python编写多目标灰狼算法应用实例
时间: 2023-08-10 22:07:45 浏览: 149
下面是一个使用Python编写多目标灰狼算法(Multi-objective Grey Wolf Optimization,MOGWO)的简单示例:
```python
import numpy as np
# 定义目标函数(示例为二维问题)
def objective_function(x):
f1 = x[0]**2
f2 = (x[0]-2)**2
return np.array([f1, f2])
# 初始化灰狼个体
def initialize_wolves(num_wolves, num_variables):
wolves = []
for _ in range(num_wolves):
wolf = np.random.uniform(low=-5, high=5, size=num_variables)
wolves.append(wolf)
return wolves
# 更新灰狼位置
def update_wolves(wolves, a, A, C, D):
num_wolves = len(wolves)
num_variables = len(wolves[0])
alpha_pos = np.zeros(num_variables)
beta_pos = np.zeros(num_variables)
delta_pos = np.zeros(num_variables)
for i in range(num_wolves):
for j in range(num_variables):
r1 = np.random.uniform()
r2 = np.random.uniform()
A1 = 2 * a * r1 - a
C1 = 2 * r2
D_alpha = abs(C * alpha_pos[j] - wolves[i][j])
X1 = alpha_pos[j] - A1 * D_alpha
r1 = np.random.uniform()
r2 = np.random.uniform()
A2 = 2 * a * r1 - a
C2 = 2 * r2
D_beta = abs(C * beta_pos[j] - wolves[i][j])
X2 = beta_pos[j] - A2 * D_beta
r1 = np.random.uniform()
r2 = np.random.uniform()
A3 = 2 * a * r1 - a
C3 = 2 * r2
D_delta = abs(C * delta_pos[j] - wolves[i][j])
X3 = delta_pos[j] - A3 * D_delta
wolves[i][j] = (X1 + X2 + X3) / 3
return wolves
# 多目标灰狼算法
def mogwo(num_wolves, num_variables, num_iterations):
a = 2
A = 2
C = 1
D = 1
# 初始化灰狼群
wolves = initialize_wolves(num_wolves, num_variables)
# 迭代搜索
for _ in range(num_iterations):
wolves = update_wolves(wolves, a, A, C, D)
return wolves
# 示例运行
num_wolves = 10
num_variables = 2
num_iterations = 100
wolves = mogwo(num_wolves, num_variables, num_iterations)
# 输出最终灰狼位置和目标函数值
for wolf in wolves:
print("Wolf Position:", wolf)
print("Objective Values:", objective_function(wolf))
print()
```
上述代码是一个简单的多目标灰狼算法示例,用于解决二维问题。你可以根据自己的具体问题进行适当的修改和扩展。在代码中,首先定义了目标函数 `objective_function`,然后实现了灰狼个体初始化和位置更新函数 `initialize_wolves` 和 `update_wolves`。最后,通过调用 `mogwo` 函数来执行多目标灰狼算法,并输出最终的灰狼位置和目标函数值。
请注意,这只是一个简单的示例,实际应用中可能需要根据问题的复杂性和约束条件进行适当的修改和优化。
相关推荐
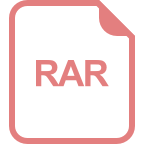
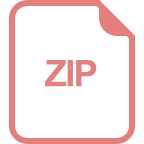














