YOLOv8 Practical Case: Crop Pest and Disease Detection in Smart Agriculture
发布时间: 2024-09-15 07:42:49 阅读量: 20 订阅数: 21 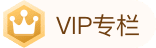
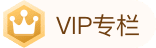
# 1. YOLOv8 Overview
YOLOv8 represents a groundbreaking advancement in the field of object detection, elevating both the accuracy and speed to new heights. YOLOv8 employs an innovative neural network architecture that combines the strengths of Convolutional Neural Networks (CNN) ***pared to previous versions of YOLO, YOLOv8 shows significant improvements in both accuracy and speed.
In YOLOv8, CNNs are utilized for image feature extraction, while Transformers handle sequence data. This combination enables YOLOv8 to capture both local and global features of images. Furthermore, YOLOv8 introduces new training strategies, such as self-supervised learning and knowledge distillation, further enhancing the model's performance.
# 2. Crop Pest and Disease Detection
### 2.1 Problem Definition and Data Preparation
#### 2.1.1 Common Types of Crop Pests and Diseases
There is a wide variety of crop pests and diseases, ***mon types include:
- **Diseases:** Caused by pathogens such as fungi, bacteria, and viruses, examples include powdery mildew, rust, and leaf spot.
- **Pests:** Caused by insects, mites, nematodes, etc., examples include aphids, spider mites, and corn borers.
- **Weeds:** Plants that compete with crops for nutrients, water, and sunlight, examples include barnyardgrass, foxtail, and crabgrass.
#### 2.1.2 Dataset Collection and Preprocessing
Training a crop pest and disease detection model requires a substantial amount of high-quality data. Dataset collection involves:
- **Image Acquisition:** Capturing crop images using cameras or drones at different growth stages and under various environmental conditions.
- **Annotation:** Labeling pests and diseases in the images, including location, type, and severity.
Data preprocessing includes:
- **Image Preprocessing:** Adjusting image size, format, and enhancing contrast.
- **Data Augmentation:** Techniques such as rotation, flipping, and cropping to increase dataset diversity.
- **Data Splitting:** Dividing the dataset into training, validation, and test sets.
### 2.2 Model Training and Evaluation
#### 2.2.1 YOLOv8 Model Structure and Training Parameters
YOLOv8 adopts the improved CSPDarknet53 backbone network, featuring:
- **CSP Structure:** Reduces computational load and improves feature extraction efficiency through cross-stage partial connections.
- **Mish Activation Function:** A non-monotonic activation function that enhances model convergence and robustness.
- **PAN Path Aggregation Network:** Fuses feature maps of different scales, enhancing the accuracy and robustness of object detection.
Training parameters include:
- **Batch Size:** The number of images in each training batch, affecting training speed and memory consumption.
- **Learning Rate:** The step size used by the optimizer to update model parameters, impacting model convergence speed and final performance.
- **Weight Decay:** Prevents overfitting by penalizing large weight values to regularize the model.
#### 2.2.2 Training Process and Evaluation Metrics
The model training is an iterative process, consisting of:
- **Forward Propagation:** Inputting images and predicting target locations and categories through the model.
- **Backward Propagation:** Calculating the loss function between predictions and true labels, and backpropagating errors to update model parameters.
Evaluation metrics include:
- **Mean Average Precision (mAP):** Measures the accuracy and recall of the model in detecting different categories of objects.
- **Frames Per Second (FPS):** Measures the real-time detection speed of the model.
- **Loss Function:** Measures the difference between model predictions and true labels, such as cross-entropy loss or IoU loss.
### 2.3 Model Deployment and Application
#### 2.3.1 Model Optimization and Deployment Methods
Model optimization can reduce model size and computational requirements, facilitating deployment. Optimization methods include:
- **Quantization:** Converting floating-point weights and activations to lower precision integers.
- **Pruning:** Removing unimportant network connections and weights.
- **Distillation:** Transferring knowledge from a large teacher model to a smaller student model.
Deployment methods include:
- **Local Deployment:** Deploying the model on edge devices or servers for real-time detection.
- **Cloud Deployment:** Deploying the model on cloud platforms for scalability and high performance.
#### 2.3.2 Real-time Detection and Result Presentation
Once deployed, the model can perform real-time detection:
- **Image Input:** Input the image to be detected.
- **Model Inference:** The model predicts target locations and categories.
- **Result Presentation:** Draws detection boxes and labels on the image.
Detection results can be presented in the following ways:
- **Visualization Interface:** Display detection results on Web or mobile applications.
- **API Interface:** Provides RESTful API interfaces for easy integration with other systems.
- **File Output:** Exports detection results as CSV or JSON files.
# 3.1 Convolutional Neural Networks (CNN)
#### 3.1.1 Basic Principles and Architecture of CNN
A Convolutional Neural Network (CNN) is a deep learning model designed specifically for processing grid-like data, such as images and videos. The core concept of CNNs is the use of convolutional operations to extract local features from the data.
0
0
相关推荐
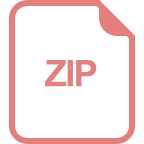
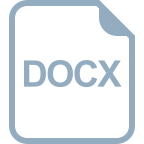
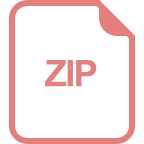
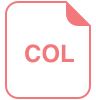
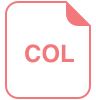
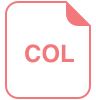
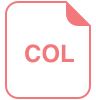
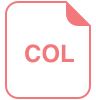
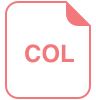