请解释 for fold in range(2, 18): y_Pre1 = [] y_lbl1 = [] y_Pre = [[]] y_lbl = [[]]
时间: 2024-05-29 14:12:48 浏览: 101
这段代码使用了循环语句,使用变量`fold`从2到17进行循环,表示需要进行2到17次的操作。
在每次循环中,代码会创建4个空列表:`y_Pre1`、`y_lbl1`、`y_Pre`和`y_lbl`。
其中,`y_Pre1`和`y_lbl1`用于存储某些数据的预测值和标签值,`y_Pre`和`y_lbl`则是二维列表,用于存储每次循环中的所有`y_Pre1`和`y_lbl1`。
换句话说,每次循环中会生成一个`y_Pre`和`y_lbl`的列表,而这些列表会被添加到`y_Pre`和`y_lbl`这两个二维列表中,最终得到的就是一个包含多个`y_Pre`和`y_lbl`的二维列表。
相关问题
for k in k_choices: k_to_accuracies[k] = [] for i in range(num_folds): X_train_fold = np.concatenate([ fold for j, fold in enumerate(X_train_folds) if i != j ]) y_train_fold = np.concatenate([ fold for j, fold in enumerate(y_train_folds) if i != j ]) X_val = X_train_folds[i] y_val = y_train_folds[i] classifier.train(X_train_fold, y_train_fold) y_pred_fold = classifier.predict(X_val, k=k, num_loops=0) num_correct = np.sum(y_pred_fold == y_val) accuracy = float(num_correct) / X_val.shape[0] k_to_accuracies[k].append(accuracy)
这段代码是一个 k-fold 交叉验证的过程,用于评估分类器在不同 k 值下的准确率。其中,k_choices 是一个包含不同 k 值的列表,k_to_accuracies 是一个字典,用于存储每个 k 值对应的准确率列表。
在每个 k 值的循环中,首先将当前 k 值对应的准确率列表初始化为空。然后,在每个折叠循环中,通过 np.concatenate 将除了当前折叠之外的所有折叠样本合并为训练集 X_train_fold 和 y_train_fold。同时,将当前折叠样本作为验证集 X_val 和 y_val。
接下来,使用分类器的 train 方法在训练集上进行训练。然后,使用分类器的 predict 方法在验证集上进行预测,设置 k 值为当前循环的 k 值,num_loops 为 0。
计算预测正确的数量 num_correct,然后通过除以验证集的样本数量 X_val.shape[0] 得到准确率,并将其添加到当前 k 值对应的准确率列表中。
最终,返回包含不同 k 值对应准确率列表的字典 k_to_accuracies。
def get_k_fold_data(k, i, X, y): assert k > 1 fold_size = X.shape[0] // k X_train, y_train = None, None for j in range(k): idx = slice(j * fold_size, (j + 1) * fold_size) X_part, y_part = X[idx,:], y[idx] if j == i: X_valid, y_valid = X_part, y_part elif X_train is None: X_train, y_train = X_part, y_part else: X_train = nd.concat(X_train, X_part, dim=0) y_train = nd.concat(y_train, y_part, dim=0) return X_train, y_train, X_valid, y_valid 对代码进行注释
# 定义一个函数,用于生成 k 折交叉验证数据集
# k: 折数
# i: 当前为第 i 折作为验证集
# X: 特征数据
# y: 标签数据
def get_k_fold_data(k, i, X, y):
# 断言 k 的值必须大于 1
assert k > 1
# 计算每一折数据集的大小
fold_size = X.shape[0] // k
# 初始化训练集和验证集的特征数据和标签数据
X_train, y_train = None, None
# 遍历每一折数据集
for j in range(k):
# 计算当前折数据集的索引范围
idx = slice(j * fold_size, (j + 1) * fold_size)
# 划分出当前折的特征数据和标签数据作为验证集
X_part, y_part = X[idx,:], y[idx]
if j == i:
# 如果当前折是验证集,则将其作为验证集
X_valid, y_valid = X_part, y_part
elif X_train is None:
# 如果当前训练集为空,则将当前折的特征数据和标签数据作为训练集
X_train, y_train = X_part, y_part
else:
# 如果当前训练集不为空,则在训练集的特征数据和标签数据后面拼接上当前折的特征数据和标签数据
X_train = nd.concat(X_train, X_part, dim=0)
y_train = nd.concat(y_train, y_part, dim=0)
# 返回训练集和验证集的特征数据和标签数据
return X_train, y_train, X_valid, y_valid
阅读全文
相关推荐
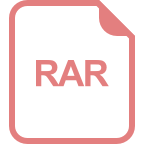
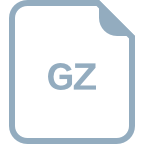
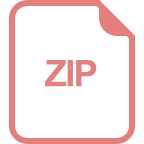














