YOLOv8 Data Augmentation Techniques: Exploring Image Preprocessing and Augmentation Strategies
发布时间: 2024-09-14 00:42:42 阅读量: 56 订阅数: 21 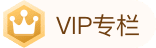
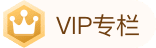
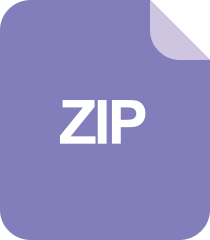
(179979052)基于MATLAB车牌识别系统【带界面GUI】.zip
# Introduction to Data Augmentation Techniques for YOLOv8: Image Preprocessing and Augmentation Strategies
Data augmentation is an image processing technique used to generate new training data to enhance the generalization capabilities of machine learning models. In the field of object detection, the YOLOv8 model is widely utilized for object detection tasks. Data augmentation is crucial for training the YOLOv8 model as it helps the model learn various changes of objects in images, thereby improving its robustness and accuracy.
# Image Preprocessing Techniques
### 2.1 Image Sizing and Scaling
Image resizing and scaling are common and important operations in image preprocessing. Their purpose is to adjust images to a specific size or aspect ratio to meet model input or other processing requirements.
#### 2.1.1 Scaling and Stretching
Scaling and stretching operations can change the dimensions of an image to fit a predetermined aspect ratio. Scaling will either shrink or enlarge an image to a specified size, whereas stretching will expand or compress an image to a specified size.
```python
import cv2
# Scale the image to the specified size
image = cv2.imread("image.jpg")
scaled_image = cv2.resize(image, (224, 224))
# Stretch the image to the specified size
stretched_image = cv2.resize(image, (300, 150))
```
**Parameter Explanation:**
* `image`: The input image
* `(width, height)`: The specified image dimensions
**Logical Analysis:**
* The `cv2.resize()` function uses bilinear interpolation to scale or stretch the image.
* The `(width, height)` parameter specifies the dimensions of the scaled or stretched image.
#### 2.1.2 Cropping and Padding
Cropping and padding operations can extract a specific region from an image or pad an image to a specified size. Cropping will cut out a region of the specified size from the image, while padding will add pixels of a specified color around the edges of the image.
```python
import cv2
# Crop the image
cropped_image = image[y:y+h, x:x+w]
# Pad the image
padded_image = cv2.copyMakeBorder(image, top, bottom, left, right, cv2.BORDER_CONSTANT, value=[0, 0, 0])
```
**Parameter Explanation:**
* `image`: The input image
* `(x, y, w, h)`: The coordinates and size of the cropping region
* `(top, bottom, left, right)`: The size of the padding region
* `value`: The color of the pixels to pad
**Logical Analysis:**
* The statement `image[y:y+h, x:x+w]` crops the specified region from the image.
* The `cv2.copyMakeBorder()` function pads the image with pixels of a specified color around the edges.
### 2.2 Image Color Space Conversion
Image color space conversion transforms an image from one color space (e.g., RGB) to another (e.g., grayscale or HSV). This can enhance certain features of an image or make it more suitable for specific tasks.
#### 2.2.1 RGB to Grayscale
The RGB to grayscale conversion transforms an image from the RGB color space to the grayscale color space. Grayscale images only contain luminance information and do not include color information.
```python
import cv2
# RGB to grayscale conversion
gray_image = cv2.cvtColor(image, cv2.COLOR_RGB2GRAY)
```
**Parameter Explanation:**
* `image`: The input image
* `cv2.COLOR_RGB2GRAY`: The color space conversion code
**Logical Analysis:**
* The `cv2.cvtColor()` function uses a weighted average method to convert an RGB image to a grayscale image.
#### 2.2.2 RGB to HSV
The RGB to HSV conversion transforms an image from the RGB color space to the HSV color space. The HSV color space includes three components: Hue, Saturation, and Value.
```python
import cv2
# RGB to HSV conversion
hsv_image = cv2.cvtColor(image, cv2.COLOR_RGB2HSV)
```
**Parameter Explanation:**
* `image`: The input image
* `cv2.COLOR_RGB2HSV`: The color space conversion code
**Logical Analysis:**
* The `cv2.cvtColor()` function uses a nonlinear transformation to convert an RGB image to an HSV image.
### 2.3 Image Noise Processing
Image noise is the unnecessary random variation in an image that can affect the quality and interpretability of the image. Image noise processing can remove or reduce noise in an image.
#### 2.3.1 Gaussian Noise
Gaussian noise is a common type of image noise that follows a normal distribution. Gaussian noise can smooth an image by removing high-frequency noi
0
0