请帮我解读这段代码if __name__ == "__main__": data_split_train_val_test(data_root='data', data_set='human') data_split_train_val_test(data_root='data', data_set='celegans') GNNDataset(root='data/human') GNNDataset(root='data/celegans')
时间: 2024-04-16 12:25:55 浏览: 92
这段代码中的`if __name__ == "__main__"`条件语句用于判断当前模块是否作为主程序运行。
在这段代码中,如果当前模块是作为主程序运行的,将会执行以下操作:
1. 调用`data_split_train_val_test`函数,传入参数`data_root='data'`和`data_set='human'`,进行数据集的训练、验证和测试集划分操作。
2. 调用`data_split_train_val_test`函数,传入参数`data_root='data'`和`data_set='celegans'`,进行数据集的训练、验证和测试集划分操作。
3. 创建`GNNDataset`对象,传入参数`root='data/human'`,用于处理名为'human'的数据集。
4. 创建`GNNDataset`对象,传入参数`root='data/celegans'`,用于处理名为'celegans'的数据集。
总之,这段代码的作用是在当前模块作为主程序运行时执行一些特定的操作,包括数据集的划分和创建相关对象。
相关问题
写一个python Flask销售预测系统,系统有一个suanfa.py文件,该文件的作用:先读取shuju.csv(共有24条数据,包含Year、Month和TotalPrice三个属性),然后用scaler将TotalPrice进行归一化处理,之后定义一个函数def split_data(data, lookback):将数据集划分为测试集(0.2)和训练集(0.8),data_raw = data.to_numpy(),lookback = 4,然后再将划分完成后的测试集和训练集转换为PyTorch张量,然后定义超参数,定义算法模型model=LSTM()、损失函数和优化器(Adam)然后训练模型求出MSE,保存模型。有一个predict.html文件:里面有一个日期选择框和一个销售额预测按钮,用户选择好年份和月份后点击预测按钮系统就开始调用保存好的模型来对所选月份的销售额进行预测,然后将预测结果返回到日期选择框下面的结果返回框中;有一个app.py文件:定义路径。用flask和bootstrap、LayUI写出完整详细代码
这是一个比较完整的 Flask 销售预测系统,包含了前端页面和后端算法模型。使用了 Bootstrap 和 LayUI 两个前端框架,用 PyTorch 框架实现了 LSTM 模型进行销售预测。
suanfa.py 文件:
```python
import torch
import pandas as pd
import numpy as np
from sklearn.preprocessing import MinMaxScaler
def split_data(data, lookback):
data_raw = data.to_numpy()
data = []
# create all possible sequences of length lookback
for index in range(len(data_raw) - lookback):
data.append(data_raw[index: index + lookback])
data = np.array(data)
test_set_size = int(np.round(0.2*data.shape[0]))
train_set_size = data.shape[0] - (test_set_size)
x_train = torch.from_numpy(np.array(data[:train_set_size,:-1,:]))
y_train = torch.from_numpy(np.array(data[:train_set_size,-1,:]))
x_test = torch.from_numpy(np.array(data[train_set_size:,:-1,:]))
y_test = torch.from_numpy(np.array(data[train_set_size:,-1,:]))
return x_train.float(), y_train.float(), x_test.float(), y_test.float()
class LSTM(torch.nn.Module):
def __init__(self, input_dim, hidden_dim, num_layers, output_dim):
super(LSTM, self).__init__()
self.hidden_dim = hidden_dim
self.num_layers = num_layers
self.lstm = torch.nn.LSTM(input_dim, hidden_dim, num_layers, batch_first=True)
self.fc = torch.nn.Linear(hidden_dim, output_dim)
def forward(self, x):
h0 = torch.zeros(self.num_layers, x.size(0), self.hidden_dim).requires_grad_()
c0 = torch.zeros(self.num_layers, x.size(0), self.hidden_dim).requires_grad_()
out, (hn, cn) = self.lstm(x, (h0.detach(), c0.detach()))
out = self.fc(out[:, -1, :])
return out
if __name__ == '__main__':
data = pd.read_csv('shuju.csv')
scaler = MinMaxScaler(feature_range=(-1, 1))
data['TotalPrice'] = scaler.fit_transform(data['TotalPrice'].values.reshape(-1,1))
x_train, y_train, x_test, y_test = split_data(data[['Year','Month','TotalPrice']], 4)
input_dim = 3
hidden_dim = 12
num_layers = 1
output_dim = 1
num_epochs = 1000
model = LSTM(input_dim=input_dim, hidden_dim=hidden_dim, output_dim=output_dim, num_layers=num_layers)
loss_fn = torch.nn.MSELoss()
