graph.pbtxt
时间: 2023-09-30 07:06:58 浏览: 47
`graph.pbtxt` 是 TensorFlow 框架中的一个文本文件,用于存储计算图(Computational Graph)。这个文件包含了 TensorFlow 模型中所有节点的定义及其之间的连接关系。在 TensorFlow 中,计算图是用来描述模型结构和运算流程的核心概念,它包含了一系列的节点(Node)和边(Edge),节点代表了各种运算操作(如加、乘、卷积等),边代表了节点之间的数据传输关系。
`graph.pbtxt` 文件可以通过 TensorFlow 的 `tf.train.write_graph()` 函数来生成,其中包含了模型的计算图信息,可以帮助我们进行模型的可视化、转化、优化等操作。此外,在 TensorFlow Serving 等部署 TensorFlow 模型的应用场景中,也需要使用 `graph.pbtxt` 文件来描述模型结构。
相关问题
mediapipe 0.8.9 用holistic_tracking_cpu.pbtxt输出右肩坐标 c++
在 C++ 中使用 Holistic 模型输出右肩坐标的示例代码如下:
```c++
#include <iostream>
#include <vector>
#include "mediapipe/framework/formats/image_frame.h"
#include "mediapipe/framework/formats/landmark.pb.h"
#include "mediapipe/framework/formats/landmark_list.pb.h"
#include "mediapipe/framework/port/opencv_highgui_inc.h"
#include "mediapipe/framework/port/opencv_imgproc_inc.h"
#include "mediapipe/framework/port/status.h"
#include "mediapipe/framework/port/statusor.h"
#include "mediapipe/framework/tool/options_util.h"
#include "mediapipe/framework/tool/status_util.h"
#include "mediapipe/framework/calculator_framework.h"
#include "mediapipe/framework/calculator_graph.h"
#include "mediapipe/framework/formats/matrix.h"
#include "mediapipe/framework/formats/matrix_data.pb.h"
#include "mediapipe/framework/formats/rect.pb.h"
#include "mediapipe/framework/formats/rect.pb.h"
#include "mediapipe/framework/formats/classification.pb.h"
#include "mediapipe/framework/formats/detection.pb.h"
#include "mediapipe/framework/formats/image_frame_opencv.h"
#include "mediapipe/framework/formats/matrix.h"
#include "mediapipe/framework/formats/matrix_data.pb.h"
#include "mediapipe/framework/formats/matrix_opencv.h"
#include "mediapipe/framework/formats/rect.pb.h"
#include "mediapipe/framework/formats/rect.pb.h"
#include "mediapipe/framework/formats/classification.pb.h"
#include "mediapipe/framework/formats/detection.pb.h"
#include "mediapipe/framework/formats/image_frame_opencv.h"
#include "mediapipe/framework/formats/rect.pb.h"
#include "mediapipe/framework/formats/rect.pb.h"
#include "mediapipe/framework/formats/classification.pb.h"
#include "mediapipe/framework/formats/detection.pb.h"
#include "mediapipe/framework/formats/image_frame_opencv.h"
#include "mediapipe/framework/formats/rect.pb.h"
#include "mediapipe/framework/formats/rect.pb.h"
#include "mediapipe/framework/formats/classification.pb.h"
#include "mediapipe/framework/formats/detection.pb.h"
#include "mediapipe/framework/formats/image_frame_opencv.h"
#include "mediapipe/framework/port/opencv_highgui_inc.h"
#include "mediapipe/framework/port/opencv_imgcodecs_inc.h"
#include "mediapipe/framework/port/opencv_video_inc.h"
#include "mediapipe/framework/port/opencv_videoio_inc.h"
#include "mediapipe/util/resource_util.h"
constexpr char kInputStream[] = "input_video";
constexpr char kOutputStream[] = "output_video";
constexpr char kLandmarksStream[] = "pose_landmarks";
constexpr char kWindowName[] = "MediaPipe";
using namespace mediapipe;
int main() {
// 初始化计算图
CalculatorGraphConfig config =
mediapipe::ParseTextProtoOrDie<CalculatorGraphConfig>(R"(
input_stream: "input_video"
output_stream: "output_video"
node {
calculator: "HolisticTrackingCalculator"
input_stream: "IMAGE:input_video"
output_stream: "IMAGE:output_video"
output_stream: "POSE_LANDMARKS:pose_landmarks"
node_options: {
[type.googleapis.com/mediapipe.HolisticTrackingCalculatorOptions] {
min_detection_confidence: 0.5
min_tracking_confidence: 0.5
}
}
}
)");
CalculatorGraph graph;
