plt.plot(range(len(meiguo_Num["confirmNum"])-7,len(meiguo_Num["confirmNum"])),arima314.forecast(7)[0],color="red",label="预测值")
时间: 2023-11-21 09:44:50 浏览: 103
这段代码是在使用matplotlib库进行可视化操作,其中plt.plot()函数可以用来绘制线性图。在这里,它的第一个参数是x轴的范围,通过range()函数生成了一个长度为len(meiguo_Num["confirmNum"])-7的序列,表示预测值的横坐标范围。第二个参数arima314.forecast(7)[0]表示预测值的纵坐标,其中arima314是ARIMA模型对象,forecast(7)表示预测未来7天的值,[0]表示取出预测值的第一个元素。color参数指定线条颜色,label参数指定图例标签。
相关问题
temp = range(len(meiguo_Num["confirmNum"].values)) plt.plot(temp,meiguo_Num["confirmNum"].values)
这段代码使用了Python的matplotlib库,通过plt.plot函数绘制了美国新冠病例的累计确诊数曲线。其中,meiguo_Num["confirmNum"].values表示美国新冠病例的累计确诊数数据,而range(len(meiguo_Num["confirmNum"].values))则是用来生成x轴坐标的序列,表示该数据的第0个到第(len(meiguo_Num["confirmNum"].values)-1)个数据对应的x轴坐标。
绘制训练集和测试集的真实值和预测值图像 train_predict_plot = np.empty_like(data_scaled) train_predict_plot[:, :] = np.nan train_predict_plot[time_steps:len(train_predict) + time_steps, :] = train_predict test_predict_plot = np.empty_like(data_scaled) test_predict_plot[:, :] = np.nan test_predict_plot[len(train_predict) + time_steps * 2 + 1:len(data_scaled) - 1, :] = test_predict plt.figure(figsize=(10, 6)) plt.plot(scaler.inverse_transform(data_scaled)) plt.plot(train_predict_plot) plt.plot(test_predict_plot) plt.legend(['True', 'Train Predict', 'Test Predict']) plt.xlabel('Time/h') plt.ylabel('kwh') plt.show(改写以上代码,使得训练集部分和测试集部分分别绘制在两张图上
下面是改写后的代码,将训练集和测试集的真实值和预测值分别绘制在两张图上:
```
train_predict_plot = np.empty_like(data_scaled)
train_predict_plot[:, :] = np.nan
train_predict_plot[time_steps:len(train_predict) + time_steps, :] = train_predict
test_predict_plot = np.empty_like(data_scaled)
test_predict_plot[:, :] = np.nan
test_predict_plot[len(train_predict) + time_steps * 2 + 1:len(data_scaled) - 1, :] = test_predict
# 绘制训练集部分真实值和预测值图像
plt.figure(figsize=(10, 6))
plt.plot(scaler.inverse_transform(data_scaled[:len(train_predict)]))
plt.plot(train_predict_plot[:len(train_predict)])
plt.legend(['True', 'Train Predict'])
plt.xlabel('Time/h')
plt.ylabel('kwh')
plt.title('Training Set')
plt.show()
# 绘制测试集部分真实值和预测值图像
plt.figure(figsize=(10, 6))
plt.plot(scaler.inverse_transform(data_scaled[len(train_predict) + time_steps * 2 + 1:]))
plt.plot(test_predict_plot[len(train_predict) + time_steps * 2 + 1:])
plt.legend(['True', 'Test Predict'])
plt.xlabel('Time/h')
plt.ylabel('kwh')
plt.title('Test Set')
plt.show()
```
阅读全文
相关推荐
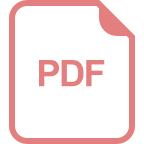













