Optimization Methods for YOLOv8 Model: Network Pruning and Quantization
发布时间: 2024-09-15 07:31:59 阅读量: 46 订阅数: 23 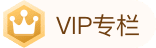
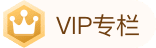
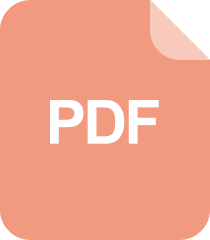
First-Order and Stochastic Optimization Methods for Machine Learning.pdf

# Optimization Techniques for the YOLOv8 Model: Network Pruning and Quantization
## 1. Introduction to YOLOv8 Model
YOLOv8 is the latest object detection algorithm released by Megvii Technology in 2022, which has achieved significant improvements in both speed and accuracy. YOLOv8 adopts a new network architecture and incorporates various optimization techniques, giving it outstanding performance in a wide range of application scenarios.
The network structure of YOLOv8 employs CSPDarknet53 as the backbone network, characterized by its lightweight and high efficiency. Building upon CSPDarknet53, YOLOv8 also introduces a new PAN path aggregation module, which effectively fuses features of different scales, thereby improving the model's detection accuracy.
Beyond network architecture optimization, YOLOv8 also employs a variety of optimization techniques, including:
***Data Augmentation Techniques:** YOLOv8 employs a variety of data augmentation techniques, such as random scaling, cropping, flipping, etc., to enhance the model's generalization capability.
***Loss Function Optimization:** YOLOv8 adopts a new loss function that can effectively balance classification loss and regression loss, thereby improving the model's detection accuracy.
***Training Strategy Optimization:** YOLOv8 adopts a new training strategy that can effectively improve the model's convergence speed and accuracy.
## ***work Pruning Optimization
### 2.1 Overview of Pruning Strategies
Pruning is a network optimization technique that reduces the model size and computational requirements by removing unimportant weights or channels. Pruning strategies can be broadly categorized into two types:
#### 2.1.1 Weight Pruning
Weight pruning involves removing unimportant weights from the model. The importance of weights can be measured by their absolute values, gradients, ***mon weight pruning algorithms include:
- **L1 Norm Pruning:** Removing weights with the smallest absolute values.
- **L2 Norm Pruning:** Removing weights with the smallest norms.
- **Gradient Pruning:** Removing weights with the smallest gradients.
#### 2.1.2 Channel Pruning
Channel pruning involves removing unimportant channels from the model. The importance of channels can be measured by their activation values, gradients, ***mon channel pruning algorithms include:
- **Max Average Pooling Pruning:** Removing channels with the smallest max average pooling values.
- **L1 Norm Pruning:** Removing channels with the smallest absolute values.
- **Gradient Pruning:** Removing channels with the smallest gradients.
### 2.2 Pruning Algorithms
Pruning algorithms can be broadly classified into two categories:
#### 2.2.1 Sparsification Pruning
Sparsification pruning creates sparse models by setting weights or channels to zero. Sparsification pruning algorithms include:
- **Threshold Pruning:** Setting weights or channels with absolute values below a threshold to zero.
- **Random Pruning:** Randomly removing weights or channels.
- **Structured Pruning:** Removing entire convolution kernels or channels.
#### 2.2.2 Structured Pruning
Structured pruning creates structured sparse models by removing entire convolution kernels or channels. Structured pruning algorithms include:
- **Pruning Convolution:** Removing entire convolution kernels.
- **Pruning Channels:** Removing entire channels.
- **Pruning Layers:** Removing entire layers.
### 2.3 Model Restoration After Pruning
After pruning, the model's accuracy may decline. To restore accuracy, ***mon restoration methods include:
- **Retraining:** Using the pruned model as initialization, retrain the model.
- **Fine-tuning:** Fine-tuning the pruned model to restore accuracy.
- **Knowledge Distillation:** Using knowledge distillation with the pruned model and an unpruned model to restore accuracy.
## 3. Quantization Optimization
### 3.1 Overview of Quantization
Quantization is a technique that converts floating-point data into fixed-point data, effectively reducing the model's storage and computational costs. In deep learning, quantization is often used to compress model size and increase inference speed.
#### 3.1.1 Types of Quantization
Quantization types are mainly divided into the following two:
- **Linear Quantization:** Linearly maps floating-point data to fixed-point data, maintaining the shape of the data distribution.
- **Symmetric Quantization:** Symmetrically maps floating-point data to fixed-point data, with the data distribution centered around zero.
#### 3.1.2 Methods of Quantization
Quantization methods are mainly divided into the following two:
- **Post-Training Quantization:** Quantizes model parameters and activation values after model training.
- **Training-Aware Quantization:** Incorporates quantization as part of the training process, allowing the model to maintain high accuracy after quantization.
### 3.2 Quantization Algorithms
#### 3.2.1 Linear Quantization
The linear quantization algorithm linearly maps floating-point data `x` to fixed-point data `y`:
```python
def linear_quantization(x, n_bits):
"""Linear quantization algorithm
Args:
x: Floating-point data
n_bits: Number of bits for fixed-point data
Returns:
Quantized fixed-point data
"""
min_val = np.min(x)
max_val = np.max(x)
scale = (max_val - min_val) / (2 ** n_bits - 1)
y = np.round((x - min_val) / scale)
return y
```
**Parameter Explanation:**
- `x`: Floating-point data
- `n_bits`: Number of bits for fixed-point data
**Code Logic Analysis:**
1. Calculate the minimum and maximum values of the floating-point data.
2. Calculate the quantization scale, which is the ratio of the floating-point data range to the fixed-point data range.
3. Subtract the minimum value from the floating-point data, then divide
0
0
相关推荐
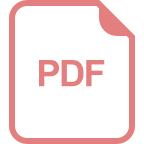
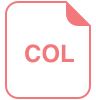
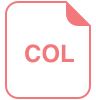
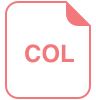
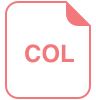
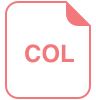
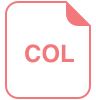
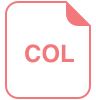