feature_extract = True
时间: 2023-08-20 18:07:43 浏览: 67
这行代码用于设置一个布尔变量`feature_extract`为`True`。根据代码上下文的不同,`feature_extract`可能是用于控制模型的特征提取方式的一个标志。
当`feature_extract`为`True`时,表示模型将仅用于特征提取,即只使用预训练模型的卷积层(或部分卷积层)作为特征提取器,而不对模型的其他层进行训练。这在迁移学习中比较常见,可以通过固定预训练模型的参数来加快训练速度并减少内存消耗。
当`feature_extract`为`False`时,表示模型将进行端到端的训练,即对整个模型进行训练,包括预训练模型的参数和自定义添加的层。
具体的使用方式和含义可能需要根据代码的上下文来确定。
相关问题
详细解释一下这段代码,每一句都要进行注解:tgt = f'/kaggle/working/{dataset}-{scene}' # Generate a simple reconstruction with SIFT (https://en.wikipedia.org/wiki/Scale-invariant_feature_transform). if not os.path.isdir(tgt): os.makedirs(f'{tgt}/bundle') os.system(f'cp -r {src}/images {tgt}/images') database_path = f'{tgt}/database.db' sift_opt = pycolmap.SiftExtractionOptions() sift_opt.max_image_size = 1500 # Extract features at low resolution could significantly reduce the overall accuracy sift_opt.max_num_features = 8192 # Generally more features is better, even if behond a certain number it doesn't help incresing accuracy sift_opt.upright = True # rotation invariance device = 'cpu' t = time() pycolmap.extract_features(database_path, f'{tgt}/images', sift_options=sift_opt, verbose=True) print(len(os.listdir(f'{tgt}/images'))) print('TIMINGS --- Feature extraction', time() - t) t = time() matching_opt = pycolmap.SiftMatchingOptions() matching_opt.max_ratio = 0.85 # Ratio threshold significantly influence the performance of the feature extraction method. It varies depending on the local feature but also on the image type # matching_opt.max_distance = 0.7 matching_opt.cross_check = True matching_opt.max_error = 1.0 # The ransac error threshold could help to exclude less accurate tie points pycolmap.match_exhaustive(database_path, sift_options=matching_opt, device=device, verbose=True) print('TIMINGS --- Feature matching', time() - t) t = time() mapper_options = pycolmap.IncrementalMapperOptions() mapper_options.extract_colors = False mapper_options.min_model_size = 3 # Sometimes you want to impose the first image pair for initialize the incremental reconstruction mapper_options.init_image_id1 = -1 mapper_options.init_image_id2 = -1 # Choose which interior will be refined during BA mapper_options.ba_refine_focal_length = True mapper_options.ba_refine_principal_point = True mapper_options.ba_refine_extra_params = True maps = pycolmap.incremental_mapping(database_path=database_path, image_path=f'{tgt}/images', output_path=f'{tgt}/bundle', options=mapper_options) print('TIMINGS --- Mapping', time() - t)
这段代码主要是使用 PyCOLMAP 库实现对图像的特征提取、特征匹配和增量式三维重建。具体解释如下:
```tgt = f'/kaggle/working/{dataset}-{scene}'```
定义了一个字符串变量 tgt,表示输出路径。
```if not os.path.isdir(tgt):```
如果输出路径不存在,则创建该路径。
```os.makedirs(f'{tgt}/bundle')```
在输出路径下创建子目录 bundle。
```os.system(f'cp -r {src}/images {tgt}/images')```
将源目录 src 中的 images 目录复制到输出路径下的 images 目录中。
```database_path = f'{tgt}/database.db'```
定义一个字符串变量 database_path,表示 PyCOLMAP 库中使用的数据库文件路径。
```sift_opt = pycolmap.SiftExtractionOptions()```
创建一个 SIFT 特征提取选项对象。
```sift_opt.max_image_size = 1500```
设置 SIFT 特征提取选项对象的最大图像尺寸为 1500×1500 像素。
```sift_opt.max_num_features = 8192```
设置 SIFT 特征提取选项对象的最大特征点数为 8192 个。
```sift_opt.upright = True```
设置 SIFT 特征提取选项对象的旋转不变性为 True,即不考虑图像旋转。
```device = 'cpu'```
定义一个字符串变量 device,表示计算设备类型。
```pycolmap.extract_features(database_path, f'{tgt}/images', sift_options=sift_opt, verbose=True)```
调用 PyCOLMAP 库中的 extract_features 函数,对输出路径下的图像进行 SIFT 特征提取,并将特征保存到数据库文件中。
```print(len(os.listdir(f'{tgt}/images')))```
