Final Updates %--------------------------------- CostNew = Cost(coord); step = Step(coord); % Sigma update if CostNew < CostOld SigmaNew = SigmaNew + step * desc; end Sigma = SigmaNew;
时间: 2023-08-15 10:12:12 浏览: 38
这段代码看起来是一个优化算法的更新步骤。其中,CostNew是根据当前坐标(coord)计算得到的新的代价函数值,CostOld是之前的代价函数值,step是当前步长,desc是代价函数的梯度方向。如果CostNew比CostOld小,就将SigmaNew按照一定的步长(step * desc)进行更新。最后将Sigma赋值为SigmaNew。根据这个描述,可能是一个梯度下降法的实现。
相关问题
k-means均值聚类算法matlab代码
Sure, here is an example of the k-means clustering algorithm implemented in MATLAB:
```matlab
function [centroids, assignments] = kMeansClustering(data, k)
% Initialize centroids randomly
nSamples = size(data, 1);
centroidIndices = randperm(nSamples, k);
centroids = data(centroidIndices, :);
% Initialize assignments
assignments = zeros(nSamples, 1);
% Main loop
isConverged = false;
while ~isConverged
% Assign data points to nearest centroid
oldAssignments = assignments;
distances = pdist2(data, centroids);
[~, assignments] = min(distances, [], 2);
% Update centroids
for i = 1:k
clusterData = data(assignments == i, :);
if ~isempty(clusterData)
centroids(i, :) = mean(clusterData);
end
end
% Check convergence
isConverged = isequal(oldAssignments, assignments);
end
end
```
In this code, the `kMeansClustering` function takes two input arguments: `data` (the dataset to be clustered) and `k` (the number of clusters). It returns the final centroids (`centroids`) and the assignments of data points to clusters (`assignments`).
The algorithm starts by randomly initializing the centroids. Then, it iteratively assigns each data point to the nearest centroid and updates the centroids based on the assigned data points' mean. The iterations continue until the assignments no longer change.
You can use this function by passing your dataset (`data`) and the desired number of clusters (`k`). The function will return the final centroids and cluster assignments.
Please note that this implementation assumes you have already loaded your data into a matrix called `data`, where each row represents a data point and each column represents a feature. You may need to modify the code if your data is represented differently or if you have additional requirements.
can you use c++ write a k-means
Yes, it is possible to use the C programming language to implement the k-means algorithm. Here is an example implementation:
```
#include <stdio.h>
#include <stdlib.h>
#include <math.h>
#define K 3 // number of clusters
#define N 10 // number of data points
#define D 2 // number of dimensions
typedef struct {
double x[D];
} Point;
double distance(Point p1, Point p2) {
double sum = 0;
for (int i = 0; i < D; i++) {
sum += pow(p1.x[i] - p2.x[i], 2);
}
return sqrt(sum);
}
int main() {
Point data[N] = { {1, 2}, {2, 1}, {2, 3}, {3, 2}, {5, 6}, {6, 5}, {6, 7}, {7, 6}, {9, 8}, {8, 9} };
Point centroids[K] = { {1, 2}, {5, 6}, {9, 8} };
int assignment[N];
// repeat until convergence
int iter = 0;
while (1) {
// assignment step
int changed = 0;
for (int i = 0; i < N; i++) {
double min_dist = INFINITY;
int min_idx;
for (int j = 0; j < K; j++) {
double dist = distance(data[i], centroids[j]);
if (dist < min_dist) {
min_dist = dist;
min_idx = j;
}
}
if (assignment[i] != min_idx) {
assignment[i] = min_idx;
changed = 1;
}
}
if (!changed) {
break;
}
// update step
int counts[K] = { 0 };
for (int i = 0; i < N; i++) {
int idx = assignment[i];
for (int j = 0; j < D; j++) {
centroids[idx].x[j] += data[i].x[j];
}
counts[idx]++;
}
for (int j = 0; j < K; j++) {
if (counts[j] > 0) {
for (int l = 0; l < D; l++) {
centroids[j].x[l] /= counts[j];
}
}
}
iter++;
}
// print results
printf("Iterations: %d\n", iter);
for (int i = 0; i < K; i++) {
printf("Centroid %d: (%.2f, %.2f)\n", i+1, centroids[i].x[0], centroids[i].x[1]);
}
for (int i = 0; i < N; i++) {
printf("Data point %d assigned to centroid %d\n", i+1, assignment[i]+1);
}
return 0;
}
```
This implementation uses a fixed dataset of 10 two-dimensional points and 3 clusters. The algorithm iteratively assigns each data point to the nearest centroid and updates the centroids based on the assigned points. It continues until convergence, i.e., when no data points change assignments. The final centroids and assignments are printed to the console. Note that this implementation does not handle error cases or input validation, and can be improved for better performance.
相关推荐
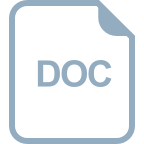
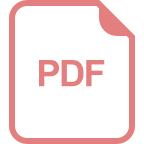
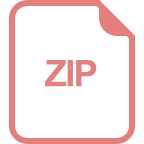












