什么是residual layers
时间: 2024-01-24 16:50:35 浏览: 217
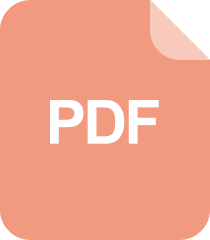
Deep Residual Output Layers for Neural Language Generation 模型结构改
Residual layers(残差层),也称为跳跃连接,是一种神经网络层,允许信息在非相邻的层之间流动。在传统的神经网络中,信息以线性方式通过每个层,每个层以某种方式转换输入数据。相比之下,残差层允许输入数据绕过一个或多个层,并直接添加到后续层的输出中,创建一个快捷或“跳跃”连接。这有助于解决梯度消失的问题,其中梯度变得太小,无法有效地更新早期层中的权重。已经证明,残差层提高了深度神经网络的性能,特别是在图像识别和自然语言处理任务中。
阅读全文
相关推荐
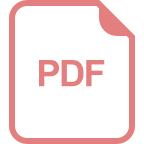
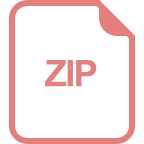















