YOLOv8 Practical Case: Road Traffic Vehicle Detection and Counting
发布时间: 2024-09-15 07:36:44 阅读量: 4 订阅数: 22 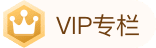
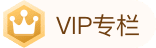
#YOLOv8 Real-world Case Study: Road Traffic Vehicle Detection and Counting
## 1.1 Introduction to YOLOv8 Model
YOLOv8 is one of the most advanced real-time object detection models, ***pared to its predecessor YOLOv7, YOLOv8 has significantly improved in accuracy and speed. YOLOv8 adopts a new network architecture known as Cross-Stage Partial Connections (CSP), which reduces the amount of computation while maintaining the model's accuracy. Additionally, YOLOv8 employs a new training strategy called SimOTA, which enhances the model's performance in detecting small objects.
## 2. Setting Up the YOLOv8 Practical Environment and Data Preparation
### 2.1 Environment Setup and Dependency Installation
**Environment Requirements:**
* Operating System: Ubuntu 18.04 or higher versions
* Python: 3.7 or higher versions
* CUDA: 11.1 or higher versions
* cuDNN: 8.0 or higher versions
* PyTorch: 1.8 or higher versions
**Dependency Installation:**
1. Install CUDA and cuDNN, referring to the official documentation for specific steps.
2. Create a virtual environment and install PyTorch:
```bash
python3 -m venv venv
source venv/bin/activate
pip install torch torchvision
```
3. Install YOLOv8:
```bash
git clone ***
***
***
```
### 2.2 Dataset Acquisition and Preprocessing
**Dataset Acquisition:**
* COCO dataset: Contains 80 object categories, used for training general-purpose object detection models.
* VOC dataset: Contains 20 object categories, used for training models in specific domains.
* Custom dataset: Collect images and annotations that meet the specific task requirements.
**Dataset Preprocessing:**
1. **Image preprocessing:** Resize images to a uniform size and perform normalization.
2. **Annotation preprocessing:** Convert annotation files to a format supported by YOLOv8, including class IDs, bounding box coordinates, and confidence scores.
3. **Dataset splitting:** Divide the dataset into training, validation, and test sets, typically in a ratio of 7:2:1.
**Code Example:**
```python
import os
from PIL import Image
import numpy as np
# Image preprocessing
def preprocess_image(image_path, input_size):
image = Image.open(image_path)
image = image.resize((input_size, input_size))
image = np.array(image) / 255.0
return image
# Annotation preprocessing
def preprocess_label(label_path, input_size):
with open(label_path, "r") as f:
labels = f.readlines()
labels = [label.strip().split(" ") for label in labels]
for label in labels:
label[1:] = [float(x) for x in label[1:]]
label[1:] = label[1:] * input_size
return labels
# Dataset splitting
def split_dataset(dataset_path, train_ratio=0.7, val_ratio=0.2):
images = os.listdir(dataset_path)
np.random.shuffle(images)
train_size = int(len(images) * train_ratio)
val_size = int(len(images) * val_ratio)
train_images = images[:train_size]
val_images = images[train_size:train_size+val_size]
test_images = images[train_size+val_size:]
return train_images, val_images, test_images
```
## 3. YOLOv8 Model Training and Evaluation
### 3.1 Training Configuration and Parameter Tuning
#### Training Configuration
The YOLOv8 model training configuration mainly includes the following parameters:
| Parameter | Description |
|---|---|
| batch_size | Training batch size |
| epochs | Number of training epochs |
| learning_rate | Learning rate |
| momentum | Momentum |
| weight_decay | Weight decay |
| warmup_epochs | Warm-up epochs |
| warmup_lr_multiplier | Warm-up learning rate multiplier |
| optimizer | Optimizer |
| loss | Loss function |
| lr_scheduler | Learning rate scheduler |
#### Parameter Tuning
Training configuration parameter tuning is a key step in improving model performance. Here are some common parameter tuning tips:
- **Batch size:** The batch size affects the training speed and model performance. Generally, a larger batch size can increase training speed but may lead to overfitting.
- **Learning rate:** The learning rate controls the speed of model parameter updates. A higher learning rate can accelerate training but may cause instability in the model.
- **Momentum:** Momentum can smooth gradient updates and help prevent the model from getting stuck in local optima.
- **Weight decay:** Weight decay can prevent overfitting but may reduce model performance.
- **Warm-up phase:** The warm-up phase gradually increases the learning rate, which helps with model stability.
##
0
0
相关推荐
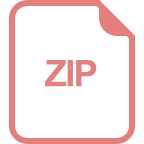
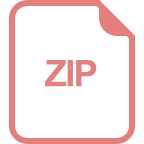
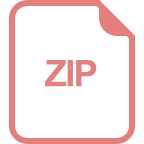





