class Net(torch.nn.Module): def __init__(self,dim_in,dim_out,class_number): super(Net,self).__init__() ''' 2. dim_in,dim_out,或者加层数 ''' self.layer1=torch.nn.Linear(dim_in,dim_out) # 权重矩阵 引入线性映射 self.layer2=torch.nn.Linear(dim_out,class_number) def forward(self,x): ''' 激活函数 ''' x=F.relu(self.layer1(x)) # 非线性激活函数 y=self.layer2(x) return y
时间: 2024-04-18 12:27:29 浏览: 20
你的代码定义了一个名为`Net`的类,它是`torch.nn.Module`类的子类。这个类表示了一个神经网络模型,并且具有两个线性层。
在`__init__`方法中,你接受了三个参数:`dim_in`、`dim_out`和`class_number`,分别表示输入维度、输出维度和类别数量。然后,你调用了父类`torch.nn.Module`的构造函数来初始化网络。接下来,你定义了两个线性层,并将它们保存在成员变量`layer1`和`layer2`中。
在`forward`方法中,你实现了网络的前向传播过程。首先,输入张量`x`通过第一个线性层`layer1`进行线性映射,并通过ReLU激活函数进行非线性转换。然后,得到的结果再通过第二个线性层`layer2`进行线性映射,得到最终的输出张量`y`。
这样,你定义的神经网络模型就完成了。
如果你有其他问题,请随时提问!我会尽力帮助你。
相关问题
net=Net(dim_in=1,dim_out=10,class_number=1)
这行代码是创建一个名为 "net" 的神经网络模型,其中包含了一个输入维度为 1、输出维度为 10 的线性层,并且设置类别数量为 1。
根据提供的代码,可以推测 `Net` 是一个自定义的神经网络类,它可能如下所示:
```python
import torch.nn as nn
class Net(nn.Module):
def __init__(self, dim_in, dim_out, class_number):
super(Net, self).__init__()
self.layer = nn.Linear(dim_in, dim_out)
self.classifier = nn.Linear(dim_out, class_number)
def forward(self, x):
x = self.layer(x)
x = self.classifier(x)
return x
```
在这个自定义的神经网络类中,我们首先定义了两个线性层:`self.layer` 和 `self.classifier`。`self.layer` 的输入维度是 `dim_in`,输出维度是 `dim_out`,它用于对输入进行一次线性变换。`self.classifier` 的输入维度是 `dim_out`,输出维度是 `class_number`,它用于将特征映射到类别空间。
在 forward 方法中,我们对输入数据 x 进行前向传播。首先将 x 通过 `self.layer` 进行线性变换,然后再通过 `self.classifier` 进行线性变换,并将结果返回。这样就完成了整个神经网络的前向计算过程。
请注意,以上只是一个推测的自定义神经网络类的代码示例,实际情况可能有所不同。
class C3HB(nn.Module): # CSP HorBlock with 3 convolutions by iscyy/yoloair def __init__(self, c1, c2, n=1, shortcut=True, g=1, e=0.5): # ch_in, ch_out, number, shortcut, groups, expansion super().__init__() c_ = int(c2 * e) # hidden channels self.cv1 = Conv(c1, c_, 1, 1) self.cv2 = Conv(c1, c_, 1, 1) self.cv3 = Conv(2 * c_, c2, 1) self.m = nn.Sequential(*(HorBlock(c_) for _ in range(n))) def forward(self, x): return self.cv3(torch.cat((self.m(self.cv1(x)), self.cv2(x)), dim=1))
这段代码定义了一个名为C3HB的PyTorch神经网络模型类,实现了CSP (cross stage partial) HorBlock,即跨阶段局部连接水平块。该模型包含了3个卷积层和1个Sequential模块,其中Sequential模块由n个HorBlock组成。HorBlock是一个自定义的卷积块,由多个卷积层和残差连接构成。
在该模型的初始化函数中,定义了输入通道数c1,输出通道数c2,以及一些可选的参数,如卷积层数量n,是否使用快捷连接shortcut,组数g和扩展率e等。在初始化函数中,定义了3个卷积层,分别为cv1、cv2和cv3。其中cv1和cv2的输入通道数均为c1,输出通道数为c_,即隐藏通道数;cv3的输入通道数为2*c_,输出通道数为c2。同时,定义了一个Sequential模块m,由n个HorBlock组成。
在前向传播函数forward中,首先对输入x分别进行cv1和cv2的卷积操作,然后将cv1(x)和m(cv1(x))进行拼接,再与cv2(x)进行拼接,最后将两个拼接结果进行拼接后,输入到cv3中进行卷积操作,得到最终的输出结果。
相关推荐
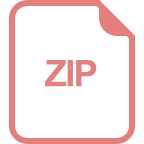












