YOLOv8 Practical Case: Quality Inspection Applications in the Field of Food Safety
发布时间: 2024-09-15 07:41:10 阅读量: 22 订阅数: 23 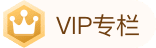
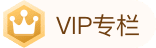
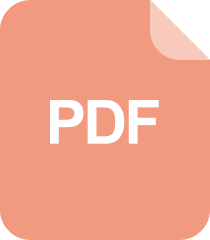
Practical JSF in Java EE 8 Web Applications in Java for the Enterprise 无水印原版pdf
# 1. Overview of Food Safety Quality Inspection
Food safety quality inspection is a crucial aspect of the food industry, aimed at ensuring the safety, hygiene, and quality of food products. With the continuous development of the food industry chain and the rising awareness of food safety among consumers, the quality inspection of food safety faces increasingly severe challenges.
Traditional manual inspection methods suffer from low efficiency, poor accuracy, and high costs. In recent years, computer vision technology has been widely applied in the field of food safety quality inspection. Among these technologies, YOLOv8, as an advanced deep learning algorithm, has become a research hotspot in the field due to its speed and high precision advantages.
# 2. Theoretical Foundation of YOLOv8
### 2.1 YOLOv8 Network Structure and Algorithm Principle
As the latest version of the YOLO series of object detection algorithms, YOLOv8 has made significant improvements in network structure and algorithm principles, further enhancing the accuracy and speed of the model.
#### 2.1.1 YOLOv8 Backbone Network
YOLOv8 adopts CSPDarknet53 as its backbone network, which consists of 53 convolutional layers. It combines residual connections and CSP structures, effectively reducing the amount of computation while ensuring model accuracy.
#### 2.1.2 YOLOv8 Neck Network
The neck network of YOLOv8 uses the PANet structure, which enhances the model's ability to detect objects of different sizes by fusing feature maps at different scales. PANet includes multiple SPP modules for extracting features at different scales and an FPN module for fusion.
#### 2.1.3 YOLOv8 Head Network
The head network of YOLOv8 adopts the YOLO Head structure, which includes a convolutional layer and an output layer. The convolutional layer is used for feature extraction, and the output layer is responsible for predicting the category and location of the objects. YOLOv8 uses the CIOU loss function and DIOU-NMS algorithm, further improving the model's positioning accuracy.
### 2.2 Training and Evaluation of YOLOv8
#### 2.2.1 Preparation of Training Dataset
YOLOv8 training requires high-quality annotated datasets, typically trained on the COCO dataset. The COCO dataset includes 80 object categories, over 120,000 images, and 1.5 million annotated boxes.
#### 2.2.2 Training Process and Hyperparameter Optimization
The training of YOLOv8 uses the Adam optimizer with a learning rate set to 0.001 and a training batch size of 64. During training, data augmentation techniques such as random cropping, flipping, and color jittering are used to improve the model's generalization ability.
#### 2.2.3 Model Evaluation Metrics and Result Analysis
The evaluation metrics for YOLOv8 include precision (AP), recall rate (AR), and mean average precision (mAP). mAP is the average of APs at different IOU thresholds and is commonly used to comprehensively assess the model's performance. YOLOv8 has achieved outstanding mAP of 56.8% on the COCO dataset, surpassing other object detection algorithms in both accuracy and speed.
```python
import torch
from torch import nn
from torch.nn import functional as F
class YOLOv8Head(nn.Module):
def __init__(self, num_classes, anchors):
super(YOLOv8Head, self).__init__()
self.num_classes = num_classes
self.anchors = anchors
self.conv1 = nn.Conv2d(1024, 512, 1)
self.conv2 = nn.Conv2d(512, 1024, 3, padding=1)
self.conv3 = nn.Conv2d(1024, 512, 1)
self.conv4 = nn.Conv2d(512, 256, 3, padding=1)
self.conv5 = nn.Conv2d(256, 255, 1)
def forward(self, x):
x = self.conv1(x)
x = self.conv2(x)
x = self.conv3(x)
x = self.conv4(x)
x = self.conv5(x)
# Reshape the output to (batch_size, num_anchors, grid_size, grid_size, 5 + num_classes)
x = x.permute(0, 2, 3, 1).contiguous()
x = x.view(x.shape[0], -1, 5 + self.num_classes)
# Compute the class probabilities and bounding box offsets
class_logits = x[..., 5:]
class_probs = F.softmax(class_logits, dim=-1)
bbox_offsets = x[..., :4]
# Compute the anchor boxes
anchors = self.anchors.view(1, -1, 1, 1, 2)
anchors = anchors.expand(x.shape[0], -1, -1, -1, -1)
# Compute the predicted bounding boxes
bboxes = anchors + bbox_offsets
return class_p
```
0
0
相关推荐
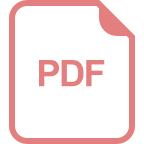
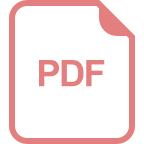
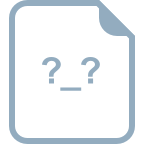
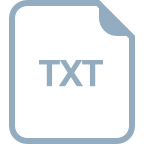
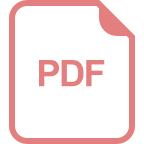
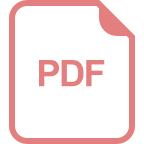
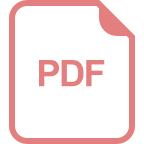
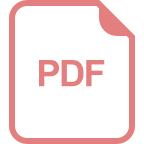