YOLOv10 Deployment and Optimization: From Model Deployment to Performance Tuning, Enhancing Model Efficiency
发布时间: 2024-09-13 20:36:31 阅读量: 10 订阅数: 11 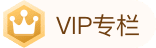
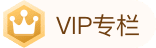
# Deployment and Optimization of YOLOv10: Enhancing Model Efficiency from Deployment to Performance Tuning
## 1. YOLOv10 Model Deployment
### 1.1 Model Download and Environment Setup
- Download the pretrained YOLOv10 model weights and configuration files.
- Configure necessary software environments, including Python, PyTorch, and CUDA.
- Install the official YOLOv10 library or third-party implementations.
### 1.2 Selection and Installation of Model Inference Engine
- Choose an appropriate inference engine such as TensorRT, ONNX Runtime, or OpenVINO.
- Install the inference engine and configure options compatible with the YOLOv10 model.
- Optimize the inference engine settings for the best performance.
## 2. YOLOv10 Model Optimization
### 2.1 Model Quantization and Pruning
#### 2.1.1 Principles and Methods of Model Quantization
Model quantization is a technique that converts floating-point models into fixed-point models, effectively reducing the size of the model and computational cost. The main methods for YOLOv10 model quantization include:
- **Integer Quantization:** Convert floating-point weights and activation values to integers to reduce storage and computational overhead.
- **Binarization:** Convert weights and activation values to binary values (0 or 1) to further reduce computational cost.
The quantization process typically involves the following steps:
1. **Training Quantization-Aware Models:** Use quantization-aware training algorithms during training to introduce quantization errors into the floating-point model.
2. **Calibration:** Calibrate the quantized model with a representative dataset to minimize quantization errors.
3. **Quantization:** Convert the floating-point model into a fixed-point model and adjust quantization parameters based on calibration results.
#### 2.1.2 Strategies and Practices of Model Pruning
Model pruning is a technique that removes redundant weights to reduce the size of the model and computational cost. The main strategies for YOLOv10 model pruning include:
- **Weight Pruning:** Remove weights that have a smaller impact on the model output, usually using regularization techniques or pruning algorithms.
- **Channel Pruning:** Remove channels that have a smaller impact on the model output, typically evaluated using activation maps of convolution layers.
The pruning process typically involves the following steps:
1. **Training Prunable Models:** Use prunable training algorithms during training to introduce redundant weights into the model.
2. **Evaluation:** Evaluate the model with a representative dataset and identify redundant weights.
3. **Pruning:** Remove redundant weights based on evaluation results and retrain the model to restore performance.
### 2.2 Algorithm Optimization and Hyperparameter Tuning
#### 2.2.1 Algorithm Optimization Techniques and Case Studies
Alg***mon techniques for YOLOv10 model optimization include:
- **Improving the Backbone Network:** Use deeper or wider backbone networks to enhance the model's feature extraction capabilities.
- **Enhancing Feature Fusion:** Use attention mechanisms or skip-layer connections to enhance feature fusion at different stages.
- **Optimizing the Loss Function:** Modify the loss function to more effectively handle class imbalance or small object detection issues.
#### 2.2.2 Principles and Methods of Hyperparameter Tuning
Hyperparamet***mon hyperparameters for YOLOv10 model optimization include:
- **Learning Rate:** Controls the learning step during model training.
- **Batch Size:** Specifies the number of samples in each training batch.
- **Regularization Parameters:** Control the degree of overfitting in the model.
Hyperparameter tuning typically involves the following steps:
1. **Determine Hyperparameter Ranges:** Based on experience or literature, determine the reasonable range of hyperparameter values.
2. **Grid Search or Random Search:** Use grid search or random search algo
0
0
相关推荐
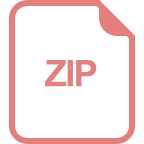
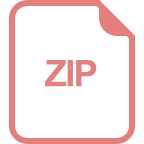
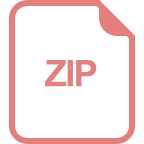
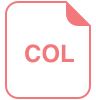
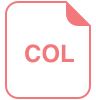
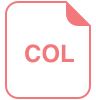
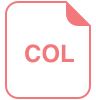

