H = homography2d(original_points, target_points);函数定义函数体MATLAB
时间: 2023-06-12 09:07:31 浏览: 69
function H = homography2d(original_points, target_points)
% HOMOGRAPHY2D Computes the homography between two sets of 2D points
% H = HOMOGRAPHY2D(ORIGINAL_POINTS, TARGET_POINTS) computes the
% homography between two sets of 2D points using the Direct Linear
% Transform (DLT) algorithm.
%
% INPUTS:
% original_points - 2xN matrix of N original 2D points
% target_points - 2xN matrix of N target 2D points
%
% OUTPUTS:
% H - 3x3 homography matrix that maps original points to target points
% Check inputs
assert(size(original_points, 1) == 2, 'original_points should be a 2xN matrix');
assert(size(target_points, 1) == 2, 'target_points should be a 2xN matrix');
assert(size(original_points, 2) == size(target_points, 2), 'original_points and target_points should have the same number of points');
% Normalize points
[original_points_normalized, T_original] = normalize_points(original_points);
[target_points_normalized, T_target] = normalize_points(target_points);
% Construct matrix A
N = size(original_points_normalized, 2);
A = zeros(2*N, 9);
for i=1:N
A(2*i-1, :) = [0, 0, 0, -original_points_normalized(1,i), -original_points_normalized(2,i), -1, target_points_normalized(2,i)*original_points_normalized(1,i), target_points_normalized(2,i)*original_points_normalized(2,i), target_points_normalized(2,i)];
A(2*i, :) = [original_points_normalized(1,i), original_points_normalized(2,i), 1, 0, 0, 0, -target_points_normalized(1,i)*original_points_normalized(1,i), -target_points_normalized(1,i)*original_points_normalized(2,i), -target_points_normalized(1,i)];
end
% Solve Ah = 0 using SVD
[~, ~, V] = svd(A);
h = V(:, end);
% Reshape h to obtain H
H = reshape(h, 3, 3)';
% Denormalize H
H = T_target \ H * T_original;
end
function [points_normalized, T] = normalize_points(points)
% NORMALIZE_POINTS Normalizes 2D points to have zero mean and unit standard deviation
% [POINTS_NORMALIZED, T] = NORMALIZE_POINTS(POINTS) normalizes 2D points
% to have zero mean and unit standard deviation by scaling and translating
% the points according to the formula: x' = (x - mu) / sigma, where x is
% the original point, x' is the normalized point, mu is the mean of all
% points and sigma is the standard deviation of all points. The function
% returns the normalized points and the transformation matrix T that has
% to be applied to the points to obtain their normalized version.
% Compute mean and standard deviation of points
mu = mean(points, 2);
sigma = std(points, 0, 2);
% Compute transformation matrix
T = [1/sigma(1) 0 -mu(1)/sigma(1); 0 1/sigma(2) -mu(2)/sigma(2); 0 0 1];
% Apply transformation matrix to points
points_normalized = T * [points; ones(1, size(points, 2))];
% Discard last row of ones
points_normalized = points_normalized(1:2, :);
end
相关推荐
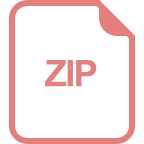
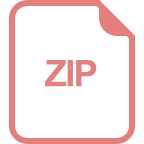














