tensorflow建立session代码
时间: 2023-08-30 08:10:44 浏览: 64
以下是tensorflow建立session的示例代码:
```python
import tensorflow as tf
# 创建一个常量tensor
a = tf.constant(2)
b = tf.constant(3)
# 创建一个加法操作
add = tf.add(a, b)
# 创建一个乘法操作
mul = tf.multiply(a, b)
# 创建会话
with tf.Session() as sess:
# 执行加法和乘法操作
print("加法操作的结果: %i" % sess.run(add))
print("乘法操作的结果: %i" % sess.run(mul))
```
在这个示例中,我们首先创建了两个常量tensor `a` 和 `b`。然后,我们创建了加法和乘法操作 `add` 和 `mul`,分别将tensor `a` 和 `b` 相加和相乘。最后,在 `with` 语句中创建了一个会话,使用 `sess.run()` 方法来执行加法和乘法操作,并将结果打印出来。在完成操作后,会话会自动关闭。
相关问题
tensorflow建立神经网络实例代码
这是一个使用 TensorFlow 建立并训练简单的神经网络的代码示例:
```python
import tensorflow as tf
# 定义输入和输出
x = tf.placeholder(tf.float32, shape=[None, 28, 28, 1])
y = tf.placeholder(tf.float32, shape=[None, 10])
# 建立卷积层
conv1 = tf.layers.conv2d(x, 32, 5, activation=tf.nn.relu)
# 建立池化层
pool1 = tf.layers.max_pooling2d(conv1, 2, 2)
# 建立更多的卷积和池化层
conv2 = tf.layers.conv2d(pool1, 64, 3, activation=tf.nn.relu)
pool2 = tf.layers.max_pooling2d(conv2, 2, 2)
# 建立全连接层
flat = tf.reshape(pool2, [-1, 64 * 7 * 7])
dense = tf.layers.dense(flat, 1024, activation=tf.nn.relu)
# 建立输出层
logits = tf.layers.dense(dense, 10)
prediction = tf.nn.softmax(logits)
# 定义损失函数和优化器
loss = tf.losses.sparse_softmax_cross_entropy(labels=y, logits=logits)
train_op = tf.train.AdamOptimizer(learning_rate=0.001).minimize(loss)
# 定义评估指标
accuracy = tf.metrics.accuracy(labels=tf.argmax(y, axis=1), predictions=tf.argmax(prediction, axis=1))[1]
# 初始化变量
init_op = tf.group(tf.global_variables_initializer(), tf.local_variables_initializer())
# 开始训练
with tf.Session() as sess:
sess.run(init_op)
for step in range(10000):
sess.run(train_op, feed_dict={x: x_train, y: y_train})
if step % 100 == 0:
acc = sess.run(accuracy, feed_dict={x: x_test, y: y_test})
print("Step: %d, Test accuracy: %f" % (step, acc))
```
这段代码建立了一个含有两个卷积层和两
dqn代码 tensorflow
以下是一个DQN算法的TensorFlow代码示例:
```python
import tensorflow as tf
import numpy as np
import gym
# 神经网络类
class DQN:
def __init__(self, n_actions, n_features, learning_rate=0.01, reward_decay=0.9, e_greedy=0.9, replace_target_iter=300, memory_size=500, batch_size=32, e_greedy_increment=None):
self.n_actions = n_actions
self.n_features = n_features
self.lr = learning_rate
self.gamma = reward_decay
self.epsilon_max = e_greedy
self.replace_target_iter = replace_target_iter
self.memory_size = memory_size
self.batch_size = batch_size
self.epsilon_increment = e_greedy_increment
self.epsilon = 0 if e_greedy_increment is not None else self.epsilon_max
self.learn_step_counter = 0
self.memory = np.zeros((self.memory_size, n_features * 2 + 2))
self.build_net()
t_params = tf.get_collection('target_net_params')
e_params = tf.get_collection('eval_net_params')
self.replace_target_op = [tf.assign(t, e) for t, e in zip(t_params, e_params)]
self.sess = tf.Session()
self.sess.run(tf.global_variables_initializer())
self.cost_his = []
# 建立神经网络
def build_net(self):
self.s = tf.placeholder(tf.float32, [None, self.n_features], name='s')
self.q_target = tf.placeholder(tf.float32, [None, self.n_actions], name='Q_target')
with tf.variable_scope('eval_net'):
c_names = ['eval_net_params', tf.GraphKeys.GLOBAL_VARIABLES]
n_l1 = 10
w_initializer = tf.random_normal_initializer(0., 0.3)
b_initializer = tf.constant_initializer(0.1)
with tf.variable_scope('l1'):
w1 = tf.get_variable('w1', [self.n_features, n_l1], initializer=w_initializer, collections=c_names)
b1 = tf.get_variable('b1', [1, n_l1], initializer=b_initializer, collections=c_names)
l1 = tf.nn.relu(tf.matmul(self.s, w1) + b1)
with tf.variable_scope('l2'):
w2 = tf.get_variable('w2', [n_l1, self.n_actions], initializer=w_initializer, collections=c_names)
b2 = tf.get_variable('b2', [1, self.n_actions], initializer=b_initializer, collections=c_names)
self.q_eval = tf.matmul(l1, w2) + b2
with tf.variable_scope('loss'):
self.loss = tf.reduce_mean(tf.squared_difference(self.q_target, self.q_eval))
with tf.variable_scope('train'):
self._train_op = tf.train.RMSPropOptimizer(self.lr).minimize(self.loss)
self.s_ = tf.placeholder(tf.float32, [None, self.n_features], name='s_')
with tf.variable_scope('target_net'):
