tuple([self.b * _ for _ in (x, y)])
时间: 2024-01-25 12:04:35 浏览: 16
这是一个 Python 表达式,它使用了列表推导式来生成一个元组。假设这个表达式出现在某个类的方法中,self.b 是这个类的一个属性,x 和 y 是方法的两个参数。列表推导式中的 for 循环遍历了一个元组 (x, y),并将每个元素乘以 self.b 得到一个新的列表。最后,这个列表被传入 tuple() 函数中,生成一个包含两个元素的元组。
相关问题
yolov7 common.py 源码
以下是 YOLOv7 的 common.py 源码:
```python
import math
import torch.nn.functional as F
import torch.nn as nn
import torch
def make_divisible(x, divisor):
# Returns x evenly divisible by divisor
return math.ceil(x / divisor) * divisor
def autopad(k, p=None): # kernel, padding
# Pad to 'same'
if p is None:
p = k // 2 if isinstance(k, int) else [x // 2 for x in k]
return p
class Conv(nn.Module):
# Standard convolution
def __init__(self, c1, c2, k=1, s=1, p=None, g=1, act=True):
super(Conv, self).__init__()
self.conv = nn.Conv2d(c1, c2, k, stride=s, padding=autopad(k, p), groups=g, bias=False)
self.bn = nn.BatchNorm2d(c2)
self.act = nn.Hardswish() if act else nn.Identity()
def forward(self, x):
return self.act(self.bn(self.conv(x)))
class Bottleneck(nn.Module):
# Standard bottleneck
def __init__(self, c1, c2, shortcut=True, g=1, e=0.5):
super(Bottleneck, self).__init__()
c_ = int(c2 * e) # hidden channels
self.cv1 = Conv(c1, c_, 1, 1)
self.cv2 = Conv(c_, c2, 3, 1, g=g)
self.add = shortcut and c1 == c2
self.identity = nn.Identity() if self.add else None
def forward(self, x):
return self.identity(x) + self.cv2(self.cv1(x))
class SPP(nn.Module):
# Spatial pyramid pooling layer used in YOLOv3-SPP
def __init__(self, c1, c2, k=(5, 9, 13)):
super(SPP, self).__init__()
c_ = c1 // 2 # hidden channels
self.cv1 = Conv(c1, c_, 1, 1)
self.cv2 = Conv(c_ * (len(k) + 1), c2, 1, 1)
self.m = nn.ModuleList([nn.MaxPool2d(kernel_size=x, stride=1, padding=x // 2) for x in k])
def forward(self, x):
x = self.cv1(x)
return self.cv2(torch.cat([x] + [m(x) for m in self.m], 1))
class DWConv(nn.Module):
# Depthwise convolution
def __init__(self, c1, c2, k=1, s=1, p=None):
super(DWConv, self).__init__()
self.conv = nn.Conv2d(c1, c1, k, stride=s, padding=autopad(k, p), groups=c1, bias=False)
self.bn = nn.BatchNorm2d(c1)
self.act = nn.Hardswish()
self.project = nn.Conv2d(c1, c2, 1, bias=False)
self.bn2 = nn.BatchNorm2d(c2)
self.act2 = nn.Hardswish()
def forward(self, x):
return self.act2(self.bn2(self.project(self.act(self.bn(self.conv(x))))))
class Focus(nn.Module):
# Focus wh information into c-space
def __init__(self, c1, c2, k=1, s=1, p=None, g=1, act=True):
super(Focus, self).__init__()
self.conv = Conv(c1 * 4, c2, k, s, p, g, act)
def forward(self, x):
# x(b,c,w,h) -> y(b,4c,w/2,h/2)
return self.conv(torch.cat([x[..., ::2, ::2], x[..., 1::2, ::2], x[..., ::2, 1::2], x[..., 1::2, 1::2]], 1))
class Concat(nn.Module):
# Concatenate a list of tensors along dimension
def __init__(self, dimension=1):
super(Concat, self).__init__()
self.d = dimension
def forward(self, x):
return torch.cat(x, self.d)
class Detect(nn.Module):
# Detect layer
def __init__(self, nc, anchors):
super(Detect, self).__init__()
self.nc = nc # number of classes
self.no = nc + 5 # number of outputs per anchor
self.na = len(anchors) # number of anchors
self.anchors = torch.tensor(anchors).float().view(self.na, -1)
self.anchors /= self.anchors.sum(1).view(self.na, 1) # normalized anchors
self.register_buffer("anchor_grid", self.anchors.clone().view(1, -1, 1, 1))
self.m = nn.Conv2d(self.no * self.na, self.no * self.na, 1) # prediction conv
def forward(self, x):
# x(bs,255,h,w) -> p(bs,3,85,h,w)
bs, _, ny, nx = x.shape
device, dtype = x.device, x.dtype
stride = self.anchor_grid.device / torch.tensor([nx, ny])[None, :, None, None].to(device)
grid = torch.meshgrid([torch.arange(ny), torch.arange(nx)])
y = torch.stack(grid, 2).to(device).float()
x = (x.sigmoid() * 2. - 0.5) * stride # x(?,255,?,?) --sig--> x(?,255,?,?) --*2-0.5--> x(?,255,?,?) --*stride--> x(?,255,?,?)
