ResNet50 Flatten层的作用
时间: 2023-07-24 18:05:13 浏览: 46
ResNet50模型中的Flatten层的作用是将最后一层卷积层输出的特征图(即具有深度的二维矩阵)展平成一维向量,以便于接下来的全连接层进行处理。也就是说,Flatten层将多个特征图中的所有特征点(或称为像素点)平铺成一个长向量,这个向量可以看作是该图像的特征向量,用于表示该图像的特征。这样可以将图像分类问题转化为一个常见的分类问题,即将高维的特征向量映射到类别标签上。
相关问题
resnet50最后flatten 层
ResNet50是一个深度神经网络模型,它的最后一层不是Flatten层,而是一个全局平均池化层(Global Average Pooling)。这个层的作用是将卷积核生成的特征图进行平均池化,将特征图转换为一个固定长度的向量,这个向量可以被输入到全连接层中进行分类或回归。这个全局平均池化层有利于减少模型的参数数量,防止过拟合,使得模型更加轻量化。因此,ResNet50的最后一层是一个全局平均池化层,而不是Flatten层。
resnet50全连接层
ResNet50是一个卷积神经网络模型,其全连接层通常被称为“分类层”,因为它将卷积层的输出转换为预测结果。在ResNet50中,全连接层由一个Flatten层、一个Dense层和一个Softmax层组成。
Flatten层将卷积层的输出展平成一个向量,使其可以被输入到Dense层中。Dense层是一个全连接层,其中包含一些神经元,每个神经元与前一层的每个神经元都连接。最后,Softmax层将Dense层的输出转换为概率分布,用于预测输入图像的类别。
需要注意的是,ResNet50的全连接层通常是在预训练的模型中定义好的,而在微调时可能会被替换或修改,以适应不同的任务或数据集。
相关推荐
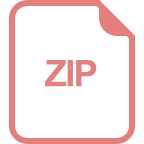
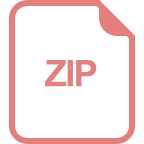












