataLoader(train_dataset, batch_size=batch_size, shuffle=True, num_workers=0) validate_dataset = datasets.ImageFolder(root=os.path.join(image_path, "val"), transform=data_transform["val"]) val_num = len(validate_dataset) validate_loader = torch.utils.data.DataLoader(validate_dataset, batch_size=batch_size, shuffle=False, num_workers=0) print("using {} images for training, {} images for validation.".format(train_num, val_num))
# 创建一个包含所有花的列表,列表中每个元素都是一种花的类别索引
flower_list = train_dataset.class_to_idx
# 将key和value互换位置,将花的类别索引转换为花的名称
cla_dict = dict((val, key) for key, val in flower_list.items())
# 将字典转换为json格式的字符串
json_str = json.dumps(cla_dict, indent=4)
# 将json字符串写入文件class_indices.json中
with open('class_indices.json', 'w') as json_file:
json_file.write(json_str)
# 设置批大小为16,设置dataloader的worker数量,确保不超过CPU核心数和批大小
batch_size = 16
nw = min([os.cpu_count(), batch_size if batch_size > 1 else 0, 8])
print('Using {} dataloader workers every process'.format(nw))
# 创建训练集dataloader
train_loader = torch.utils.data.DataLoader(train_dataset, batch_size=batch_size, shuffle=True, num_workers=0)
# 创建验证集dataset和dataloader
validate_dataset = datasets.ImageFolder(root=os.path.join(image_path, "val"), transform=data_transform["val"])
val_num = len(validate_dataset)
validate_loader = torch.utils.data.DataLoader(validate_dataset, batch_size=batch_size, shuffle=False, num_workers=0)
# 打印训练集和验证集的图片数量
print("using {} images for training, {} images for validation.".format(train_num, val_num))
class AbstractGreedyAndPrune(): def __init__(self, aoi: AoI, uavs_tours: dict, max_rounds: int, debug: bool = True): self.aoi = aoi self.max_rounds = max_rounds self.debug = debug self.graph = aoi.graph self.nnodes = self.aoi.n_targets self.uavs = list(uavs_tours.keys()) self.nuavs = len(self.uavs) self.uavs_tours = {i: uavs_tours[self.uavs[i]] for i in range(self.nuavs)} self.__check_depots() self.reachable_points = self.__reachable_points() def __pruning(self, mr_solution: MultiRoundSolution) -> MultiRoundSolution: return utility.pruning_multiroundsolution(mr_solution) def solution(self) -> MultiRoundSolution: mrs_builder = MultiRoundSolutionBuilder(self.aoi) for uav in self.uavs: mrs_builder.add_drone(uav) residual_ntours_to_assign = {i : self.max_rounds for i in range(self.nuavs)} tour_to_assign = self.max_rounds * self.nuavs visited_points = set() while not self.greedy_stop_condition(visited_points, tour_to_assign): itd_uav, ind_tour = self.local_optimal_choice(visited_points, residual_ntours_to_assign) residual_ntours_to_assign[itd_uav] -= 1 tour_to_assign -= 1 opt_tour = self.uavs_tours[itd_uav][ind_tour] visited_points |= set(opt_tour.targets_indexes) # update visited points mrs_builder.append_tour(self.uavs[itd_uav], opt_tour) return self.__pruning(mrs_builder.build()) class CumulativeGreedyCoverage(AbstractGreedyAndPrune): choice_dict = {} for ind_uav in range(self.nuavs): uav_residual_rounds = residual_ntours_to_assign[ind_uav] if uav_residual_rounds > 0: uav_tours = self.uavs_tours[ind_uav] for ind_tour in range(len(uav_tours)): tour = uav_tours[ind_tour] quality_tour = self.evaluate_tour(tour, uav_residual_rounds, visited_points) choice_dict[quality_tour] = (ind_uav, ind_tour) best_value = max(choice_dict, key=int) return choice_dict[best_value] def evaluate_tour(self, tour : Tour, round_count : int, visited_points : set): new_points = (set(tour.targets_indexes) - visited_points) return round_count * len(new_points) 如何改写上述程序,使其能返回所有已经探索过的目标点visited_points的数量,请用代码表示
可以在 `solution()` 方法中添加一个变量来记录已经探索过的目标点数量,然后在每次更新 `visited_points` 后更新这个变量。下面是修改后的代码:
```
class AbstractGreedyAndPrune():
def __init__(self, aoi: AoI, uavs_tours: dict, max_rounds: int, debug: bool = True):
self.aoi = aoi
self.max_rounds = max_rounds
self.debug = debug
self.graph = aoi.graph
self.nnodes = self.aoi.n_targets
self.uavs = list(uavs_tours.keys())
self.nuavs = len(self.uavs)
self.uavs_tours = {i: uavs_tours[self.uavs[i]] for i in range(self.nuavs)}
self.__check_depots()
self.reachable_points = self.__reachable_points()
def __pruning(self, mr_solution: MultiRoundSolution) -> MultiRoundSolution:
return utility.pruning_multiroundsolution(mr_solution)
def solution(self) -> Tuple[MultiRoundSolution, int]:
mrs_builder = MultiRoundSolutionBuilder(self.aoi)
for uav in self.uavs:
mrs_builder.add_drone(uav)
residual_ntours_to_assign = {i : self.max_rounds for i in range(self.nuavs)}
tour_to_assign = self.max_rounds * self.nuavs
visited_points = set()
explored_points = 0
while not self.greedy_stop_condition(visited_points, tour_to_assign):
itd_uav, ind_tour = self.local_optimal_choice(visited_points, residual_ntours_to_assign)
residual_ntours_to_assign[itd_uav] -= 1
tour_to_assign -= 1
opt_tour = self.uavs_tours[itd_uav][ind_tour]
new_points = set(opt_tour.targets_indexes) - visited_points
explored_points += len(new_points)
visited_points |= new_points # update visited points
mrs_builder.append_tour(self.uavs[itd_uav], opt_tour)
return self.__pruning(mrs_builder.build()), explored_points
class CumulativeGreedyCoverage(AbstractGreedyAndPrune):
def evaluate_tour(self, tour : Tour, round_count : int, visited_points : set):
new_points = set(tour.targets_indexes) - visited_points
return round_count * len(new_points)
def local_optimal_choice(self, visited_points, residual_ntours_to_assign):
choice_dict = {}
for ind_uav in range(self.nuavs):
uav_residual_rounds = residual_ntours_to_assign[ind_uav]
if uav_residual_rounds > 0:
uav_tours = self.uavs_tours[ind_uav]
for ind_tour in range(len(uav_tours)):
tour = uav_tours[ind_tour]
quality_tour = self.evaluate_tour(tour, uav_residual_rounds, visited_points)
choice_dict[quality_tour] = (ind_uav, ind_tour)
best_value = max(choice_dict, key=int)
return choice_dict[best_value]
相关推荐
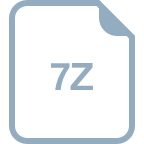
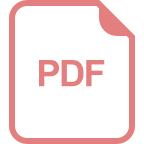
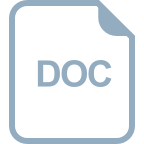