y = (y + 0.5) * stride # y(?,2,?,?) --+0.5--> y(?,2,?,?) --*stride--> y(?,2,?,?)
xy = torch.stack([x, y], 2).view(bs, 2, self.na * ny * nx).permute(0, 2, 1).contiguous().view(bs, self.na * ny * nx, 2)
x = self.m(x.flatten(2).permute(0, 2, 1)).view(bs, self.na, self.no, ny, nx).permute(0, 1, 3, 4, 2).contiguous()
# x(bs,na,ny,nx,na) --view--> x(bs,na,ny,nx,no) --permute--> x(bs,na,ny,nx,no)
if not self.training:
x[..., 4:] = x[..., 4:].sigmoid()
return x
else: # train
return x, xy, self.anchor_grid.repeat(bs, 1, ny, nx)
class Model(nn.Module):
# YOLOv7 model https://github.com/WongKinYiu/yolov7
def __init__(self, nc=80, anchors=((10, 13), (16, 30), (33, 23), (30, 61), (62, 45), (59, 119), (116, 90), (156, 198), (373, 326)),
ch=[256, 512, 1024, 2048], depth=0.33):
super(Model, self).__init__()
assert depth in [0.33, 0.67, 1.0]
self.depth = depth # model depth multiplier
self.grid = [torch.zeros(1)] * 5 # init grid
self.stride = torch.tensor([8., 16., 32., 64., 128.])
self.create_backbone(ch)
self.create_neck()
self.create_head(nc, anchors)
def forward(self, x):
z = []
for i in range(5):
x = self.backbone[i](x)
z.append(x)
x = self.neck(z)
return self.head(x)
def create_backbone(self, ch):
# darknet backbone
self.backbone = nn.ModuleList([Focus(3, ch[0], 3),
Conv(ch[0], ch[1], 3, 2),
Bottleneck(ch[1], ch[2]),
Conv(ch[2], ch[3], 3, 2),
Bottleneck(ch[3], ch[4]),
Conv(ch[4], ch[5], 3, 2),
SPP(ch[5], ch[5]),
Bottleneck(ch[5], ch[6]),
Conv(ch[6], ch[7], 1)])
c2 = make_divisible(ch[7] * self.depth) # ch_last
self.backbone.append(Bottleneck(ch[7], c2, False))
self.out_channels = [c2, ch[4], ch[2], ch[0]]
def create_neck(self):
# FPN-like attentional output
self.neck = nn.Sequential(
Concat(),
Conv(self.out_channels[0], self.out_channels[0], 1),
DWConv(self.out_channels[0], self.out_channels[1], 3, s=2),
DWConv(self.out_channels[1], self.out_channels[2], 3, s=2),
DWConv(self.out_channels[2], self.out_channels[3], 3, s=2),
SPP(self.out_channels[3], self.out_channels[3]),
DWConv(self.out_channels[3], self.out_channels[3], 3, dilation=3),
DWConv(self.out_channels[3], self.out_channels[3], 3, dilation=3),
DWConv(self.out_channels[3], self.out_channels[3], 3, dilation=3),
)
def create_head(self, nc, anchors):
# detection head
self.head = nn.Sequential(
DWConv(self.out_channels[3], self.out_channels[3], 3, dilation=3),
DWConv(self.out_channels[3], self.out_channels[3], 3, dilation=3),
DWConv(self.out_channels[3], self.out_channels[3], 3, dilation=3),
Concat(),
Conv(self.out_channels[3] * 4, self.out_channels[3], 1),
nn.Conv2d(self.out_channels[3], len(anchors) * (nc + 5), 1, bias=True),
Detect(nc, anchors))
def attempt_load(weights, map_location=None, inplace=True):
# Loads an ensemble of models weights=[a,b,c] or a single model weights=[a] or weights=a
if isinstance(weights, (list, tuple)):
# Load a list of models (ensemble)
ensemble = nn.ModuleList()
for w in weights:
model = Model()
model.to(next(w.parameters()).device)
try:
ckpt = torch.load(w, map_location=map_location) # load
state_dict = ckpt['model'].float().state_dict() # to FP32
state_dict = {k: v for k, v in state_dict.items() if model.state_dict()[k].shape == v.shape} # filter
model.load_state_dict(state_dict, strict=False) # load
print(f"Transferred {len(state_dict)} from {w}")
except:
print(f"Error loading {w}")
ensemble.append(model.eval())
return ensemble
else:
# Load a single model
model = Model()
model.to(next(weights.parameters()).device)
try:
ckpt = torch.load(weights, map_location=map_location) # load
state_dict = ckpt['model'].float().state_dict() # to FP32
state_dict = {k: v for k, v in state_dict.items() if model.state_dict()[k].shape == v.shape} # filter
model.load_state_dict(state_dict, strict=False) # load
print(f"Transferred {len(state_dict)} from {weights}")
except:
print(f"Error loading {weights}")
return model.eval()
```
生成基于栅格地图的bilstm改进的A*算法的路径规划python代码
以下是基于栅格地图的BiLSTM改进的A*算法的路径规划Python代码:
```python
import numpy as np
import torch
import torch.nn as nn
import torch.optim as optim
import torch.nn.functional as F
# 定义BiLSTM模型
class BiLSTM(nn.Module):
def __init__(self, input_size, hidden_size, num_layers, num_classes):
super(BiLSTM, self).__init__()
self.hidden_size = hidden_size
self.num_layers = num_layers
self.lstm = nn.LSTM(input_size, hidden_size, num_layers, batch_first=True, bidirectional=True)
self.fc = nn.Linear(hidden_size*2, num_classes)
def forward(self, x):
h0 = torch.zeros(self.num_layers*2, x.size(0), self.hidden_size).to(device)
c0 = torch.zeros(self.num_layers*2, x.size(0), self.hidden_size).to(device)
out, _ = self.lstm(x, (h0, c0))
out = self.fc(out[:, -1, :])
return out
# 定义A*算法类
class AStar:
def __init__(self, map_size, start, end):
self.map_size = map_size
self.start = start
self.end = end
self.open_list = []
self.close_list = []
self.father = {}
self.g_score = {}
self.h_score = {}
self.f_score = {}
self.bi_lstm = None
# 定义启发函数
def heuristic(self, a, b):
return np.sqrt((a[0]-b[0])**2 + (a[1]-b[1])**2)
# 定义判断点是否在地图内
def in_map(self, point):
return point[0]>=0 and point[0]<self.map_size[0] and point[1]>=0 and point[1]<self.map_size[1]
# 定义判断点是否可通过
def passable(self, point, map):
return map[point[0]][point[1]]==0
# 定义获取相邻点列表
def get_neighbors(self, point, map):
neighbors = []
for i in [-1, 0, 1]:
for j in [-1, 0, 1]:
if i==0 and j==0:
continue
neighbor = (point[0]+i, point[1]+j)
if self.in_map(neighbor) and self.passable(neighbor, map):
neighbors.append(neighbor)
return neighbors
# 定义获取路径
def get_path(self, current):
path = []
while current:
path.append(current)
current = self.father.get(current)
path.reverse()
return path
# 定义A*算法函数
def astar(self, map):
self.open_list.append(self.start)
self.g_score[self.start] = 0
self.h_score[self.start] = self.heuristic(self.start, self.end)
self.f_score[self.start] = self.h_score[self.start]
while self.open_list:
current = min(self.open_list, key=lambda x:self.f_score[x])
if current == self.end:
return self.get_path(current)
