Deployment and Optimization of YOLOv8 Model on Mobile Devices
发布时间: 2024-09-15 07:19:49 阅读量: 29 订阅数: 22 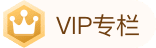
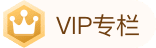
# Introduction to the YOLOv8 Model
YOLOv8 is one of the most advanced real-time object detection models, known for its exceptional accuracy and speed. It is based on the YOLOv7 architecture and incorporates various innovative technologies, including Cross-Stage Partial Connections (CSP) and Spatial Attention Module (SAM), further enhancing model performance.
The YOLOv8 model consists of a backbone network and a detection head. The backbone network is responsible for extracting image features, while the detection head is responsible for predicting object bounding boxes and class probabilities. YOLOv8 uses an Anchor-Free mechanism, directly predicting the center points and sizes of objects without predefined Anchor boxes.
# YOLOv8 Model Deployment Techniques
### 2.1 Model Quantization
#### 2.1.1 Quantization Principles and Methods
Model quantization is a technique that converts floating-point models into fixed-point models, reducing model size and computational cost by lowering the precision of model parameters and activation values. The principle of quantization is to approximate high-precision floating-point values with low-precision fixed-point values, ***
***mon quantization methods include:
- **Uniform Quantization:** Mapping floating-point values uniformly to a fixed-point range.
- **Non-uniform Quantization:** Mapping floating-point values to a fixed-point range based on their distribution, to minimize quantization errors.
#### 2.1.2 Quantization Tools and Practices
Deep learning frameworks like PyTorch and TensorFlow provide quantization tools, such as:
- **PyTorch:** quantization module
- **TensorFlow:** quantization-aware training module
Quantization practice steps:
1. **Prepare the model:** Convert the trained floating-point model into a quantized model.
2. **Choose a quantization method:** Select an appropriate quantization method based on the model and deployment requirements.
3. **Quantize the model:** Use quantization tools to quantize model parameters and activation values to fixed-point values.
4. **Evaluate performance:** Compare the accuracy and speed of the quantized model with the floating-point model.
### 2.2 Model Pruning
#### 2.2.1 Pruning Principles and Methods
Model pruning is a technique that reduces model size by removing redundant or unimportant parts of the model. The principle of pruning is based on the assumption that:
- There are redundant or unimportant ***
***
***mon pruning methods include:
- **Weight Pruning:** Removing unimportant weights from the model.
- **Neuron Pruning:** Removing unimportant neurons from the model.
#### 2.2.2 Pruning Tools and Practices
Deep learning frameworks like PyTorch and TensorFlow provide pruning tools, such as:
- **PyTorch:** prune module
- **TensorFlow:** pruning module
Pruning practice steps:
1. **Prepare the model:** Convert the trained floating-point model into a pruned model.
2. **Choose a pruning method:** Select an appropriate pruning method based on the model and deployment requirements.
3. **Prune the model:** Use pruning tools to remove redundant parts of the model.
4. **Evaluate performance:** Compare the accuracy and speed of the pruned model with the floating-point model.
### 2.3 Model Distillation
#### 2.3.1 Distillation Principles and Methods
Model distillation is a technique that transfers knowledge from a large teacher model to a smaller student model. The principle of distillation is based on the assumption that:
- The large teacher model contains rich knowledge and features.
- The smaller student model can learn these knowledge and features from the teacher model.
Distillation methods achieve knowledge transfer by minimizing the loss function between the teacher and student models. Loss functions include:
- **Classification Loss:** Measures the performance of the student model on classification tasks.
- **Knowledge Distillation Loss:** Measures the knowledge the student model learns from the teacher model.
#### 2.3.2 Distillation Tools and Practices
Deep learning frameworks like PyTorch and TensorFlow provide distillation tools, such as:
- **PyTorch:** distillation module
- **TensorFlow:** knowledge_distillation module
Distillation practice steps:
1. **Prepare the model:** Train a large teacher model and a smaller student model.
2. **Choose a distillation method:** Select an appropriate distillation method based on the model and deployment requirements.
3. **Distill the model:** Use distillation tools to transfer knowledge from the teacher model to the student model.
4. **Evaluate performance:** Compare the accuracy and speed of the distilled model with the floating-point model.
# YOLOv8 Model Optimization Tips
### 3.1 Algorithm
0
0
相关推荐
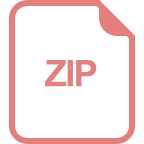
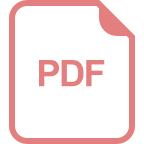
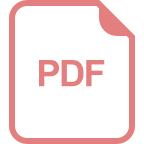
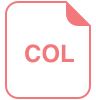
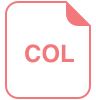
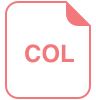
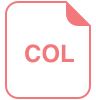

