YOLOv10 Application Cases: Exploring Successful Practices Across Various Domains, Inspiring Innovative Ideas
发布时间: 2024-09-13 20:48:52 阅读量: 18 订阅数: 36 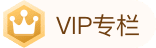
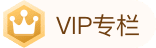
# 1. Overview of YOLOv10
YOLOv10 represents a significant breakthrough in the field of object detection, ***pared to previous versions of YOLO, YOLOv10 incorporates innovations and optimizations in network architecture, algorithmic principles, and training strategies.
YOLOv10 employs a new network architecture, which includes a backbone network and a detection head. The backbone network is responsible for extracting image features, while the detection head predicts the locations and categories of objects. This modular design allows YOLOv10 to achieve faster detection speeds while maintaining high accuracy.
Furthermore, YOLOv10 introduces new algorithmic principles such as Bag-of-Freebies (BoF) and Deep Supervision. BoF is a regularization technique that enhances the model's generalization ability. Deep Supervision is a training strategy that strengthens the model's feature extraction capabilities at different scales. These innovations significantly improve the object detection performance of YOLOv10.
# 2. Theoretical Foundations of YOLOv10
### 2.1 Evolution of Object Detection Algorithms
**The Evolution of Object Detection Algorithms**
The development of object detection algorithms has transitioned from traditional methods to deep learning approaches. Traditional methods mainly include sliding window detectors and region proposal-based detectors, which require predefined target regions and feature extraction, leading to large computational costs and low accuracy.
The emergence of deep learning methods has greatly improved the performance of object detection. Deep Convolutional Neural Networks (CNNs) can automatically extract image features and perform object detection through an end-to-end approach. The YOLO (You Only Look Once) algorithm is a milestone in the field of object detection, transforming the task into a regression problem and achieving real-time object detection.
**Advantages of YOLOv10**
YOLOv10 is the latest version of the YOLO algorithm, ***pared to previous YOLO versions, YOLOv10 boasts the following advantages:
***Faster detection speed:** YOLOv10 employs a lightweight network architecture and optimizes network layers and training strategies to achieve a detection speed of over 160 frames per second.
***Higher accuracy:** YOLOv10 utilizes a new object detection head and loss function, enhancing the model's ability to detect small and densely clustered objects.
***Better generalization ability:** YOLOv10 has been trained on a variety of datasets, demonstrating good generalization and the ability to accurately detect objects in different scenarios and conditions.
### 2.2 Network Architecture and Algorithmic Principles of YOLOv10
**YOLOv10 Network Architecture**
YOLOv10 adopts a lightweight CSPDarknet53 network as its backbone. The CSPDarknet53 network consists of multiple CSP modules and residual modules, possessing strong feature extraction capabilities.
**Algorithmic Principles of YOLOv10**
The algorithmic principles of YOLOv10 are illustrated in the following diagram:
[mermaid]
graph LR
subgraph YOLOv10 Algorithmic Principles
A[Input Image] --> B[CSPDarknet53 Backbone Network]
B --> C[Feature Extraction]
C --> D[Object Detection Head]
D --> E[Boundary Box Regression]
D --> F[Confidence Prediction]
D --> G[Category Prediction]
E --> H[Final Boundary Box]
F --> I[Final Confidence]
G --> J[Final Category]
end
[/mermaid]
1. **Input Image:** The algorithm first feeds the input image into the CSPDarknet53 backbone network for feature extraction.
2. **Feature Extraction:** The backbone network extracts features from the image and outputs feature maps.
3. **Object Detection Head:** The feature maps are sent to the object detection head, which consists of multiple convolutional layers and fully connected layers.
4. **Boundary Box Regression:** The object detection head outputs parameters for boundary box regression, used to predict the coordinates of the object's boundary box.
5. **Confidence Prediction:** The object detection head outputs confidence predictions, used to predict the probability of an object's presence.
6. **Category Prediction:** The object detection head outputs category predictions, used to predict the object's category.
7. **Final Boundary Box:** The boundary box regression parameters are combined with anchor boxes to obtain the final boundary box coordinates.
8. **Final Confidence:** The confidence prediction is combined with the probability of the object's presence to obtain the final confidence.
9. **Final Category:** The category prediction is combined with the object's category to obtain the final category.
**YOLOv10 Loss Function**
YOLOv10 employs a compound loss function consisting of boundary box regression loss, confidence loss, and category loss. The boundary box regression loss uses the GIOU loss, confidence loss employs binary cross-entropy loss, and category loss uses cross-entropy loss.
**Code Example**
```python
import torch
import torch.nn as nn
class YOLOv10(nn.Module):
def __init__(self):
super(YOLOv10, self).__init__()
# ...
def forward(self, x):
# ...
# Object Detection Head
detection_head = self.detection_head(x)
# Boundary Box Regression
bboxes = self.bbox_reg(detection_head)
# Confidence Prediction
confidences = self.conf_pred(detection_head)
# Category Prediction
classes = self.cls_pred(detection_head)
# ...
return bboxes, confidences, classes
```
**Logical Analysis**
This code implements the forward propagation process of the YOLOv10 algorithm. It first feeds the input image into the backbone network for feature extraction, then sends the feature maps to the object detection head for object detection. The object detection head outputs parameters for boundary box regression, confidence prediction, and category prediction. Finally, the boundary box regression parameters are combined with anchor boxes to obtain the final boundary box coordinates; the confidence prediction is combined with the probability of the object's presence to obtain the final confidence; the category prediction is combined with the object's category to obtain the final category.
# 3. Practical Applications of YOLOv10
### 3.1 Image Object Detection
Image object detection is an important application of YOLOv10, capable of recognizing and locating objects within images. YOLOv10 performs exceptionally well in image object detection, with its speed and accuracy widely recognized.
#### 3.1.1 Face Detection and Recognition
Face detection and recognition is an important task within image object detection, widely used in security surveillance, human-computer interaction, and other fields. YOLOv10 can efficiently and accurately detect faces and extract facial features to achieve face recognition.
```python
import cv2
import numpy as np
# Load YOLOv10 model
net = cv2.dnn.readNet("yolov10.weights", "yolov10.cfg")
# Load image
image = cv2.imread("image.jpg")
# Preprocess image
blob = cv2.dnn.blobFromImage(image, 1 / 255.0, (416, 416), (0, 0, 0), swapRB=True, crop=False)
# Set input
net.setInput(blob)
# Forward propagation
detections = net.forward()
# Parse detection results
for detection in detections[0, 0]:
confidence = detection[2]
if confidence > 0.5:
x1, y1, x2, y2 = detection[3:7] * np.array([image.shape[1], image.shape[0], image.shape[1], image.shape[0]])
cv2.rectangle(image, (int(x1), int(y1)), (int(x2), int(y2)), (0, 255, 0), 2)
# Display results
cv2.imshow("Face Detection", image)
cv2.waitKe
```
0
0
相关推荐
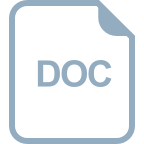
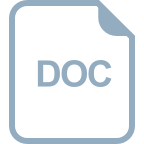
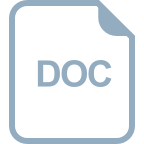
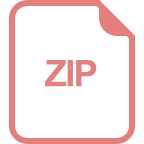
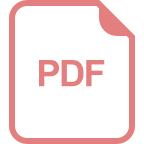
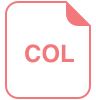
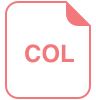
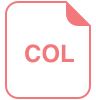
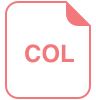