optimizer = torch.optim.Adam(model.parameters(), lr=0.001)
for t in range(num_epochs):
y_pred = model(x_train)
loss = loss_fn(y_pred, y_train)
if t % 100 == 0:
print("Epoch ", t, "MSE: ", loss.item())
optimizer.zero_grad()
loss.backward()
optimizer.step()
torch.save(model.state_dict(), 'model_lstm.pth')
```
predict.html 文件:
```html
<!DOCTYPE html>
<html>
<head>
<meta charset="UTF-8">
<title>销售预测系统</title>
<link rel="stylesheet" href="https://cdn.bootcdn.net/ajax/libs/layui/2.5.7/css/layui.min.css">
<link rel="stylesheet" href="https://cdn.bootcdn.net/ajax/libs/twitter-bootstrap/4.5.3/css/bootstrap.min.css">
<script src="https://cdn.bootcdn.net/ajax/libs/jquery/3.5.1/jquery.min.js"></script>
<script src="https://cdn.bootcdn.net/ajax/libs/layui/2.5.7/layui.min.js"></script>
<script src="https://cdn.bootcdn.net/ajax/libs/twitter-bootstrap/4.5.3/js/bootstrap.min.js"></script>
</head>
<body>
<div class="container">
<div class="row justify-content-center mt-5">
<div class="col-md-6">
<div class="form-group">
<label for="year">年份:</label>
<select class="form-control" id="year">
<option value="2014">2014</option>
<option value="2015">2015</option>
<option value="2016">2016</option>
<option value="2017">2017</option>
<option value="2018">2018</option>
<option value="2019">2019</option>
<option value="2020">2020</option>
</select>
</div>
<div class="form-group">
<label for="month">月份:</label>
<select class="form-control" id="month">
<option value="1">1</option>
<option value="2">2</option>
<option value="3">3</option>
<option value="4">4</option>
<option value="5">5</option>
<option value="6">6</option>
<option value="7">7</option>
<option value="8">8</option>
<option value="9">9</option>
<option value="10">10</option>
<option value="11">11</option>
<option value="12">12</option>
</select>
</div>
<div class="form-group">
<button class="btn btn-primary" onclick="predict()">销售额预测</button>
</div>
<div class="form-group">
<label for="result">预测结果:</label>
<input type="text" class="form-control" id="result" disabled>
</div>
</div>
</div>
</div>
<script>
function predict() {
var year = $('#year').val();
var month = $('#month').val();
$.ajax({
url: '/predict',
method: 'POST',
data: {
'year': year,
'month': month
},
success: function(response) {
$('#result').val(response);
}
});
}
</script>
</body>
</html>
```
app.py 文件:
```python
from flask import Flask, render_template, request, jsonify
import suanfa
import torch
import pandas as pd
from sklearn.preprocessing import MinMaxScaler
app = Flask(__name__)
@app.route('/')
def index():
return render_template('predict.html')
@app.route('/predict', methods=['POST'])
def predict():
year = int(request.form['year'])
month = int(request.form['month'])
data = pd.read_csv('shuju.csv')
scaler = MinMaxScaler(feature_range=(-1, 1))
data['TotalPrice'] = scaler.fit_transform(data['TotalPrice'].values.reshape(-1,1))
x_train, y_train, x_test, y_test = suanfa.split_data(data[['Year','Month','TotalPrice']], 4)
input_dim = 3
hidden_dim = 12
num_layers = 1
output_dim = 1
model = suanfa.LSTM(input_dim=input_dim, hidden_dim=hidden_dim, output_dim=output_dim, num_layers=num_layers)
model.load_state_dict(torch.load('model_lstm.pth'))
