Anchor Box Strategy in YOLOv10: The Foundation for Optimizing Object Detection, Enhancing Model Accuracy
发布时间: 2024-09-13 20:29:09 阅读量: 38 订阅数: 42 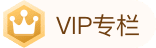
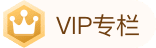
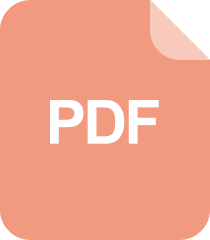
Optimizing the F-measure for Threshold-free Salient Object Detection (ICCV 2019)
# The Anchoring Strategy in YOLOv10: The Cornerstone of Optimizing Object Detection, Enhancing Model Accuracy
# 1. An Overview of Object Detection
Object detection is a fundamental task in computer vision, aiming to identify and localize specific objects within images or videos. Unlike image classification, which only requires the recognition of objects, object detection also needs to determine their positions in the image.
Object detection algorithms generally consist of two steps: the first is to generate candidate regions, which are image areas that may contain targets; the second is to classify these candidate regions and predict the bounding boxes of the targets. The anchoring strategy is an essential component of object detection algorithms, as it provides guidance for the generation of candidate regions.
# 2. Fundamental Theories of the Anchoring Strategy
### 2.1 The Concept and Role of Anchor Boxes
In object detection tasks, an anchor box (or prior box) is a predefined rectangular box that represents potential positions and sizes where objects may appear. The anchoring strategy is a crucial component of object detection models, determining how the model maps features in the input image to target bounding boxes.
The main roles of anchor boxes include:
- **Providing Prior Knowledge:** Anchor boxes provide the model with prior knowledge about potential positions and sizes of objects. This helps the model learn features of target bounding boxes more effectively during training.
- **Reducing Search Space:** The anchoring strategy breaks the object detection task down into a series of classification and regression problems. By using anchor boxes, the model can limit the search space to the areas covered by the anchor boxes, reducing computational complexity.
- **Improving Localization Accuracy:** Anchor boxes can help the model locate objects more accurately. By regressing the anchor boxes, the model can predict the offset of the target bounding box relative to the anchor box, resulting in more precise target bounding boxes.
### 2.2 The Generation Mech***
***mon methods include:
- **Based on Image Size:** Divide the image into a grid and generate multiple anchor boxes in each grid cell, with the size and shape of the anchor boxes determined by the image size.
- **Based on Feature Map Size:** Divide the feature map into a grid and generate multiple anchor boxes in each grid cell, with the size and shape of the anchor boxes determined by the feature map size.
- **Based on Clustering:** Cluster the target bounding boxes in the training set and use the cluster centers as anchor boxes.
#### Code Example:
```python
import numpy as np
def generate_anchors(image_size, feature_map_size, anchor_scales, anchor_ratios):
"""
Generates anchor boxes based on image size.
Parameters:
image_size: The size of the image, (height, width)
feature_map_size: The size of the feature map, (height, width)
anchor_scales: Scales of the anchor boxes
anchor_ratios: Aspect ratios of the anchor boxes
Returns:
anchors: Generated anchor boxes, (num_anchors, 4)
"""
image_height, image_width = image_size
feature_height, feature_width = feature_map_size
anchor_scales = np.array(anchor_scales)
anchor_ratios = np.array(anchor_ratios)
num_anchors = len(anchor_scales) * len(anchor_ratios)
anchors = np.zeros((num_anchors, 4))
for i in range(len(anchor_scales)):
for j in range(len(anchor_ratios)):
anchor_height = anchor_scales[i] * image_height / feature_height
anchor_width = anchor_scales[i] * image_width / feature_width
anchor_center_x = (j + 0.5) * image_width / feature_width
anchor_center_y = (i + 0.5) * image_height / feature_height
anchors[i * len(anchor_ratios) + j, :] = [anchor_center_x, anchor_center_y, anchor_width, anchor_height]
return anchors
```
#### Code Logic Analysis:
This code generates anchor boxes based on the image size. It divides the image into a grid and generates multiple anchor boxes in each grid cell. The size and shape of the anchor boxes are determined by the image size and predefined anchor scales and aspect ratios.
#### Parameter Explanation:
- `image_size`: The size of the image, formatted as `(height, width)`.
- `feature_map_size`: The size of the feature map, formatted as `(height, width)`.
- `anchor_scales`: Anchor scales, representing the ratio of anchor boxes to image size.
- `anchor_ratios`: Anchor aspect ratios, representing the ratio of width to height of anchor boxes.
#
0
0