Data Augmentation Techniques and Effect Evaluation in YOLOv8
发布时间: 2024-09-15 07:33:22 阅读量: 18 订阅数: 21 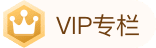
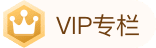
# Data Augmentation Techniques in YOLOv8 and Their Effectiveness Evaluation
Data augmentation is a common technique in the field of computer vision, used to expand the training dataset and improve the model's generalization ability. YOLOv8, as an advanced object detection algorithm, also extensively employs data augmentation techniques.
YOLOv8 offers a variety of data augmentation methods, including image transformation augmentation, geometric transformation augmentation, and mosaic augmentation. These methods can effectively alter the distribution of training images, forcing the model to learn more general features, thereby enhancing its detection performance in different scenarios.
# Data Augmentation Techniques in YOLOv8 in Practice
### Image Transformation Augmentation
#### Random Scaling and Cropping
Random scaling and cropping are common techniques in image transformation augmentation, aimed at altering the size and position of images to increase the model's robustness to targets of different sizes and positions.
```python
import cv2
def random_scale_and_crop(image, min_scale=0.5, max_scale=1.5):
"""
Randomly scale and crop the image.
Parameters:
image: Input image.
min_scale: Minimum scaling factor.
max_scale: Maximum scaling factor.
Returns:
Scaled and cropped image.
"""
# Randomly scale the image
scale = np.random.uniform(min_scale, max_scale)
scaled_image = cv2.resize(image, (0, 0), fx=scale, fy=scale)
# Randomly crop the image
height, width, channels = scaled_image.shape
crop_size = np.random.randint(height, size=1)[0]
crop_x = np.random.randint(width - crop_size + 1)
crop_y = np.random.randint(height - crop_size + 1)
cropped_image = scaled_image[crop_y:crop_y + crop_size, crop_x:crop_x + crop_size, :]
return cropped_image
```
*The `random_scale_and_crop()` function accepts an image as input and randomly scales and crops it.*
*The `min_scale` and `max_scale` parameters specify the minimum and maximum scaling factors.*
*The function first uses `cv2.resize()` to randomly scale the image.*
*Then, it uses `np.random.randint()` to randomly crop a subregion from the image.*
*Finally, it returns the scaled and cropped image.*
#### Color Space Conversion
Color space conversion is another commonly used technique in image transformation augmentation, aimed at altering the color distribution of images to increase the model's robustness to different color conditions.
```python
import cv2
def color_space_conversion(image):
"""
Color space conversion.
Parameters:
image: Input image.
Returns:
Image after color space conversion.
"""
# Convert the image from BGR color space to HSV color space
hsv_image = cv2.cvtColor(image, cv2.COLOR_BGR2HSV)
# Randomly adjust the hue, saturation, and value of the image
hue = np.random.uniform(-180, 180)
saturation = np.random.uniform(0.5, 1.5)
value = np.random.uniform(0.5, 1.5)
hsv_image[:, :, 0] += hue
hsv_image[:, :, 1] *= saturation
hsv_image[:, :, 2] *= value
# Convert the image back from HSV color space to BGR color space
bgr_image = cv2.cvtColor(hsv_image, cv2.COLOR_HSV2BGR)
return bgr_image
```
*The `color_space_conversion()` function accepts an image as input and converts its color space to HSV.*
*Then, it randomly adjusts the hue, saturation, and value of the image.*
*Finally, it converts the image back from HSV color space to BGR color space.*
### Geometric Transformation Augmentation
#### Random Rotation and Flipping
Random rotation and flipping are common techniques in geometric transformation augmentation, aimed at altering the rotation and flipping of images to increase the model's robustness to targets in different perspectives and orientations.
```python
import cv2
def random_rotation_and_flip(image):
"""
Randomly rotate and flip the image.
Parameters:
image: Input image.
Returns:
Image after rotation and flipping.
"""
# Randomly rotate the image
angle = np.random.uniform(-180, 180)
rotated_image = cv2.rotate(image, cv2.ROTATE_90_CLOCKWISE, angle)
# Randomly horizontally flip the image
if np.random.rand() > 0.5:
flipped_image = cv2.flip(rotated_image, 1)
else:
flipped_image = rotated_image
return flipped_image
```
*The `random_rotation_and_flip()` function accepts an image as input and randomly rotates and flips it.*
*The `angle` parameter specifies the angle of rotation for the image.*
*The `cv2.ROTATE_90_CLOCKWISE` parameter specifies a clockwise rotation of 90 degrees.*
*The `cv2.flip()` function horizontally flips the image.*
#### Perspective Transformatio
0
0