nn.BCEWithLogitsLoss()对代码解释
时间: 2023-11-13 16:14:06 浏览: 30
`nn.BCEWithLogitsLoss()` 是 PyTorch 中用于计算二分类问题的损失函数。其中 BCE 代表 Binary Cross Entropy,即二元交叉熵损失函数。而 `WithLogits` 则表示输入的张量没有经过 Sigmoid 函数转换。这个函数的输入包括两个张量,一个是模型输出的预测得分,另一个是真实标签的二元编码,即正例为 1,负例为 0。在计算损失时,该函数首先会对模型输出的预测得分进行 Sigmoid 函数转换,将其转换为 0 到 1 之间的概率值,然后再计算损失。这样做的好处是可以避免数值不稳定的情况出现,同时也可以提高模型的收敛速度。
相关问题
还有个问题,可否帮助我解释这个问题:RuntimeError: torch.nn.functional.binary_cross_entropy and torch.nn.BCELoss are unsafe to autocast. Many models use a sigmoid layer right before the binary cross entropy layer. In this case, combine the two layers using torch.nn.functional.binary_cross_entropy_with_logits or torch.nn.BCEWithLogitsLoss. binary_cross_entropy_with_logits and BCEWithLogits are safe to autocast.
这个错误是在告诉你,使用`torch.nn.functional.binary_cross_entropy`或`torch.nn.BCELoss`计算二元交叉熵损失是不安全的。它建议你使用`torch.nn.functional.binary_cross_entropy_with_logits`或`torch.nn.BCEWithLogitsLoss`来代替。
在使用二元交叉熵损失的时候,通常需要在计算交叉熵损失之前使用sigmoid函数将输出转化为概率值。`binary_cross_entropy_with_logits`和`BCEWithLogitsLoss`已经内置了sigmoid函数,所以你可以直接使用它们而不用担心sigmoid函数带来的问题。
举个例子,你可以将如下代码:
```
import torch.nn as nn
# Compute the loss using the sigmoid of the output and the binary cross entropy loss
output = model(input)
loss = nn.functional.binary_cross_entropy(nn.functional.sigmoid(output), target)
```
改为如下代码:
```
import torch.nn as nn
# Compute the loss using the binary cross entropy loss with logits
output = model(input)
loss = nn.BCEWithLogitsLoss(output, target)
```
class BCEBlurWithLogitsLoss(nn.Module): # BCEwithLogitLoss() with reduced missing label effects. def __init__(self, alpha=0.05): super().__init__() self.loss_fcn = nn.BCEWithLogitsLoss(reduction='none') # must be nn.BCEWithLogitsLoss() self.alpha = alpha def forward(self, pred, true): loss = self.loss_fcn(pred, true) pred = torch.sigmoid(pred) # prob from logits dx = pred - true # reduce only missing label effects # dx = (pred - true).abs() # reduce missing label and false label effects alpha_factor = 1 - torch.exp((dx - 1) / (self.alpha + 1e-4)) loss *= alpha_factor return loss.mean()这个代码什么意思
这个代码实现了一个自定义的损失函数 `BCEBlurWithLogitsLoss`,它是基于二元交叉熵损失函数 `nn.BCEWithLogitsLoss` 的改进。
在二元交叉熵损失函数中,如果某些标签是缺失的,则该标签对应的损失将被忽略。但是在某些情况下,即使标签存在,模型的预测也可能是错误的,这会导致一些 false label effects。因此,在这个自定义的损失函数中,通过引入一个参数 alpha,同时减少了 missing label effects 和 false label effects。
具体来说,该函数的输入参数为模型的预测 `pred` 和真实标签 `true`,首先通过调用 `nn.BCEWithLogitsLoss` 计算二元交叉熵损失。接着,将模型的预测值 `pred` 通过 `torch.sigmoid()` 转换为概率值,然后计算预测值和真实值之间的差值 `dx`。进一步,将 `dx` 映射到一个 alpha_factor 权重,用于减少 missing label effects 和 false label effects,并将其应用于计算的损失值中,最终返回平均损失值。
相关推荐
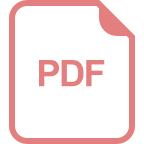
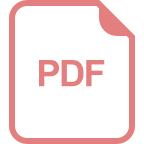
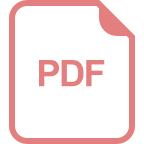












