Exploring the Application of YOLOv8 in Real-world Scenarios: Sharing Practical Experience in Object Detection
发布时间: 2024-09-14 00:50:57 阅读量: 11 订阅数: 16 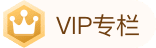
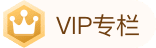
# Discussion on the Application of YOLOv8 in Real-World Scenarios: Sharing Practical Experience in Object Detection
## 1. Overview and Principle of YOLOv8
YOLOv8 is the latest generation of YOLO (You Only Look Once) object detection algorithm developed by the Ultralytics team. It continues the advantages of the YOLO series algorithms, such as fast speed and high accuracy, and introduces multiple innovations and optimizations on this basis.
YOLOv8 adopts a new Backbone network structure called Cross-Stage Partial Connections (CSP). CSP structures reduce the amount of computation by connecting residual blocks in series and using partial connections, thus improving the model's inference speed. In addition, YOLOv8 introduces the Path Aggregation Network (PAN) module, which fuses feature maps from different stages together, enhancing the model's feature extraction ability.
## 2. Practical Application of YOLOv8
### 2.1 Preparation and Preprocessing of Datasets
#### 2.1.1 Obtaining and Annotating Datasets
Datasets are the foundation for training YOLOv8 models, and choosing high-quality, diverse datasets is crucial. Here are some methods for obtaining datasets:
- **Public Datasets:** such as COCO, VOC, ImageNet, etc., which provide a large number of well-annotated images and annotations.
- **Custom Datasets:** Collect and annotate data for specific application scenarios to ensure that the dataset closely matches the actual application sc***
***mon annotation tools include:
- **LabelImg:** an open-source graphical interface annotation tool that supports rectangular and polygonal annotations.
- **CVAT:** a web-based annotation tool that supports image, video, and point cloud annotations.
- **VGG Image Annotator:** an annotation tool developed by the Visual Geometry Group at Oxford University that supports various types of annotations.
#### 2.1.2 Data Preprocessing and Augmentation
Data preprocessing and augmentation are key steps to improving the model'***mon preprocessing methods include:
- **Image Scaling and Cropping:** Scale or crop images to a uniform size to meet the model input requirements.
- **Color Space Conversion:** Convert images from RGB to other color spaces such as HSV or Lab to enhance the model's robustness to lighting and color variations.
- **Data Normalization:** Normalize image pixel values to the range of [0, 1] or [-1, 1] to accelerate model training.
Data augmentation techniques generate more training samples by randomly transforming the original data, thus improving the model'***mon augmentation methods include:
- **Random Flipping:** Horizontally or vertically flip images to increase the model's robustness to different perspectives.
- **Random Rotation:** Randomly rotate images to enhance the model's robustness to rotation invariance.
- **Random Cropping:** Randomly crop different areas of images to increase the model's robustness to local features.
- **Random Blurring:** Apply Gaussian blur to images to enhance the model's robustness to noise and blurriness.
### 2.2 Model Training and Evaluation
#### 2.2.1 Setting and Tuning Training Parameters
YOLOv8 training parameters include:
- **Learning Rate:** Controls the step size of model weight updates, affecting the model's convergence speed and generalization ability.
- **Batch Size:** The number of samples trained at once, affecting the model's training speed and memory usage.
- **Iterations:** The total number of rounds of training the model, affecting the model's convergence and generalization ability.
- **Weight Decay:** A regularization technique to prevent model overfitting and improve the model's generalization ability.
- **Momentum:** An optimization algorithm that accelerates model convergence and improves model stability.
Parameter tuning is an iterative process that achieves a balance between training speed, model accuracy, and generalization ability by adjusting parameter values.
#### ***
***mon evaluation metrics include:
- **Mean Average Precision (mAP):** Measures the average detection accuracy of the model across different categories.
- **Recall:** Measures the proportion of all real objects detected by the model.
- **Accuracy:** Measures the proportion of objects predicted correctly by the model.
- **Inference Speed:** Measures the model's inference speed on specific hardware.
The optimal model is selected based on the evaluation results, which achieves the best balance in accuracy, generalization ability, and inference speed.
## 3. Deployment and Optimization of YOLOv8
### 3.1 Model Deployment and Inference
#### 3.1.1 Deployment Environment and Platform for the Model
The deployment of YOLOv8 models can be chosen based on actual needs, ***mon deployment environments include:
- **Local Deployment:** Deploy the model on local servers or edge devices to achieve real-time inference.
- **Cloud Deployment:** Deploy the model on cloud platforms, utilizing the资源优势 ***
***mon deployment platforms include:
- **CPU:** Suitable for scenarios with low inference speed requirements and lower costs.
- **GPU:** Suitable for scenarios with higher inference speed requirements and more complex models.
- **TPU:** Suitable for scenari
0
0
相关推荐
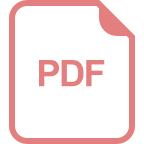
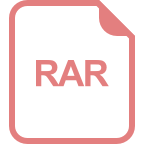
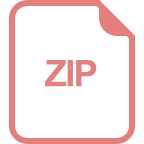