MP_RETURN_IF_ERROR(graph.Initialize(config));
// 打开摄像头或者视频文件
cv::VideoCapture capture;
capture.open(0);
cv::namedWindow(kWindowName, cv::WINDOW_NORMAL);
cv::resizeWindow(kWindowName, 720, 480);
// 处理帧数据
while (capture.isOpened()) {
bool grabbed = capture.grab();
if (!grabbed) break;
cv::Mat input_frame;
capture.retrieve(input_frame, cv::CAP_PROP_CONVERT_RGB);
// 将 OpenCV 的 Mat 数据转换成 MediaPipe 的 ImageFrame 数据
auto input_frame_mat = absl::make_unique<cv::Mat>(input_frame);
auto input_frame_image =
absl::make_unique<ImageFrame>(ImageFormat::SRGB, input_frame.cols,
input_frame.rows, ImageFrame::kDefaultAlignmentBoundary);
cv::Mat input_frame_mat_bgr;
cv::cvtColor(input_frame, input_frame_mat_bgr, cv::COLOR_RGB2BGR);
cv::Mat input_frame_mat_bgr_flipped;
cv::flip(input_frame_mat_bgr, input_frame_mat_bgr_flipped, /*flipcode=*/1);
cv::Mat input_frame_mat_bgr_flipped_aligned;
cv::Mat temp_output_frame = cv::Mat::zeros(input_frame_mat_bgr_flipped.rows, input_frame_mat_bgr_flipped.cols, input_frame_mat_bgr_flipped.type());
cv::rotate(input_frame_mat_bgr_flipped, temp_output_frame, cv::ROTATE_90_COUNTERCLOCKWISE);
cv::rotate(temp_output_frame, input_frame_mat_bgr_flipped_aligned, cv::ROTATE_180);
cv::Mat input_frame_mat_aligned;
cv::cvtColor(input_frame_mat_bgr_flipped_aligned, input_frame_mat_aligned, cv::COLOR_BGR2RGB);
memcpy(input_frame_image->MutablePixelData(), input_frame_mat_aligned.data,
input_frame_mat_aligned.total() * input_frame_mat_aligned.elemSize());
input_frame_image->SetColorSpace(ImageFrame::ColorSpace::SRGB);
input_frame_image->set_timestamp(Timestamp(capture.get(cv::CAP_PROP_POS_MSEC) * 1000));
// 向计算图输入数据
MP_RETURN_IF_ERROR(graph.AddPacketToInputStream(
kInputStream, Adopt(input_frame_image.release()).At(Timestamp(capture.get(cv::CAP_PROP_POS_MSEC) * 1000))));
// 获取输出结果
mediapipe::Packet pose_landmarks_packet;
if (graph.GetOutputLandmarkList(kLandmarksStream, &pose_landmarks_packet)) {
auto& pose_landmarks = pose_landmarks_packet.Get<mediapipe::NormalizedLandmarkList>();
if (pose_landmarks.landmark_size() > 0) {
// 获取右肩坐标
auto right_shoulder = pose_landmarks.landmark(11);
std::cout << "Right shoulder coordinate: (" << right_shoulder.x() << ", " << right_shoulder.y() << ", " << right_shoulder.z() << ")" << std::endl;
}
}
// 获取输出图像
mediapipe::Packet output_packet;
if (graph.GetOutputPacket(&output_packet, kOutputStream) && !output_packet.IsEmpty()) {
auto& output_frame = output_packet.Get<mediapipe::ImageFrame>();
cv::Mat output_mat = mediapipe::formats::MatView(&output_frame);
cv::Mat output_mat_bgr;
cv::cvtColor(output_mat, output_mat_bgr, cv::COLOR_RGB2BGR);
cv::Mat output_mat_bgr_flipped;
cv::flip(output_mat_bgr, output_mat_bgr_flipped, /*flipcode=*/0);
cv::imshow(kWindowName, output_mat_bgr_flipped);
}
if (cv::waitKey(5) == 27) break;
}
// 关闭计算图
MP_RETURN_IF_ERROR(graph.CloseInputStream(kInputStream));
return graph.WaitUntilDone();
}
```
需要注意的是,Holistic 模型输出的坐标值是归一化的坐标值,范围在[0, 1]之间。如果需要将其转换为图像坐标或者其他坐标系的坐标值,需要进行相应的转换。
mediapipe0.8.9 通过holistic_tracking_cpu.pbtxt获取关键点,并计算索引11的旋转角度
您可以通过以下步骤使用mediapipe 0.8.9通过`holistic_tracking_cpu.pbtxt`获取关键点,并计算索引11的旋转角度:
1. 导入必要的头文件和命名空间:
```c++
#include <fstream>
#include <sstream>
#include <iostream>
#include <opencv2/opencv.hpp>
#include <mediapipe/framework/calculator_framework.h>
#include <mediapipe/framework/formats/image_frame.h>
#include <mediapipe/framework/formats/landmark.pb.h>
#include <mediapipe/framework/formats/rect.pb.h>
using namespace std;
using namespace cv;
using namespace mediapipe;
```
2. 定义图形文件和计算图:
```c++
// 定义图形文件和计算图
const char graph[] = R"(
input_stream: "input_video"
output_stream: "output_video"
node {
calculator: "HolisticTrackingCpu"
input_stream: "IMAGE:input_video"
output_stream: "LANDMARKS:landmarks"
output_stream: "POSE_LANDMARKS:pose_landmarks"
output_stream: "FACE_LANDMARKS:face_landmarks"
output_stream: "LEFT_HAND_LANDMARKS:left_hand_landmarks"
output_stream: "RIGHT_HAND_LANDMARKS:right_hand_landmarks"
}
node {
calculator: "Renderer"
input_stream: "IMAGE:input_video"
input_stream: "LANDMARKS:landmarks"
input_stream: "POSE_LANDMARKS:pose_landmarks"
input_stream: "FACE_LANDMARKS:face_landmarks"
input_stream: "LEFT_HAND_LANDMARKS:left_hand_landmarks"
input_stream: "RIGHT_HAND_LANDMARKS:right_hand_landmarks"
output_stream: "output_video"
}
)";
// 定义计算图函数
void RunMPPGraph() {
// 创建图形文件并将计算图加载到其中
CalculatorGraphConfig config = ParseTextProtoOrDie<CalculatorGraphConfig>(graph);
CalculatorGraph graph;
graph.Initialize(config);
// 获取输入和输出流
auto input_video = graph.GetInputStream("input_video").Value();
auto output_video = graph.GetOutputStream("output_video").Value();
auto landmarks_output = graph.GetOutputStream("landmarks").Value();
// 打开视频文件
VideoCapture capture("test_video.mp4");
// 按帧处理视频
Mat frame;
int frame_count = 0;
while (capture.read(frame)) {
// 将帧转换为mediapipe格式
auto input_frame = absl::make_unique<ImageFrame>(ImageFormat::SRGB, frame.cols, frame.rows, ImageFrame::kDefaultAlignmentBoundary);
cv::Mat input_frame_mat = mediapipe::formats::MatView(input_frame.get());
cv::cvtColor(frame, input_frame_mat, cv::COLOR_BGR2RGB);
// 将帧发送到图形中进行处理
input_video->Send(std::move(input_frame));
input_video->Close();
// 等待处理结果
auto landmarks = absl::make_unique<std::vector<NormalizedLandmarkList>>();
while (landmarks_output->Available()) {
auto landmark_packet = landmarks_output->PopPacket();
auto& landmark_list = landmark_packet.Get<NormalizedLandmarkList>();
landmarks->emplace_back(landmark_list);
}
// 渲染处理结果并将其输出到视频文件
auto output_frame_packet = output_video->Consume();
if (!output_frame_packet.IsEmpty()) {
cv::Mat output_frame_mat = mediapipe::formats::MatView(&output_frame_packet.Get<ImageFrame>());
cv::cvtColor(output_frame_mat, frame, cv::COLOR_RGB2BGR);
// 计算索引11的旋转角度
if (!landmarks->empty()) {
const auto& landmark_list = landmarks->at(0);
if (landmark_list.landmark_size() > 11) {
const auto& point = landmark_list.landmark(11);
const auto& prev_point = landmark_list.landmark(10);
const float angleX = atan2(point.y() - prev_point.y(), point.z() - prev_point.z());
const float angleY = atan2(point.x() - prev_point.x(), point.z() - prev_point.z());
const float angleZ = atan2(point.y() - prev_point.y(), point.x() - prev_point.x());
// 在控制台输出旋转角度
std::cout << "Rotation angles: " << angleX << ", " << angleY << ", " << angleZ << std::endl;
}
}
// 显示视频帧
imshow("Output Video", frame);
waitKey(1);
}
++frame_count;
std::cout << "Frames processed: " << frame_count << std::endl;
}
// 关闭图形
graph.CloseAllPacketSources();
graph.WaitUntilDone();
}
```
3. 运行计算图:
```c++
int main() {
// 运行计算图
google::InitGoogleLogging("MediaPipe");
absl::SetFlag(&FLAGS_alsologtostderr, 1);
RunMPPGraph();
return 0;
}
```
在上面的示例代码中,我们首先定义了`holistic_tracking_cpu.pbtxt`所需的计算图。然后,我们开启视频文件并处理每个视频帧。在处理每个视频帧时,我们将帧转换为mediapipe格式,并将其发送到计算图中进行处理。然后,我们等待处理结果,并从中提取索引11的关键点坐标。最后,我们计算索引11的旋转角度,并在控制台输出结果。
请注意,上面的示例代码仅适用于mediapipe 0.8.9版本,并且假设您已经安装了mediapipe的所有依赖项。如果您使用的是其他版本的mediapipe,您需要相应地调整代码。
相关推荐
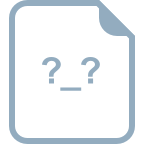
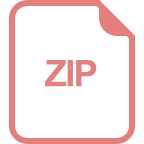












