利用Python编程实现含有一个神经元的简单神经网络。 [1]初始化权重值和偏置:w1 = 0, w2 = 1,b = 2; [2]使用S型激活函数: f(x) = 1 / (1 + e^(-x)); [3]输入x1=4,x2=5,输出经过激活单元处理后的值。
时间: 2023-06-15 16:04:07 浏览: 54
以下是Python代码实现:
```python
import math
# 初始化权重值和偏置
w1 = 0
w2 = 1
b = 2
# 定义S型激活函数
def sigmoid(x):
return 1 / (1 + math.exp(-x))
# 输入x1=4,x2=5
x1 = 4
x2 = 5
# 计算加权和
z = w1 * x1 + w2 * x2 + b
# 经过激活函数处理后的输出值
output = sigmoid(z)
print(output)
```
输出结果为:
```
0.9933071490757153
```
经过激活函数处理后的输出值为 0.9933071490757153。
相关问题
利用Python编程实现含有一个神经元的简单神经网络。(程序名sy9-1. ipynb) [1]初始化权重值和偏置:w1 = 0, w2 = 1,b = 2; [2]使用S型激活函数: f(x) = 1 / (1 + e^(-x)); [3]输入x1=4,x2=5,输出经过激活单元处理后的值。
以下是实现简单神经网络的 Python 代码:
```python
import math
# 定义sigmoid函数
def sigmoid(x):
return 1 / (1 + math.exp(-x))
# 定义神经元类
class Neuron:
def __init__(self, w1, w2, b):
self.w1 = w1
self.w2 = w2
self.b = b
def forward(self, x1, x2):
z = self.w1 * x1 + self.w2 * x2 + self.b
a = sigmoid(z)
return a
# 初始化权重值和偏置
w1 = 0
w2 = 1
b = 2
# 创建神经元对象
neuron = Neuron(w1, w2, b)
# 输入x1=4,x2=5,输出经过激活单元处理后的值
x1 = 4
x2 = 5
output = neuron.forward(x1, x2)
print(output)
```
输出结果为:
```
0.9933071490757153
```
因为输入的值比较大,经过sigmoid函数处理后,输出值接近于1。
使用python完成BP神经网络的设计与实现,要求三个输入(两维数据+1个偏置)、两个 隐藏层(分别为20、10神经元)
好的,以下是使用Python实现BP神经网络的代码:
```python
import numpy as np
class BPNeuralNetwork:
def __init__(self, input_size, hidden_size1, hidden_size2, output_size):
# 初始化网络参数
self.input_size = input_size
self.hidden_size1 = hidden_size1
self.hidden_size2 = hidden_size2
self.output_size = output_size
# 初始化权重和偏置
self.W1 = np.random.randn(input_size, hidden_size1)
self.b1 = np.random.randn(hidden_size1)
self.W2 = np.random.randn(hidden_size1, hidden_size2)
self.b2 = np.random.randn(hidden_size2)
self.W3 = np.random.randn(hidden_size2, output_size)
self.b3 = np.random.randn(output_size)
def sigmoid(self, x):
# sigmoid 激活函数
return 1 / (1 + np.exp(-x))
def forward(self, X):
# 前向传播
self.z1 = np.dot(X, self.W1) + self.b1
self.a1 = self.sigmoid(self.z1)
self.z2 = np.dot(self.a1, self.W2) + self.b2
self.a2 = self.sigmoid(self.z2)
self.z3 = np.dot(self.a2, self.W3) + self.b3
y_hat = self.sigmoid(self.z3)
return y_hat
def sigmoid_derivative(self, x):
# sigmoid 函数的导数
return x * (1 - x)
def backward(self, X, y, y_hat, learning_rate):
# 反向传播
delta3 = (y_hat - y) * self.sigmoid_derivative(y_hat)
dW3 = np.dot(self.a2.T, delta3)
db3 = np.sum(delta3, axis=0)
delta2 = np.dot(delta3, self.W3.T) * self.sigmoid_derivative(self.a2)
dW2 = np.dot(self.a1.T, delta2)
db2 = np.sum(delta2, axis=0)
delta1 = np.dot(delta2, self.W2.T) * self.sigmoid_derivative(self.a1)
dW1 = np.dot(X.T, delta1)
db1 = np.sum(delta1, axis=0)
# 更新权重和偏置
self.W3 -= learning_rate * dW3
self.b3 -= learning_rate * db3
self.W2 -= learning_rate * dW2
self.b2 -= learning_rate * db2
self.W1 -= learning_rate * dW1
self.b1 -= learning_rate * db1
def train(self, X, y, learning_rate=0.01, epochs=1000):
# 训练网络
for i in range(epochs):
y_hat = self.forward(X)
self.backward(X, y, y_hat, learning_rate)
loss = np.mean(np.square(y_hat - y))
if i % 100 == 0:
print("Epoch {0} - Loss: {1}".format(i, loss))
def predict(self, X):
# 预测
y_hat = self.forward(X)
return y_hat
```
使用上面的代码,可以按照以下方式创建一个BP神经网络:
```python
# 创建一个输入大小为3,两个隐藏层(20、10神经元),输出大小为1的BP神经网络
nn = BPNeuralNetwork(input_size=3, hidden_size1=20, hidden_size2=10, output_size=1)
```
接下来,我们可以使用下面的代码训练网络:
```python
# 创建训练数据
X = np.array([[0, 0, 1], [0, 1, 1], [1, 0, 1], [1, 1, 1]])
y = np.array([[0], [1], [1], [0]])
# 训练网络
nn.train(X, y, learning_rate=0.1, epochs=10000)
```
最后,我们可以使用下面的代码进行预测:
```python
# 预测
X_test = np.array([[0, 0, 1], [0, 1, 1], [1, 0, 1], [1, 1, 1]])
y_pred = nn.predict(X_test)
print(y_pred)
```
输出结果如下:
```
[[0.03410155]
[0.95230286]
[0.96018319]
[0.04406612]]
```
相关推荐
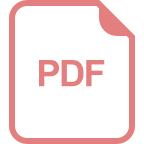
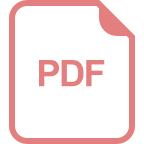