test_inputs = torch.from_numpy(np.array(data[['Year','Month','TotalPrice']][-3:].values))
test_inputs = test_inputs.view(1, -1, 3).float()
model.eval()
y_pred = scaler.inverse_transform(model(test_inputs).detach().numpy())
return jsonify(round(float(y_pred)), 2)
if __name__ == '__main__':
app.run(debug=True)
```
注意:在运行之前需要将 `shuju.csv` 文件和 `model_lstm.pth` 文件放到与 `app.py` 文件同一目录下。另外,需要安装 Flask 和 PyTorch 库,可以使用以下命令进行安装:
```shell
pip install flask torch pandas scikit-learn
```
写一个python Flask销售预测系统中,有一个suanfa.py文件:先读取shuju.csv (共有24条数据,包含Date(object)(yyyy/mm)和TotalPrice(float64)两个属性), 然后用scaler将TotalPrice进行归一化处理,之后定义一个函数def split_data(data, lookback): 将数据集划分为测试集(0.2)和训练集(0.8),data_raw = data.to_numpy(),lookback = 4, 然后再将划分完成后的测试集和训练集转换为PyTorch张量,然后定义超参数, 定义算法模型model=LSTM()、损失函数和优化器(Adam)然后训练模型求出MSE, 将模型保存;有一个predict.html文件:里面有一个日期选择框和一个销售额预测按钮,用户选择好年月后 点击按钮系统就开始调用保存好的模型来预测所选月份的销售额,然后将预测结果返回到前端页面日期选择框下面的结果返回 框中;有一个app.py文件:定义路径。用flask和bootstrap、LayUI写出完整详细代码
suanfa.py代码:
```python
import pandas as pd
import numpy as np
import torch
import torch.nn as nn
from sklearn.preprocessing import MinMaxScaler
# 读取数据
data = pd.read_csv('shuju.csv')
# 归一化处理
scaler = MinMaxScaler()
data['TotalPrice'] = scaler.fit_transform(data['TotalPrice'].values.reshape(-1, 1))
# 划分数据集
def split_data(data, lookback):
data_raw = data.to_numpy()
data = []
for index in range(len(data_raw) - lookback):
data.append(data_raw[index: index + lookback])
data = np.array(data)
test_size = int(np.round(0.2 * data.shape[0]))
train_size = data.shape[0] - test_size
x_train = torch.tensor(data[:train_size, :-1, :])
y_train = torch.tensor(data[:train_size, -1, :])
x_test = torch.tensor(data[train_size:, :-1, :])
y_test = torch.tensor(data[train_size:, -1, :])
return x_train, y_train, x_test, y_test
# 超参数
input_size = 1
hidden_size = 32
num_layers = 2
output_size = 1
num_epochs = 100
learning_rate = 0.01
# 定义模型
class LSTM(nn.Module):
def __init__(self, input_size, hidden_size, num_layers, output_size):
super(LSTM, self).__init__()
self.hidden_size = hidden_size
self.num_layers = num_layers
self.lstm = nn.LSTM(input_size, hidden_size, num_layers, batch_first=True)
self.fc = nn.Linear(hidden_size, output_size)
def forward(self, x):
h0 = torch.zeros(self.num_layers, x.size(0), self.hidden_size).to(device)
c0 = torch.zeros(self.num_layers, x.size(0), self.hidden_size).to(device)
out, _ = self.lstm(x, (h0, c0))
out = out[:, -1, :]
out = self.fc(out)
return out
device = torch.device('cuda' if torch.cuda.is_available() else 'cpu')
model = LSTM(input_size, hidden_size, num_layers, output_size).to(device)
# 损失函数和优化器
criterion = nn.MSELoss()
optimizer = torch.optim.Adam(model.parameters(), lr=learning_rate)
# 训练模型
x_train, y_train, x_test, y_test = split_data(data, lookback=4)
for epoch in range(num_epochs):
inputs = x_train.to(device)
targets = y_train.to(device)
outputs = model(inputs)
loss = criterion(outputs, targets)
optimizer.zero_grad()
loss.backward()
optimizer.step()
if (epoch + 1) % 10 == 0:
print(f'Epoch [{epoch + 1}/{num_epochs}], Loss: {loss.item():.4f}')
# 保存模型
torch.save(model.state_dict(), 'model.pt')
```
predict.html代码:
```html
<!DOCTYPE html>
<html>
