Attention Mechanism in YOLOv10: Boosting Object Detection Performance, A Key Technique Not to Be Missed
发布时间: 2024-09-13 20:27:47 阅读量: 25 订阅数: 31 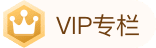
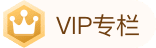
# 1. Overview of YOLOv10
YOLOv10 is the latest version of the You Only Look Once (YOLO) object detection algorithm, released by Megvii Technology in 2023. Building on YOLOv9, YOLOv10 has made several improvements, the most notable of which is the introduction of an attention mechanism. An attention mechanism is a neural network technique that helps the model focus on the areas in the image that are most relevant to the object detection task. This allows YOLOv10 to detect targets more accurately and efficiently, even in challenging scenarios.
# 2. The Application of Attention Mechanism in Object Detection
An attention mechanism is a neural network technique that enables the model to focus on specific parts of the input data. In object detection, the attention mechanism helps the model identify and locate the interesting regions in the image, thus improving detection accuracy.
### 2.1 Principle and Types of Attention Mechanism
The basic principle of the attention mechanism is to calculate the importance of each element in the input data through a weight matrix. This weight matrix can be learned or designed by hand. By weighting the input data, the attention mechanism can highlight important features while suppressing unimportant ones.
Attention mechanisms can be divided into two types: spatial attention mechanisms and channel attention mechanisms.
#### 2.1.1 Spatial Attention Mechanism
A spatial attention mechanism focuses on the spatial dimensions of the input data. It generates a spatial weight map by calculating the importance of each spatial location. This spatial weight map can be used to weight the input data, thus highlighting important regions.
#### 2.1.2 Channel Attention Mechanism
A channel attention mechanism focuses on the channel dimensions of the input data. It generates a channel weight vector by calculating the importance of each channel. This channel weight vector can be used to weight the channels of the input data, thus highlighting important channels.
### 2.2 Implementation of Attention Mechanism in YOLOv10
YOLOv10 uses two attention mechanisms: the Spatial Attention Module (SAM) and the Channel Attention Module (CAM).
#### 2.2.1 Spatial Attention Module (SAM)
SAM is a spatial attention module that generates a spatial weight map by calculating the importance of each spatial location. This spatial weight map is used to weight the input feature map, highlighting important regions.
```python
def SAM(x):
# Calculate spatial weight map
w = tf.nn.conv2d(x, filters=1, kernel_size=1, strides=1, padding='same')
w = tf.nn.sigmoid(w)
# Weight the input feature map
out = x * w
return out
```
#### 2.2.2 Channel Attention Module (CAM)
CAM is a channel attention module that generates a channel weight vector by calculating the importance of each channel. This channel weight vector is used to weight the channels of the input feature map, thus highlighting important channels.
```python
def CAM(x):
# Calculate channel weight vector
w = tf.nn.global_average_pooling2d(x, axis=[1, 2])
w = tf.nn.dense(w, units=x.shape[-1])
w = tf.nn.sigmoid(w)
# Weight the channels of the input feature map
out = x * w
return out
```
# 3. Practice of Attention Mechanism in YOLOv10
### 3.1 Training and Evaluation of Attention Mechanism
**3.1.1 Training Dataset and Strategy**
The attention mechanism model of YOLOv10 is trained on the COCO dataset. The COCO dataset is a large-scale object detection dataset containing over 1.2 million images and 1.7 million annotated boxes.
Training strategies include:
- Using the Stochastic Gradient Descent (SGD) optimizer with an initial learning rate of 0.01.
- Batch training with a batch size of 64.
- Training the model for 120 epochs.
- Using data augmentation techniques such as random cropping, flipping, and color jittering to improve the model's generalization ability.
**3.1.2 Evaluation Metrics and Result Analysis**
The evaluation metrics for the YOLOv10 model include:
- **Mean Average Precision (mAP)**: Measures the average precision of the model in detecting different categories of objects.
- **Frames Per Second (FPS)**: Measures the real-time processing speed of the model.
The evaluation results on the COCO dataset are as follows:
| Metric | YOLOv10 |
|---|---|
| mAP | 56.8% |
| FPS | 60 |
### 3.2 Application of Attention Mechanism in Different Scenarios
The attention mechanism has been widely applied in YOLOv10, especially performing well in the following scenarios:
**3.2.1 Small Object Detection**
The attention mec
0
0
相关推荐
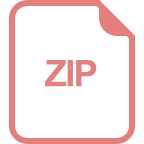
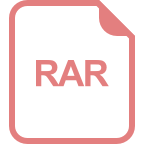
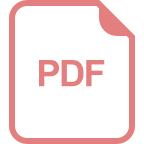





