self.P = tf.placeholder( shape=[1, self.shape_1[1], self.shape_1[2], 1], dtype=tf.float32, name="Pu"
时间: 2024-05-19 13:12:44 浏览: 123
)This line of code creates a TensorFlow placeholder named "P" with the specified shape and data type. The shape is a 4D tensor with a batch size of 1, a height of shape_1[1], a width of shape_1[2], and a channel of 1. The data type is set to float32. The placeholder is used to feed input data into the TensorFlow computational graph during runtime.
相关问题
def __init__(self, sess, state_dim, learning_rate): self.sess = sess self.s_dim = state_dim self.lr_rate = learning_rate # Create the critic network self.inputs, self.out = self.create_critic_network() # Get all network parameters self.network_params = \ tf.compat.v1.get_collection(tf.compat.v1.GraphKeys.TRAINABLE_VARIABLES, scope='critic') # Set all network parameters self.input_network_params = [] for param in self.network_params: self.input_network_params.append( tf.compat.v1.placeholder(tf.float32, shape=param.get_shape())) self.set_network_params_op = [] for idx, param in enumerate(self.input_network_params): self.set_network_params_op.append(self.network_params[idx].assign(param)) # Network target目标 V(s) self.td_target = tf.compat.v1.placeholder(tf.float32, [None, 1]) # Temporal Difference, will also be weights for actor_gradients时间差异,也将是actor_gradients的权重 self.td = tf.subtract(self.td_target, self.out) # Mean square error均方误差 self.loss = tflearn.mean_square(self.td_target, self.out) # Compute critic gradient计算临界梯度 self.critic_gradients = tf.gradients(self.loss, self.network_params) # Optimization Op self.optimize = tf.compat.v1.train.RMSPropOptimizer(self.lr_rate). \ apply_gradients(zip(self.critic_gradients, self.network_params))请对这段代码每句进行注释
# 定义一个类,表示 Critic 网络
class CriticNetwork(object):
def __init__(self, sess, state_dim, learning_rate):
# 初始化 Critic 网络的一些参数
self.sess = sess
self.s_dim = state_dim
self.lr_rate = learning_rate
# 创建 Critic 网络
self.inputs, self.out = self.create_critic_network()
# 获取 Critic 网络中所有的参数
self.network_params = tf.compat.v1.get_collection(tf.compat.v1.GraphKeys.TRAINABLE_VARIABLES, scope='critic')
# 定义一个占位符,表示 Critic 网络的输入参数
self.input_network_params = []
for param in self.network_params:
self.input_network_params.append(tf.compat.v1.placeholder(tf.float32, shape=param.get_shape()))
# 定义一个操作,用于设置 Critic 网络的所有参数
self.set_network_params_op = []
for idx, param in enumerate(self.input_network_params):
self.set_network_params_op.append(self.network_params[idx].assign(param))
# 定义一个占位符,表示 Critic 网络的目标输出
self.td_target = tf.compat.v1.placeholder(tf.float32, [None, 1])
# 计算 Critic 网络的 Temporal Difference
self.td = tf.subtract(self.td_target, self.out)
# 定义 Critic 网络的损失函数,使用均方误差
self.loss = tflearn.mean_square(self.td_target, self.out)
# 计算 Critic 网络的梯度
self.critic_gradients = tf.gradients(self.loss, self.network_params)
# 定义 Critic 网络的优化器
self.optimize = tf.compat.v1.train.RMSPropOptimizer(self.lr_rate).apply_gradients(zip(self.critic_gradients, self.network_params))
state = tf.placeholder( dtype=tf.float32, shape=[None, self.cell_size], name="initial_state" ) p_keep = tf.placeholder(dtype=tf.float32, name="p_keep") learning_rate = tf.placeholder(dtype=tf.float32, name="learning_rate") cell = tf.contrib.rnn.GRUCell(self.cell_size) drop_cell = tf.contrib.rnn.DropoutWrapper(cell, input_keep_prob=p_ke
可以推测出这是TensorFlow中的一个RNN模型,并且其中包含了一个GRU的单元。state、p_keep和learning_rate都是占位符,在模型的训练过程中用于传入实际的值。其中p_keep被用作Dropout的概率,而CELL_SIZE则表示GRU单元的状态向量大小。可以看出这是一个可训练的RNN模型。
阅读全文
相关推荐
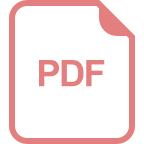
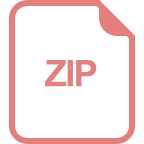
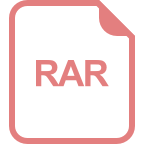










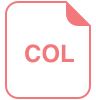


