YOLOv8 Model Acceleration Optimization Methods on GPU
发布时间: 2024-09-15 07:18:21 阅读量: 55 订阅数: 25 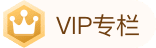
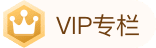
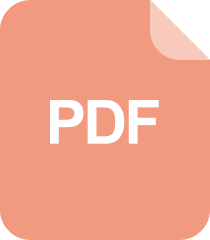
Image Blending Techniques Based on GPU Acceleration.pdf
# Introduction to the YOLOv8 Model and Acceleration Optimization Methods on GPU
## 1. Introduction to the YOLOv8 Model
YOLOv8, the latest version of the You Only Look Once (YOLO) object detection algorithm, was released by Megvii Technology in 2022. It is renowned for its exceptional accuracy and speed, achieving an AP (Average Precision) of 56.8% and an FPS (Frames Per Second) of 160 on the COCO dataset.
YOLOv8 employs a variety of innovative technologies, including:
***Bag of Freebies (BoF):** A set of free training tricks that significantly enhance model accuracy without increasing training time or computational costs.
***Cross-Stage Partial Connections (CSP):** A novel network architecture that reduces computation while maintaining model accuracy.
***Path Aggregation Network (PAN):** A feature aggregation module that improves the detection accuracy of small objects.
## 2. Theoretical Foundation for YOLOv8 Model Acceleration Optimization
### 2.1 Model Compression and Pruning
Model compression and pruning are two techniques used to accelerate model inference by reducing the size and complexity of the model.
#### 2.1.1 Model Quantization
Model quantization is the process of converting the floating-point weights and activations in the model to low-precision formats (such as int8 or int16). This can significantly reduce the size and memory footprint of the model, thereby increasing inference speed.
**Code Block:**
```python
import torch
from torch.quantization import quantize_dynamic
# Create a floating-point model
model = torch.nn.Linear(10, 10)
# Quantize the model to int8
quantized_model = quantize_dynamic(model, qconfig_spec={torch.nn.Linear: torch.quantization.default_qconfig})
```
**Logical Analysis:**
* The `quantize_dynamic` function quantizes the model to int8 format.
* `qconfig_spec` specifies the quantization configuration, where `torch.nn.Linear` indicates that linear layers should use the default quantization configuration.
#### 2.1.2 Model Distillation
Model distillation is a technique that transfers knowledge from a large "teacher" model to a smaller "student" model. This can create a student model with similar performance to the teacher model but with a smaller size and complexity.
**Code Block:**
```python
import torch
from torch.nn.utils import distill
# Create teacher and student models
teacher_model = torch.nn.Linear(10, 10)
student_model = torch.nn.Linear(5, 10)
# Distill the teacher model into the student model
distill.kl_divergence(student_model, teacher_model)
```
**Logical Analysis:**
* The `kl_divergence` function calculates the KL divergence between the teacher model and the student model and uses it as a loss function to train the student model.
* By minimizing the KL divergence, the student model learns to imitate the output distribution of the teacher model.
### 2.2 Parallel Computing and Distributed Training
Parallel computing and distributed training accelerate model training and inference by utilizing multiple computing devices, such as GPUs or TPUs.
#### 2.2.1 Data Parallelism
Data parallelism is a technique that divides the training data into multiple small batches and processes these batches in parallel on different devices. This can significantly increase training speed.
**Code Block:**
```python
import torch
import torch.nn.parallel
# Create a data parallel model
model = torch.nn.DataParallel(torch.nn.Linear(10, 10))
# Train the model in parallel
model.train()
for batch in data_loader:
model(batch)
```
**Logical Analysis:**
* `torch.nn.DataParallel` wraps the model into a data parallel model.
* During training, each device receives a small batch of data and computes the loss and updates the model weights in parallel.
#### 2.2.2 Model Parallelism
Model parallelism is a technique that divides the model into multiple smaller parts and processes these parts in parallel on different devices. This is useful for large models that cannot fit into the memory of a single device.
**Code Block:**
```python
import torch
from torch.distributed import distributed_c10d
# Create a model parallel model
model = torch.nn.parallel.DistributedDataParallel(torch.nn.Linear(10, 10))
# Train the model in parallel
model.train()
for batch in data_loader:
model(batch)
```
**Logical Analysis:**
* `torch.nn.parallel.DistributedDataParallel` wraps the model into a model parallel model.
* During training, each device receives a part of the model and computes the loss and updates the model weights in parallel.
#### 2.2.3 Distributed Training Frameworks
Distributed training frameworks provide tools and APIs for managing the distributed training process. These frameworks include:
***Horovod:** A high-performance library for distributed training on multiple GPUs.
***PyTorch-Lightning:** A high-level framework for building and training deep learning models that supports distributed training.
**Table: Comparison of Distributed Training Frameworks**
| Feature | Horovod | PyTorch-Lightning |
|---|---|---|
| Supported Devices | GPU | GPU, TPU |
| API | C++, Python | Python |
| Ease of Use | Lower | Higher |
## 3.1 PyTorch and CUDA Programming
### 3.1.1 PyTorch Basics
PyTorch is a popular deep learning framework that provides a flexible and easy-to-use API for building and training neural networks. PyTorch uses tensors (multi-dimensional arrays) as its fundamental data structure and supports dynamic computation graphs, allowing modifications to the
0
0
相关推荐







