YOLOv8 Practical Case: Intelligent Robot Visual Navigation and Obstacle Avoidance
发布时间: 2024-09-15 07:52:42 阅读量: 8 订阅数: 22 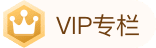
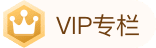
# Section 1: Overview and Principles of YOLOv8
YOLOv8 is the latest version of the You Only Look Once (YOLO) object detection algorithm, ***pared to previous versions of YOLO, YOLOv8 has seen significant improvements in accuracy and speed.
YOLOv8 employs a new network architecture known as Cross-Stage Partial Connections (CSP). The CSP architecture reduces the amount of computation required by introducing skip connections at different stages of the network while maintaining the accuracy of the model. Additionally, YOLOv8 utilizes the Path Aggregation Network (PAN) module, which enhances the model's feature extraction capabilities by fusing feature maps from different stages.
In terms of training, YOLOv8 adopts self-supervised learning techniques, meaning the model is trained without human annotations. This allows the model to learn from vast amounts of unlabeled data, thereby improving the model's generalization capabilities.
# Section 2: Intelligent Robot Vision Navigation
### 2.1 Theoretical Foundations of Robot Vision Navigation
#### 2.1.1 SLAM and Visual Odometry
**Simultaneous Localization and Mapping (SLAM)**: SLAM is a robotic technique used for building environmental maps and estimating the robot's position simultaneously. It is achieved by fusing data from sensors such as LiDAR, cameras.
**Visual Odometry**: Visual odometry is a form of SLAM technology that uses only camera data to estimate a robot's motion and position. It is implemented by tracking feature points in a sequence of images.
#### 2.1.2 Applications of Deep Learning in Vision Navigation
Deep learning models, such as YOLOv8, have been widely applied to robot vision navigation. They can perform real-time object detection, which is crucial for environmental perception and path planning.
### 2.2 YOLOv8 in Practice for Robot Vision Navigation
#### 2.2.1 Model Training and Deployment
**Model Training:**
- Gather an image dataset with labeled objects.
- Train the YOLOv8 model to detect specific categories (e.g., pedestrians, vehicles).
- Optimize model parameters to improve accuracy and speed.
**Model Deployment:**
- Deploy the trained model onto the robot platform.
- Optimize model inference time to meet real-time navigation requirements.
#### 2.2.2 Real-Time Object Detection and Navigation Decisions
**Real-Time Object Detection:**
- Use the YOLOv8 model to detect objects in the environment in real time.
- Extract information such as object location, category, and confidence.
**Navigation Decisions:**
- Based on detected objects, the robot makes navigation decisions.
- Avoid obstacles, follow paths, or navigate to specified locations.
#### 2.2.3 Obstacle Avoidance and Path Planning
**Obstacle Avoidance:**
- Use YOLOv8 to detect obstacles and estimate their positions.
- Generate obstacle avoidance paths based on this information.
**Path Planning:**
- Plan a safe path using detected objects and obstacles.
- Consider path length, obstacle distance, and navigation objectives.
### 2.2.4 Obstacle Avoidance Algorithm Optimization and Evaluation
**Algorithm Optimization:**
- Adjust algorithm parameters to improve obstacle avoidance efficiency.
- Consider robot dynamics and environmental complexity.
**Algorithm Evaluation:**
- Evaluate the performance of the obstacle avoidance algorithm using simulations or real-world scenarios.
- Measure success rates, path lengths, and navigation times.
### Code Example: YOLOv8 Real-Time Object Detection
```python
import cv2
import numpy as np
# Load YOLOv8 model
net = cv2.dnn.readNet("yolov8.weights", "yolov8.cfg")
# Read image
image = cv2.imread("image.jpg")
# Preprocess image
blob = cv2.dnn.blobFromImage(image, 1 / 255.0, (416, 416), (0, 0, 0), swapRB=True, crop=False)
# Set input
net.setInput(blob)
# Forward propagation
detections = net.forward()
# Parse detection results
for detection in detections[0, 0]:
confidence = detection[2]
if confidence > 0.5:
x, y, w, h = detection[3:7] * np.array([image.shape[1], image.shape[0], image.shape[1], image.shape[0]])
cv2.rectangle(image, (x - w / 2, y - h / 2), (x + w / 2, y + h / 2), (0, 255, 0), 2)
# Display detection results
cv2.imshow("Image", image)
cv2.waitKey(0)
```
**Code Logic Analysis:**
- Load the YOLOv8 model an
0
0
相关推荐
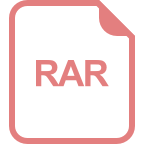
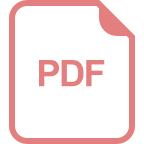
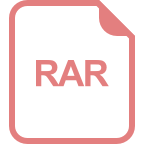





