np。linalg.lstsq
时间: 2024-06-18 18:03:51 浏览: 219
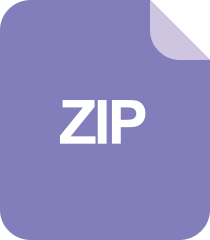
最小二乘法的 Python 程序.zip
np.linalg.lstsq是numpy中的一个函数,用于求解线性最小二乘问题,即给定一个线性方程组Ax = b,求解x的值。其中A为m x n的矩阵,b为m维的向量。该函数返回一个元组,包含了以下四个元素:
1. x:求解得到的x值;
2. residuals:残差平方和;
3. rank:矩阵A的秩;
4. s:矩阵A的奇异值。
可以使用以下代码调用该函数:
```
import numpy as np
A = np.array([[1, 2], [3, 4], [5, 6]])
b = np.array([1, 2, 3])
x, residuals, rank, s = np.linalg.lstsq(A, b, rcond=None)
```
其中rcond参数用于设置奇异值的阈值,通常使用默认值即可。如果A矩阵不是满秩的,则无法求解出唯一的x值,此时可以使用numpy中的伪逆函数np.linalg.pinv来进行求解。
阅读全文
相关推荐
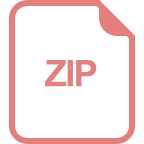
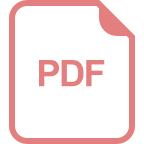















