YOLOv10 in Target Tracking: Exploring Its Potential, Empowering Intelligent Tracking
发布时间: 2024-09-13 20:30:17 阅读量: 27 订阅数: 36 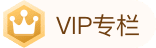
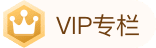
# 1. Overview of YOLOv10
YOLOv10 represents a groundbreaking advancement in the field of object detection, integrating object detection and tracking tasks for real-time and high-precision object tracking. Utilizing a one-stage detection framework, YOLOv10 simultaneously predicts object locations and categories in a single forward pass, effectively addressing issues of object loss and drift prevalent in traditional tracking methods.
The network architecture of YOLOv10 is based on YOLOv5 but has been optimized for object tracking tasks. It incorporates temporal information and object association mechanisms, enhancing the model's robustness to object movement and occlusion. Furthermore, YOLOv10 employs attention mechanisms and knowledge distillation techniques to further improve the tracking accuracy and generalization capabilities of the model.
# 2. Theoretical Basis of YOLOv10 in Object Tracking
### 2.1 Fundamental Principles of Object Tracking
Object tracking is a critical task in the field of computer vision aimed at estimating the position and state of objects within consecutive video frames. Object tracking algorithms typically follow these steps:
1. **Object Initialization:** The algorithm initializes the object's location and size in the first frame using manual annotation or other methods.
2. **Object Prediction:** Based on the object's location in the previous frame and a motion model, the algorithm predicts the object's position in the current frame.
3. **Object Matching:** The algorithm searches for objects in the current frame that match the predicted location.
4. **Object Update:** The algorithm updates the object's location and state based on the matched object.
### 2.2 Network Architecture and Algorithm Design of YOLOv10
YOLOv10 is a one-stage object detection algorithm that transforms the object tracking task into an object detection task. The network architecture of YOLOv10 mainly consists of the following parts:
- **Backbone Network:** YOLOv10 uses CSPDarknet53 as the backbone network, which is characterized by being lightweight and high-precision.
- **Neck Network:** The Neck network is responsible for fusing the feature maps extracted by the backbone network to enhance the semantic information of the features.
- **Detection Head:** The detection head is responsible for generating the bounding boxes and confidence scores of the objects.
The algorithmic designs adopted by YOLOv10 in object tracking include:
- **IoU Loss:** YOLOv10 uses an Intersection over Union (IoU) loss function to measure the overlap between predicted and true bounding boxes. A smaller IoU loss indicates that the predicted bounding box is closer to the true bounding box.
- **GIOU Loss:** YOLOv10 also introduces the Generalized IoU (GIOU) loss function, which considers not only the overlapping area but also the shape similarity of the bounding boxes.
- **DIoU Loss:** The Distance IoU (DIoU) loss function further considers the distance between the center points of the bounding boxes to improve the accuracy of bounding box prediction.
**Code Block:**
```python
import torch
import torch.nn as nn
class YOLOv10(nn.Module):
def __init__(self):
super(YOLOv10, self).__init__()
# Backbone network
self.backbone = CSPDarknet53()
# Neck network
self.neck = PANet()
# Detection head
self.detection_head = DetectionHead()
def forward(self, x):
# Backbone network
features = self.backbone(x)
# Neck network
features = self.neck(features)
# Detection head
outputs = self.detection_head(features)
return outputs
```
**Code Logic Analysis:**
This code block defines the structure of the YOLOv10 model, which includes a backbone network, a Neck network, and a detection head. The backbone network is responsible for feature extraction, the Neck network for feature fusion, and the detection head for generating the bounding boxes and confidence scores of objects.
**Parameter Description:**
- `x`: The input image.
- `features`: The feature maps extracted by the backbone network.
- `outputs`: The output generated by the detection head, including bounding boxes and confidence scores.
# 3.1 Dataset Preparation and Model Training
**Dataset Prep
0
0
相关推荐
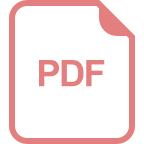
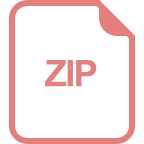
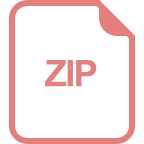
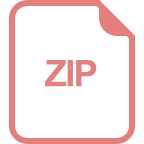
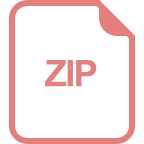
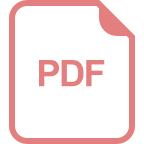
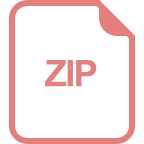
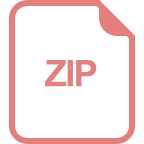
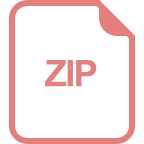