flink insert into upsert
时间: 2023-10-07 13:09:28 浏览: 39
Flink supports "upsert" operations in its Table API and SQL. The "upsert" operation allows you to insert new records into a table and update the existing records if they already exist based on a specified key.
To perform an upsert operation in Flink, you would typically follow these steps:
1. Create a Flink table using the desired schema and define a primary key on the table.
2. Convert the input data stream or batch dataset into a table.
3. Use the `upsertInto` method to specify the target table and perform the upsert operation.
Here's an example using the Flink Table API:
```java
// Create a StreamExecutionEnvironment
StreamExecutionEnvironment env = StreamExecutionEnvironment.getExecutionEnvironment();
// Create a StreamTableEnvironment
StreamTableEnvironment tableEnv = StreamTableEnvironment.create(env);
// Define the schema of your table
TableSchema schema = TableSchema.builder()
.field("id", DataTypes.INT())
.field("name", DataTypes.STRING())
.primaryKey("id")
.build();
// Convert a DataStream into a Table
DataStream<Tuple2<Integer, String>> stream = ...; // your input data stream
Table table = tableEnv.fromDataStream(stream, Schema.newBuilder()
.fromTableSchema(schema)
.build());
// Upsert the table into another table
tableEnv.executeSql("CREATE TABLE target_table (id INT PRIMARY KEY, name STRING)");
table.executeInsert("target_table").upsert(table);
```
Note that this is just a basic example to illustrate the concept. You can adapt it to your specific use case and customize it further as needed.
相关推荐
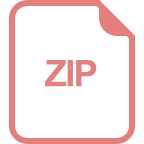
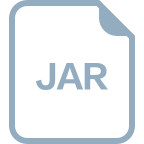