c_names = ['target_net_params', tf.GraphKeys.GLOBAL_VARIABLES]
n_l1 = 10
w_initializer = tf.random_normal_initializer(0., 0.3)
b_initializer = tf.constant_initializer(0.1)
with tf.variable_scope('l1'):
w1 = tf.get_variable('w1', [self.n_features, n_l1], initializer=w_initializer, collections=c_names)
b1 = tf.get_variable('b1', [1, n_l1], initializer=b_initializer, collections=c_names)
l1 = tf.nn.relu(tf.matmul(self.s_, w1) + b1)
with tf.variable_scope('l2'):
w2 = tf.get_variable('w2', [n_l1, self.n_actions], initializer=w_initializer, collections=c_names)
b2 = tf.get_variable('b2', [1, self.n_actions], initializer=b_initializer, collections=c_names)
self.q_next = tf.matmul(l1, w2) + b2
# 记忆
def store_transition(self, s, a, r, s_):
if not hasattr(self, 'memory_counter'):
self.memory_counter = 0
transition = np.hstack((s, [a, r], s_))
index = self.memory_counter % self.memory_size
self.memory[index, :] = transition
self.memory_counter += 1
# 选择动作
def choose_action(self, observation):
observation = observation[np.newaxis, :]
if np.random.uniform() < self.epsilon:
actions_value = self.sess.run(self.q_eval, feed_dict={self.s: observation})
action = np.argmax(actions_value)
else:
action = np.random.randint(0, self.n_actions)
return action
# 学习
def learn(self):
if self.learn_step_counter % self.replace_target_iter == 0:
self.sess.run(self.replace_target_op)
print('\ntarget_params_replaced\n')
if self.memory_counter > self.memory_size:
sample_index = np.random.choice(self.memory_size, size=self.batch_size)
else:
sample_index = np.random.choice(self.memory_counter, size=self.batch_size)
batch_memory = self.memory[sample_index, :]
q_next, q_eval4next = self.sess.run(
[self.q_next, self.q_eval],
feed_dict={
self.s_: batch_memory[:, -self.n_features:],
self.s: batch_memory[:, :self.n_features],
})
q_eval = self.sess.run(self.q_eval, {self.s: batch_memory[:, :self.n_features]})
q_target = q_eval.copy()
batch_index = np.arange(self.batch_size, dtype=np.int32)
eval_act_index = batch_memory[:, self.n_features].astype(int)
reward = batch_memory[:, self.n_features + 1]
q_target[batch_index, eval_act_index] = reward + self.gamma * np.max(q_next, axis=1)
_, self.cost = self.sess.run([self._train_op, self.loss],
feed_dict={self.s: batch_memory[:, :self.n_features],
self.q_target: q_target})
self.cost_his.append(self.cost)
self.epsilon = self.epsilon + self.epsilon_increment if self.epsilon < self.epsilon_max else self.epsilon_max
self.learn_step_counter += 1
# 训练网络
def main():
env = gym.make('CartPole-v0')
env = env.unwrapped
n_actions = env.action_space.n
n_features = env.observation_space.shape[0]
dqn = DQN(n_actions=n_actions, n_features=n_features, learning_rate=0.01, reward_decay=0.9, e_greedy=0.9, replace_target_iter=100, memory_size=2000, e_greedy_increment=0.001, )
for i_episode in range(400):
observation = env.reset()
ep_r = 0
while True:
env.render()
action = dqn.choose_action(observation)
observation_, reward, done, info = env.step(action)
x, x_dot, theta, theta_dot = observation_
r1 = (env.x_threshold - abs(x)) / env.x_threshold - 0.8
r2 = (env.theta_threshold_radians - abs(theta)) / env.theta_threshold_radians - 0.5
reward = r1 + r2
dqn.store_transition(observation, action, reward, observation_)
ep_r += reward
if dqn.memory_counter > dqn.memory_size:
dqn.learn()
if done:
print('episode: ', i_episode, 'ep_r: ', round(ep_r, 2), ' epsilon: ', round(dqn.epsilon, 2))
if done:
break
observation = observation_
print('game over')
env.close()
if __name__ == '__main__':
main()
```
这个代码演示了如何使用DQN算法来解决CartPole-v0游戏。在代码中,定义了一个DQN类,其中包含了神经网络的构建,记忆的存储,动作的选择和学习。在主函数中,创建了CartPole-v0环境,并使用DQN算法进行训练。
阅读全文
相关推荐
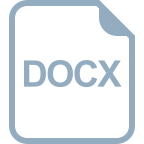
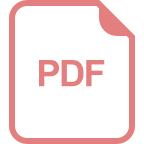
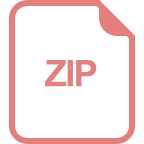
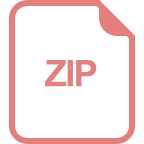
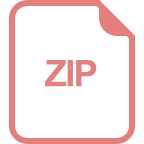
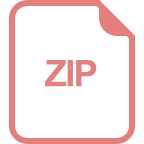
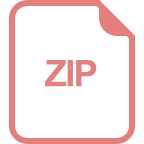
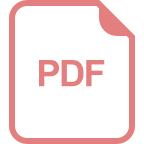
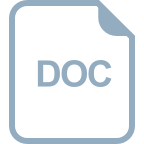
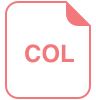
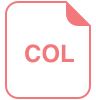
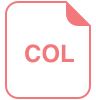