输出输出路径下的图像数量。
```print('TIMINGS --- Feature extraction', time() - t)```
输出特征提取所花费的时间。
```matching_opt = pycolmap.SiftMatchingOptions()```
创建一个 SIFT 特征匹配选项对象。
```matching_opt.max_ratio = 0.85```
设置 SIFT 特征匹配选项对象的最大匹配比率为 0.85。
```matching_opt.max_distance = 0.7```
设置 SIFT 特征匹配选项对象的最大匹配距离为 0.7。
```matching_opt.cross_check = True```
设置 SIFT 特征匹配选项对象的交叉匹配为 True,即同时匹配两幅图像。
```matching_opt.max_error = 1.0```
设置 SIFT 特征匹配选项对象的最大误差为 1.0。
```pycolmap.match_exhaustive(database_path, sift_options=matching_opt, device=device, verbose=True)```
调用 PyCOLMAP 库中的 match_exhaustive 函数,对数据库文件中的特征进行 SIFT 特征匹配,并将匹配结果保存到数据库文件中。
```print('TIMINGS --- Feature matching', time() - t)```
输出特征匹配所花费的时间。
```mapper_options = pycolmap.IncrementalMapperOptions()```
创建一个增量式三维重建选项对象。
```mapper_options.extract_colors = False```
设置增量式三维重建选项对象的颜色提取为 False,即不提取图像颜色信息。
```mapper_options.min_model_size = 3```
设置增量式三维重建选项对象的最小模型大小为 3。
```mapper_options.init_image_id1 = -1```
设置增量式三维重建选项对象的第一张图像的 ID 为 -1,表示不指定。
```mapper_options.init_image_id2 = -1```
设置增量式三维重建选项对象的第二张图像的 ID 为 -1,表示不指定。
```mapper_options.ba_refine_focal_length = True```
设置增量式三维重建选项对象的相机内参的优化为 True。
```mapper_options.ba_refine_principal_point = True```
设置增量式三维重建选项对象的相机主点的优化为 True。
```mapper_options.ba_refine_extra_params = True```
设置增量式三维重建选项对象的额外参数的优化为 True。
```maps = pycolmap.incremental_mapping(database_path=database_path, image_path=f'{tgt}/images', output_path=f'{tgt}/bundle', options=mapper_options)```
调用 PyCOLMAP 库中的 incremental_mapping 函数,对数据库文件中的匹配结果进行增量式三维重建,并将重建结果保存到输出路径下的 bundle 目录中。
```print('TIMINGS --- Mapping', time() - t)```
输出增量式三维重建所花费的时间。
feature_importances_df = pd.DataFrame({'Feature': selected_features, 'Importance': feature_importances}) in _extract_index raise ValueError("All arrays must be of the same length")
这个错误通常是由于选中的特征名称和特征重要性的数量不致所引起的。请确保创建 `feature_importances_df` 数据帧时,选中的特征名称和特征重要性具有相同的长度。
以下是一个修正后的示例代码:
```python
import pandas as pd
import numpy as np
from sklearn.ensemble import RandomForestClassifier
from sklearn.feature_selection import SelectFromModel
import matplotlib.pyplot as plt
# 1. 加载数据集
data = pd.read_csv('genotype_dataset.csv')
X = data.iloc[:, 1:] # 特征
y = data.iloc[:, 0] # 标签
# 2. 数据预处理(如果有需要)
# 3. 特征选择
rf = RandomForestClassifier(n_estimators=100, random_state=42)
rf.fit(X, y)
feature_importances = rf.feature_importances_
selector = SelectFromModel(rf, threshold='median', prefit=True)
X_selected = selector.transform(X)
selected_features = X.columns[selector.get_support()]
# 4. 输出结果为CSV文件(候选特征)
selected_data = pd.concat([y, pd.DataFrame(X_selected, columns=selected_features)], axis=1)
selected_data.to_csv('selected_features.csv', index=False)
# 5. 输出排名前50的特征为CSV文件
feature_importances_df = pd.DataFrame({'Feature': selected_features, 'Importance': feature_importances})
top_50_features = feature_importances_df.nlargest(50, 'Importance')
top_50_features.to_csv('top_50_features.csv', index=False)
# 6. 绘制特征重要性图表
plt.bar(feature_importances_df['Feature'], feature_importances_df['Importance'])
plt.xticks(rotation=90)
plt.xlabel('Feature')
plt.ylabel('Importance')
plt.title('Feature Importance')
plt.show()
```
在修正后的代码中,我将 `selected_features` 和 `feature_importances` 作为字典传递给 `pd.DataFrame`,以确保它们具有相同的长度。另外,我还添加了一个特征重要性的图表绘制部分。请确保你的数据集中的特征名称与代码中的特征名称一致,并确保已安装所需的Python库(如pandas、numpy、sklearn和matplotlib)。
相关推荐
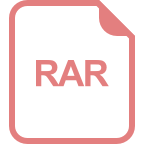
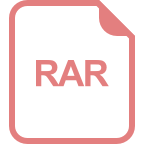













