s_j1_batch = tf.stack(s_j1_batch) readout_j1_batch = model(s_j1_batch, training=True)
时间: 2023-11-01 13:06:52 浏览: 26
这段代码是使用 TensorFlow 实现神经网络模型的前向传播过程,其中`s_j1_batch`是输入数据的一个 batch,`model`是已经定义好的神经网络模型,`training=True`表示在训练模式下进行前向传播。
具体来说,`tf.stack(s_j1_batch)`将`batch`中的每个样本堆叠成一个张量,形状为`(batch_size, input_size)`,作为模型的输入。`model`的输入是一个张量,输出是一个形状为`(batch_size, output_size)`的张量,表示对每个样本的预测结果。`readout_j1_batch`保存了模型对`batch`中所有样本的预测结果。
相关问题
s_j1_batch = tf.stack(s_j1_batch)
这行代码是使用 TensorFlow 的 stack 函数将一个 Python 列表 s_j1_batch 中的所有元素按照第一个维度进行堆叠,返回一个新的张量。具体来说,如果 s_j1_batch 是一个形状为 [batch_size, seq_len, embedding_dim] 的列表,那么 stack(s_j1_batch) 的形状将是 [batch_size, seq_len, embedding_dim, len(s_j1_batch)],其中 len(s_j1_batch) 表示列表中元素的数量。这个操作通常用于将多个批次的数据合并成一个更大的批次。
s_j1_batch = tf.stack(s_j1_batch) readout_j1_batch = model(s_j1_batch, training=True)
这段代码使用 TensorFlow 的函数 `tf.stack()` 将一个 Python 列表 `s_j1_batch` 中的多个张量按照指定的维度进行堆叠,生成一个新的张量。其中 `s_j1_batch` 列表中的每个张量都是输入模型的一个 batch,这些 batch 可能具有不同的大小(例如,不同的句子长度)。堆叠这些 batch 之后,可以一次性地将它们输入到模型中进行计算,提高计算效率。
`model(s_j1_batch, training=True)` 则是对堆叠后的 batch 进行预测,其中 `model` 是一个 TensorFlow 模型,`training=True` 表示当前是训练模式。该代码的输出是一个张量 `readout_j1_batch`,它是模型的预测结果。
相关推荐
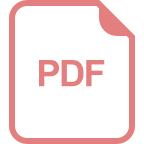
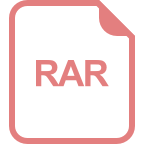