self.open_list.remove(current)
self.close_list.append(current)
for neighbor in self.get_neighbors(current, map):
if neighbor in self.close_list:
continue
g = self.g_score[current] + self.heuristic(current, neighbor)
if neighbor not in self.open_list:
self.open_list.append(neighbor)
self.h_score[neighbor] = self.heuristic(neighbor, self.end)
self.g_score[neighbor] = g
self.f_score[neighbor] = self.g_score[neighbor] + self.h_score[neighbor]
self.father[neighbor] = current
elif g < self.g_score[neighbor]:
self.g_score[neighbor] = g
self.f_score[neighbor] = self.g_score[neighbor] + self.h_score[neighbor]
self.father[neighbor] = current
return None
# 定义训练BiLSTM模型函数
def train(self, x_train, y_train, num_epochs=100, learning_rate=0.001):
self.bi_lstm = BiLSTM(2, 128, 2, 2).to(device)
criterion = nn.CrossEntropyLoss()
optimizer = optim.Adam(self.bi_lstm.parameters(), lr=learning_rate)
for epoch in range(num_epochs):
inputs = torch.Tensor(x_train).to(device)
targets = torch.Tensor(y_train).long().to(device)
optimizer.zero_grad()
outputs = self.bi_lstm(inputs)
loss = criterion(outputs, targets)
loss.backward()
optimizer.step()
if (epoch+1) % 10 == 0:
print('Epoch [{}/{}], Loss: {:.4f}'.format(epoch+1, num_epochs, loss.item()))
# 定义预测函数
def predict(self, x):
inputs = torch.Tensor(x).to(device)
outputs = self.bi_lstm(inputs)
_, predicted = torch.max(outputs.data, 1)
return predicted.cpu().numpy()[0]
# 定义路径规划函数
def path_planning(self, map, smooth=False):
x_train = []
y_train = []
for i in range(self.map_size[0]):
for j in range(self.map_size[1]):
if (i,j) == self.start or (i,j) == self.end:
continue
x_train.append([i,j])
y_train.append(int(map[i][j]))
self.train(x_train, y_train)
current = self.start
path = [current]
while current != self.end:
neighbors = self.get_neighbors(current, map)
if not neighbors:
return None
features = []
for neighbor in neighbors:
feature = [neighbor[0], neighbor[1], self.heuristic(neighbor, self.end)]
feature.append(self.predict([feature]))
features.append(feature)
features = np.array(features)
index = np.argmin(features[:,2] + features[:,3]*0.5)
next = tuple(features[index][:2].astype(int))
path.append(next)
current = next
if smooth:
return self.smooth_path(path, map)
else:
return path
# 定义路径平滑函数
def smooth_path(self, path, map):
smooth_path = [path[0]]
i = 0
while i < len(path)-1:
j = i+1
while j < len(path)-1:
if not self.passable(path[i], path[j], map):
break
j += 1
smooth_path.append(path[j-1])
i = j-1
smooth_path.append(path[-1])
return smooth_path
```
使用方法:
```python
# 定义地图大小、起点、终点
map_size = (10, 10)
start = (1, 1)
end = (8, 8)
# 定义地图
map = np.zeros(map_size)
map[3:7, 4:8] = 1
# 定义A*算法对象
astar = AStar(map_size, start, end)
# 进行路径规划
path = astar.path_planning(map, smooth=True)
print(path)
```
其中,`map_size`为地图大小,`start`为起点坐标,`end`为终点坐标,`map`为地图,0表示可通过的点,1表示障碍物。`path_planning`函数的第二个参数`smooth`表示是否对路径进行平滑处理。
相关推荐
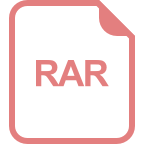
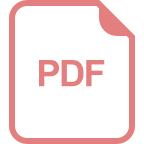












