Post-processing Techniques in YOLOv8: In-depth Analysis of Non-Maximum Suppression Algorithm
发布时间: 2024-09-14 00:48:55 阅读量: 51 订阅数: 28 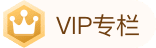
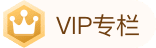
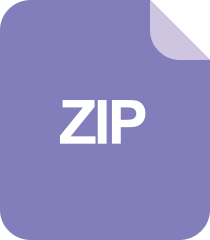
yolov8系列--Real time people counting system in computer vis.zip
# Post-processing Tricks in YOLOv8: In-depth Analysis of the Non-Maximum Suppression Algorithm
## 1. Overview of the Non-Maximum Suppression Algorithm
The Non-Maximum Suppression (NMS) algorithm is a classic technique used in the post-processing phase of object detection. Its purpose is to select the most representative bounding boxes from a set of overlapping detections to enhance detection accuracy and reduce redundancy. NMS works by calculating the overlap between bounding boxes, suppressing those with a higher degree of overlap with the box of highest confidence, thereby keeping the most representative boxes.
## 2. Theoretical Foundations of the NMS Algorithm
### 2.1 Intersection over Union (IoU) Calculation
Within the NMS algorithm, Intersection over Union (IoU) is a critical metric used to measure the overlap between two bounding boxes. The formula for IoU is as follows:
```python
IoU(box1, box2) = area_of_intersection / area_of_union
```
Here, `area_of_intersection` is the area of the intersection between the two bounding boxes, and `area_of_union` is the area of the union of the two bounding boxes.
### 2.2 NMS Algorithm Flow
The basic process of the NMS algorithm is as follows:
1. Obtain all detected bounding boxes based on the output of the object detection model.
2. Calculate the degree of overlap between all bounding boxes using IoU values.
3. Start with the bounding box with the highest IoU value and traverse all bounding boxes in turn.
4. For each bounding box, check the IoU value with previously processed bounding boxes.
5. If the IoU value exceeds the set threshold, suppress that bounding box.
6. Repeat steps 4 and 5 until all bounding boxes have been processed.
### 2.3 Variants of the NMS Algorithm
To enhance the performance and robustness of the NMS algorithm, researchers have proposed various variants, including:
- **Soft-NMS Algorithm:** The Soft-NMS algorithm performs a smooth handling of IoU values, avoiding the problem of bounding box loss caused by hard thresholds.
- **DIoU-NMS Algorithm:** The DIoU-NMS algorithm introduces directional information on top of IoU, making the NMS algorithm more robust to the rotation and deformation of bounding boxes.
- **CIoU-NMS Algorithm:** The CIoU-NMS algorithm further improves upon DIoU-NMS by incorporating information about the distance between centers and the aspect ratio, making the NMS algorithm more robust to changes in the shape of bounding boxes.
These NMS algorithm variants have demonstrated different performance advantages in various object detection tasks, allowing developers to choose based on specific requirements.
## 3. Application of the NMS Algorithm in YOLOv8
### 3.1 YOLOv8 NMS Algorithm Implementation
In YOLOv8, the NMS algorithm is mainly used to filter the candidate boxes output by the object detection model to remove redundant boxes with higher overlap, thereby improving detection accuracy. YOLOv8 employs an NMS implementation based on a greedy algorithm, with the following specific process:
1. **Sort by Confidence:** Sort all candidate boxes in descending order of confidence.
2. **Select the Highest Confidence Box:** Choose the box with the highest confidence as the box to be retained.
3. **Calculate IoU with the Retained Box:** Calculate the IoU (Intersection over Union) between the retained box and all other candidate boxes.
4. **Remove Candidate Boxes with High IoU:** If the IoU of other candidate boxes with the retained box is greater than the set threshold (commonly 0.5), then they are removed.
5. **Repeat Steps 2-4:** Repeat steps 2-4 until all candidate boxes have been processed.
```python
def nms(bboxes, scores, iou_threshold):
"""YOLOv8 NMS algorithm implementation.
Args:
bboxes: Candidate box coordinates, shape [N, 4].
scores: Confidence scores of candidate boxes, shape [N].
iou_threshold: IoU threshold.
Returns:
Indices of retained boxes, shape [K].
"""
# Sort by confidence in descending order
order = scores.argsort()[::-1]
# Initialize the list of retained box indices
keep = []
# Traverse candidate boxes
while order.size > 0:
# Select the box with the highest confidence
i = order[0]
keep.append(i)
# Calculate IoU with the retained box
ious = bbox_iou(bboxes[i], bboxes[order[1:]])
# Remove candidate boxes with high IoU
order = order[1:][ious < iou_threshold]
return keep
```
### 3.2 Impact of the NMS Algorithm on YOLOv8 Detection Accuracy
The NMS algorithm has a significant impact on YOLOv8's detection accuracy. By adjusting the IoU threshold of NMS, a balance can be struck between the number of retained boxes and detection accuracy.
When the IoU threshold is low, more candidate boxes are retained, whi
0
0
相关推荐







