self._Z = []为什么会出现错误
时间: 2023-09-25 21:12:00 浏览: 42
在代码中,self._Z = []是将_Z属性初始化为空列表,但是在getZ方法中,self._Z被赋值成为一个矩阵,所以可能会出现错误。
为了避免这种错误,应该在初始化时将self._Z初始化为空矩阵,而不是空列表,可以将类的初始化方法修改为以下内容:
```python
def __init__(self):
self._Z = np.mat([])
self._B = []
self._eigenVector = []
```
这样就可以避免在getZ方法中出现错误。同时,注意在其他方法中对self._Z的使用,需要先判断self._Z是否为空矩阵,避免出现使用空矩阵的错误。
相关问题
x = self.x_origin + (j + 0.5) * self.pixel_width y = self.y_origin + (i + 0.5) * self.pixel_height z = val self.iteSize += 1 return x, y, z dsm_path = 'C:\sanwei\jianmo\Productions\Production_2\Production_2_DSM_part_2_2.tif' dsm_data = gdal.Open(dsm_path) dsm_array = dsm_data.ReadAsArray() spiral_iterator = SpiralIterator(dsm_array,x=810,y=500) while spiral_iterator.hasNext(): x, y, z = spiral_iterator.get() print(f'Value at ({x},{y}):{z}')这段代码怎么改可以将打印的坐标保存为文档
你可以将打印出来的坐标以及对应的值写入到一个文本文件中,方法和上面类似。以下是修改后的代码示例:
```python
import gdal
class SpiralIterator:
def __init__(self, array, x=0, y=0):
self.array = array
self.x_origin = x
self.y_origin = y
self.pixel_width = 1
self.pixel_height = 1
self.iteSize = 0
self.i = 0
self.j = 0
self.di = 0
self.dj = 1
self.width = array.shape[1]
self.height = array.shape[0]
def hasNext(self):
return self.iteSize < self.width * self.height
def get(self):
val = self.array[self.i, self.j]
x = self.x_origin + (self.j + 0.5) * self.pixel_width
y = self.y_origin + (self.i + 0.5) * self.pixel_height
self.iteSize += 1
self.i, self.j, self.di, self.dj = self.getNextIndex()
return x, y, val
def getNextIndex(self):
if (self.i + self.di < 0 or self.i + self.di == self.height
or self.j + self.dj < 0 or self.j + self.dj == self.width
or self.array[self.i + self.di, self.j + self.dj] is None):
self.di, self.dj = self.dj, -self.di
return self.i + self.di, self.j + self.dj, self.di, self.dj
dsm_path = r'C:\sanwei\jianmo\Productions\Production_2\Production_2_DSM_part_2_2.tif'
dsm_data = gdal.Open(dsm_path)
dsm_array = dsm_data.ReadAsArray()
spiral_iterator = SpiralIterator(dsm_array, x=810, y=500)
# 打开文件
with open('coordinates.txt', 'w') as f:
while spiral_iterator.hasNext():
x, y, z = spiral_iterator.get()
print(f'Value at ({x},{y}): {z}')
# 将坐标和对应的值写入文件
f.write(f'({x},{y}): {z}\n')
print('Done!')
```
在这个例子中,我们使用了Python的with语句来打开文件,它可以自动管理文件的打开和关闭,避免了手动关闭文件时可能出现的错误。在while循环中,我们将打印的坐标和对应的值写入到了文件中。文件的名字可以根据你的需要自行修改。运行结束后,你可以在相应的目录下找到保存好的coordinates.txt文件。
VALID_MODULE_NAME = re.compile(r'[_a-z]\w*\.py$', re.IGNORECASE) class _FailedTest(case.TestCase): _testMethodName = None def __init__(self, method_name, exception): self._exception = exception super(_FailedTest, self).__init__(method_name) def __getattr__(self, name): if name != self._testMethodName: return super(_FailedTest, self).__getattr__(name) def testFailure(): raise self._exception return testFailure
这段代码是 Python 的 unittest 模块中的一部分。它定义了一个名为 _FailedTest 的类,这个类继承自 TestCase 类,用于测试用例执行失败时的处理。它包含一个 testFailure 方法,当测试用例执行失败时,该方法会被调用并且抛出 _exception 异常,_exception 是在 _FailedTest 的 __init__ 方法中传入的。这个类的作用是为了在测试用例执行失败时,能够更好地处理和报告错误。
相关推荐
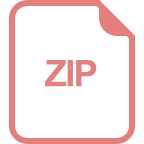
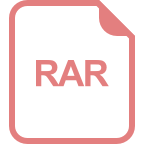
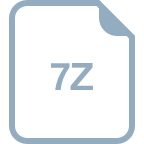













