YOLOv8 Practical Cases: Behavior Detection and Recognition in Smart Homes
发布时间: 2024-09-15 07:49:23 阅读量: 20 订阅数: 48 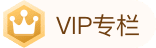
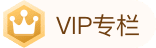
# YOLOv8 Practical Cases: Behavior Detection and Recognition in Smart Homes
## 2.1 Concepts and Methods of Behavior Detection and Recognition
### 2.1.1 Principles and Algorithms of Behavior Detection
Behavior detection refers to the identification and localization of human actions in video or image sequences. It typically involves the following steps:
***Action Detection:** Identifying moving areas in video or image sequences.
***Action Tracking:** Tracking moving areas through consecutive frames to establish motion trajectories.
***Behavior Classification:***
***mon behavior detection algorithms include:
***Optical Flow Method:** Computes pixel movements between consecutive frames to recognize moving areas.
***Background Subtraction Method:** Compares the current frame with a background model to detect moving areas.
***Deep Learning Method:** Uses Convolutional Neural Networks (CNN) to extract features from video or image sequences and classify behaviors.
### 2.1.2 Feature Extraction and Classification for Behavior Recognition
Behavior recognition is the process of further identifying the type or category of behavior. It involves extracting features from motion trajectories and body postures, which are then fed into a classifier for categorization.
Feature extraction techniques include:
***Spatial Features:** Body posture, shape, and size of motion trajectories.
***Temporal Features:** Duration, speed, and acceleration of actions.
***Skeletal Features:** Positions and angles of human joints.
Classifier algorithms include:
***Support Vector Machine (SVM):** A binary classification algorithm that can be used to categorize behaviors into different classes.
***Random Forest:** A decision tree algorithm suitable for multiclass classification problems.
***Deep Learning Method:** Using CNNs to learn behavioral patterns from features and classify them.
## 2. Theoretical Basis for Behavior Detection and Recognition in Smart Homes
### 2.1 Concepts and Methods of Behavior Detection and Recognition
#### 2.1.1 Principles and Algorithms of Behavior Detection
Behavior detection aims to identify human behaviors in video sequences. Its principle involves analyzing video frames with computer vision algorithms, extracting features, ***mon behavior detection algorithms include:
- **Optical Flow Method:** Captures motion information by computing pixel movements between consecutive frames to recognize behaviors.
- **Background Modeling Method:** Establishes a video background model to detect moving objects that differ from the background and identify their behaviors.
- **Action Recognition Method:** Utilizes deep learning models to extract action features from video frames and classify them into specific behaviors.
#### 2.1.2 Feature Extraction and Classification for Behavior Recogni***
***mon feature extraction methods include:
- **Spatiotemporal Features:** Utilizing techniques such as optical flow to extract features of motion and spatiotemporal changes in video frames.
- **Local Features:** Extracting features from local areas in video frames, such as HOG, LBP, etc.
- **Deep Features:** Using Convolutional Neural Networks (CNN) to extract high-level semantic features from video frames.
After feature extraction, ***mon classification methods include:
- **Support Vector Machine (SVM):** A supervised learning algorithm that learns decision boundaries through training data to classify features into different behaviors.
- **Decision Tree:** A tree-structured classifier that recursively divides the feature space to classify features into different behaviors.
- **Neural Network:** A multilayer perceptron that learns nonlinear relationships between features to classify them into different behaviors.
### 2.2 Behavior Analysis in Smart Home Environments
#### 2.2.1 Types and Characteristics of Common Behaviors
Common behavior types in smart home environments include:
- **Daily Activities:** Such as walking, sitting down, eating, etc.
- **Household Chores:** Such as cooking, cleaning, etc.
- **Leisure Activities:** Such as watching TV, playing games, etc.
- **Abnormal Behaviors:** Such as falling, intruding, etc.
Different behaviors have unique characteristics, such as motion patterns, duration, and participants involved.
#### 2.2.2 Challenges and Difficulties in Behavior Analysis
Behavior analysis in smart home environments faces the following challenges and difficulties:
- **Complex Backgrounds:** Smart home environments usually contain complex and variable backgrounds, which can interfere with behavior detection and recognition.
- **Diversity of Behaviors:** Human behaviors are diverse and changeable, making it difficult to enumerate all behavior patterns.
- **Occlusion and Viewpoint Issues:** Object occlusion and different viewpoints can affect the extraction and recognition of behavior features.
- **Real-time Requirements:** Smart home behavior analysis requires real-time responses to achieve timely alerts and control.
## 3.1 Training and Evaluation of the YOLOv8 Model
### 3.1.1 Preparatio***
***monly used datasets for smart home behavior detection and recognition include:
| Dataset | Description |
|---|---|
| CHARADES | 157,041 video clips, including 171 action categories |
| ActivityNet | 20,000 video clips, including 100 acti
0
0
相关推荐
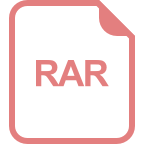
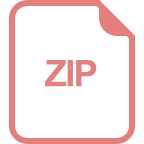
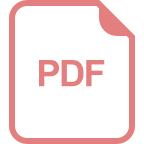
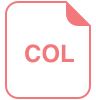
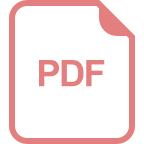
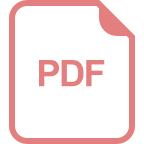
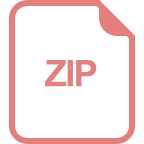
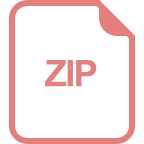
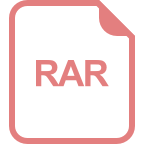