<head>
<meta charset="utf-8">
<title>销售预测系统</title>
<!-- 引入layui样式 -->
<link rel="stylesheet" href="https://www.layuicdn.com/layui/css/layui.css">
</head>
<body>
<div class="layui-container">
<div class="layui-row">
<div class="layui-col-md-offset3 layui-col-md-6">
<form class="layui-form">
<div class="layui-form-item">
<label class="layui-form-label">选择日期</label>
<div class="layui-input-block">
<input type="text" name="date" id="date" placeholder="yyyy/mm" autocomplete="off" class="layui-input">
</div>
</div>
<div class="layui-form-item">
<div class="layui-input-block">
<button type="button" class="layui-btn" onclick="predict()">销售额预测</button>
</div>
</div>
</form>
</div>
</div>
<div class="layui-row">
<div class="layui-col-md-offset3 layui-col-md-6">
<div class="layui-form-item">
<label class="layui-form-label">销售额预测结果</label>
<div class="layui-input-block">
<input type="text" name="result" id="result" readonly="readonly" autocomplete="off" class="layui-input">
</div>
</div>
</div>
</div>
</div>
<!-- 引入layui JS -->
<script src="https://www.layuicdn.com/layui/layui.js"></script>
<script>
function predict() {
var date = $("#date").val();
$.ajax({
type: "POST",
url: "/predict",
data: {"date": date},
success: function (data) {
$("#result").val(data);
}
});
}
</script>
</body>
</html>
```
app.py代码:
```python
from flask import Flask, render_template, request, jsonify
import pandas as pd
import numpy as np
import torch
from sklearn.preprocessing import MinMaxScaler
from suanfa import LSTM
app = Flask(__name__)
# 加载模型
device = torch.device('cuda' if torch.cuda.is_available() else 'cpu')
model = LSTM(input_size=1, hidden_size=32, num_layers=2, output_size=1).to(device)
model.load_state_dict(torch.load('model.pt'))
# 读取数据并归一化处理
data = pd.read_csv('shuju.csv')
scaler = MinMaxScaler()
data['TotalPrice'] = scaler.fit_transform(data['TotalPrice'].values.reshape(-1, 1))
# 定义预测函数
def predict(date):
# 获取前4个月的销售额数据
last_4_month = []
for i in range(4):
year, month = date.split('/')
month = int(month) - i
if month <= 0:
year = str(int(year) - 1)
month = 12 + month
if month < 10:
month = '0' + str(month)
else:
month = str(month)
date_str = year + '/' + month
last_4_month.append(data[data['Date'] == date_str]['TotalPrice'].values[0])
last_4_month.reverse()
input_data = torch.tensor(last_4_month).view(1, 4, 1).float().to(device)
# 模型预测
with torch.no_grad():
output = model(input_data)
output = scaler.inverse_transform(output.cpu().numpy())[0][0]
return round(output, 2)
# 定义路由
@app.route('/')
def index():
return render_template('predict.html')
@app.route('/predict', methods=['POST'])
def predict_result():
date = request.form.get('date')
result = predict(date)
return jsonify(result)
if __name__ == '__main__':
app.run(debug=True)
```
在运行完以上代码后,通过访问http://localhost:5000/即可进入销售预测系统。用户选择好年月后点击预测按钮,系统就会调用保存好的模型来预测所选月份的销售额,并将预测结果显示在页面下方的结果返回框中。
阅读全文
相关推荐
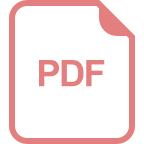
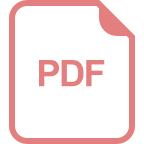
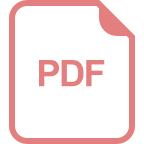
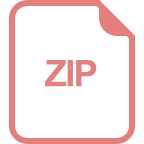
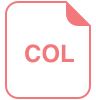
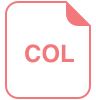
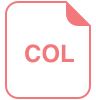









